Evaluating Effects of Resting-State Electroencephalography Data Pre-Processing on a Machine Learning Task for Parkinson’s Disease
medRxiv (Cold Spring Harbor Laboratory)(2023)
摘要
Resting-state electroencephalography (RSEEG) is a method under consideration as a potential biomarker that could support early and accurate diagnosis of Parkinson’s disease (PD). RSEEG data is often contaminated by signals arising from other electrophysiological sources and the environment, necessitating pre-processing of the data prior to applying machine learning methods for classification. Importantly, using differing degrees of pre-processing will lead to different classification results. This study aimed to examine this by evaluating the difference in experimental results when using re-referenced data, data that had undergone filtering and artefact rejection, and data without muscle artefact. The results demonstrated that, using a Random Forest Classifier for feature selection and a Support Vector Machine for disease classification, different levels of pre-processing led to markedly different classification results. In particular, the presence of muscle artefact was associated with inflated classification accuracy, emphasising the importance of its removal as part of pre-processing.
### Competing Interest Statement
The authors have declared no competing interest.
### Funding Statement
This research was funded by and has been delivered in partnership with Our Health in Our Hands (OHIOH), a strategic initiative of the Australian National University, which aims to transform health care by developing new personalized health technologies and solutions in collaboration with patients, clinicians, and health-care providers. We gratefully acknowledge the funding from the ANU School of Computing for the PhD studies of the first author.
### Author Declarations
I confirm all relevant ethical guidelines have been followed, and any necessary IRB and/or ethics committee approvals have been obtained.
Yes
The details of the IRB/oversight body that provided approval or exemption for the research described are given below:
The ACT Health Human Research Ethics Committee (protocol ETH.4.16.060) and the Australian National University (ANU) Human Research Ethics Committee (protocol No. 2020/612) gave ethical approval for this work.
I confirm that all necessary patient/participant consent has been obtained and the appropriate institutional forms have been archived, and that any patient/participant/sample identifiers included were not known to anyone (e.g., hospital staff, patients or participants themselves) outside the research group so cannot be used to identify individuals.
Yes
I understand that all clinical trials and any other prospective interventional studies must be registered with an ICMJE-approved registry, such as ClinicalTrials.gov. I confirm that any such study reported in the manuscript has been registered and the trial registration ID is provided (note: if posting a prospective study registered retrospectively, please provide a statement in the trial ID field explaining why the study was not registered in advance).
Yes
I have followed all appropriate research reporting guidelines, such as any relevant EQUATOR Network research reporting checklist(s) and other pertinent material, if applicable.
Yes
The data collected at The Canberra Hospital in the present study are available upon reasonable request to the authors. The data collected at the University of Turku are available online at The data collected at the University of New Mexico are available at
更多查看译文
关键词
parkinsons,machine learning task,machine learning,resting-state,pre-processing
AI 理解论文
溯源树
样例
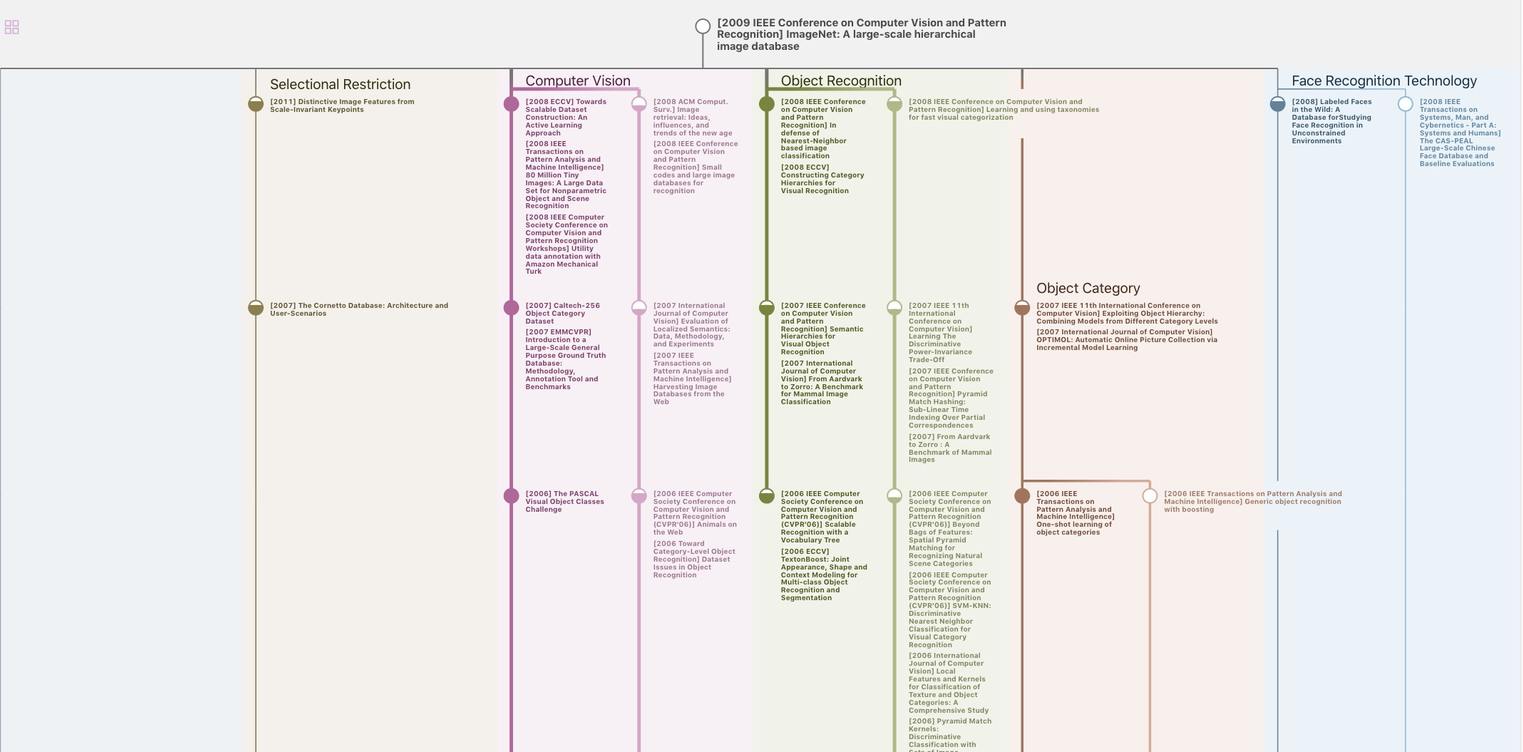
生成溯源树,研究论文发展脉络
Chat Paper
正在生成论文摘要