Deep Learning using Susceptibility-Weighted MR Sequence to Detect Microbleeds and Classify Cerebral Small Vessel Disease
medrxiv(2023)
摘要
Background Microbleeds (CMBs) serve as neuroimaging biomarkers to assess risk of intracerebral hemorrhage and diagnose cerebral small vessel disease (CSVD). Therefore, detecting CMBs can evaluate the risk of intracerebral hemorrhage and use its presence to support CSVD classification, both are conducive to optimizing CSVD management. This study aimed to develop and test a deep learning (DL) model based on susceptibility-weighted MR sequence (SWS) to detect CMBs and classify CSVD to assist neurologists in optimizing CSVD management.
Methods Patients with arteriolosclerosis (aSVD), cerebral amyloid angiopathy (CAA), and cerebral autosomal dominant arteriopathy with subcortical infarcts and leukoencephalopathy (CADASIL) treated at three centers were enrolled between January 2017 and May 2022 in this retrospective study. The SWSs of patients from two centers were used as the development set, and the SWSs of patients from the remaining center were used as the external test set. The DL model contains a Mask R-CNN for detecting CMBs and a multi-instance learning (MIL) network for classifying CSVD. The metrics for model performance included intersection over union (IoU), Dice score, recall, confusion matrices, receiver operating characteristic curve (ROC) analysis, accuracy, precision, and F1-score.
Results A total of 364 SWS were recruited, including 336 in the development set and 28 in the external test set. IoU for the model was 0.523 ± 0.319, Dice score 0.627 ± 0.296, and recall 0.706 ± 0.365 for CMBs detection in the external test set. For CSVD classification, the model achieved a weighted-average AUC of 0.908 (95% CI: 0.895-0.921), accuracy of 0.819 (95% CI: 0.768-0.870), weighted-average precision of 0.864 (95% CI: 0.831-0.897), and weighted-average F1-score of 0.829 (95% CI: 0.782-0.876) in the external set, outperforming the performance of the neurologist group.
Conclusion The DL model based on SWS can detect CMBs and classify CSVD, thereby assisting neurologists in optimizing CSVD management.
### Competing Interest Statement
The authors have declared no competing interest.
### Clinical Trial
This study was conducted following the Declaration of Helsinki. Requirements for informed consent were waived because of the study's retrospective nature.
### Funding Statement
This work was supported by the National Natural Science Foundation of China (Grant No. 81971110 and 82171307), the Key Areas R&D Program of Science and Technology Program of Guangzhou (Grant No. 202206060001), the Science and Technology Program of Guangzhou (Grant No. 202007030010), and the High-level Hospital Construction Research Project of Maoming People's Hospital, the Science and Technology Special Fund Program of Maoming (Grant No. 2020KJZX006).
### Author Declarations
I confirm all relevant ethical guidelines have been followed, and any necessary IRB and/or ethics committee approvals have been obtained.
Yes
The details of the IRB/oversight body that provided approval or exemption for the research described are given below:
The Medical Ethics Committee of the Third Affiliated Hospital of Sun Yat-sen University
I confirm that all necessary patient/participant consent has been obtained and the appropriate institutional forms have been archived, and that any patient/participant/sample identifiers included were not known to anyone (e.g., hospital staff, patients or participants themselves) outside the research group so cannot be used to identify individuals.
Yes
I understand that all clinical trials and any other prospective interventional studies must be registered with an ICMJE-approved registry, such as ClinicalTrials.gov. I confirm that any such study reported in the manuscript has been registered and the trial registration ID is provided (note: if posting a prospective study registered retrospectively, please provide a statement in the trial ID field explaining why the study was not registered in advance).
Yes
I have followed all appropriate research reporting guidelines, such as any relevant EQUATOR Network research reporting checklist(s) and other pertinent material, if applicable.
Yes
The Medical Ethics Committee of the Third Affiliated Hospital of Sun Yat-sen University approved this retrospective multicenter study.
* CMBs
: microbleeds
CSVD
: cerebral small vessel disease
DL
: deep learning
SWS
: susceptibility-weighted MR sequence
aSVD
: arteriolosclerosis
CAA
: cerebral amyloid angiopathy
CADASIL
: cerebral autosomal dominant arteriopathy with subcortical infarcts and leukoencephalopathy
MIL
: multi-instance learning
IoU
: intersection over union
ROC
: receiver operating characteristic curve
AUC
: area under receiver operating characteristic curve
SWAN
: T2-star-weighted angiography
SWI
: susceptibility-weighted imaging
STRIVE
: STandards for ReportIng Vascular changes on nEurouimaging
SYSUTH
: Third Affiliated Hospital, Sun Yat-sen University (SYSUTH)
MMPH
: Maoming People’s Hospital
STUMFH
: First Affiliated Hospital of SHANTOU University Medical College
SD
: standard deviation
Grad-CAM
: Gradient-weighted Class Activation Mapping
CI
: confidence interval
3D-CNN
: three-dimensional convolutional neural network
2D-CNN
: two-dimensional convolutional neural network
更多查看译文
关键词
vessel,susceptibility-weighted
AI 理解论文
溯源树
样例
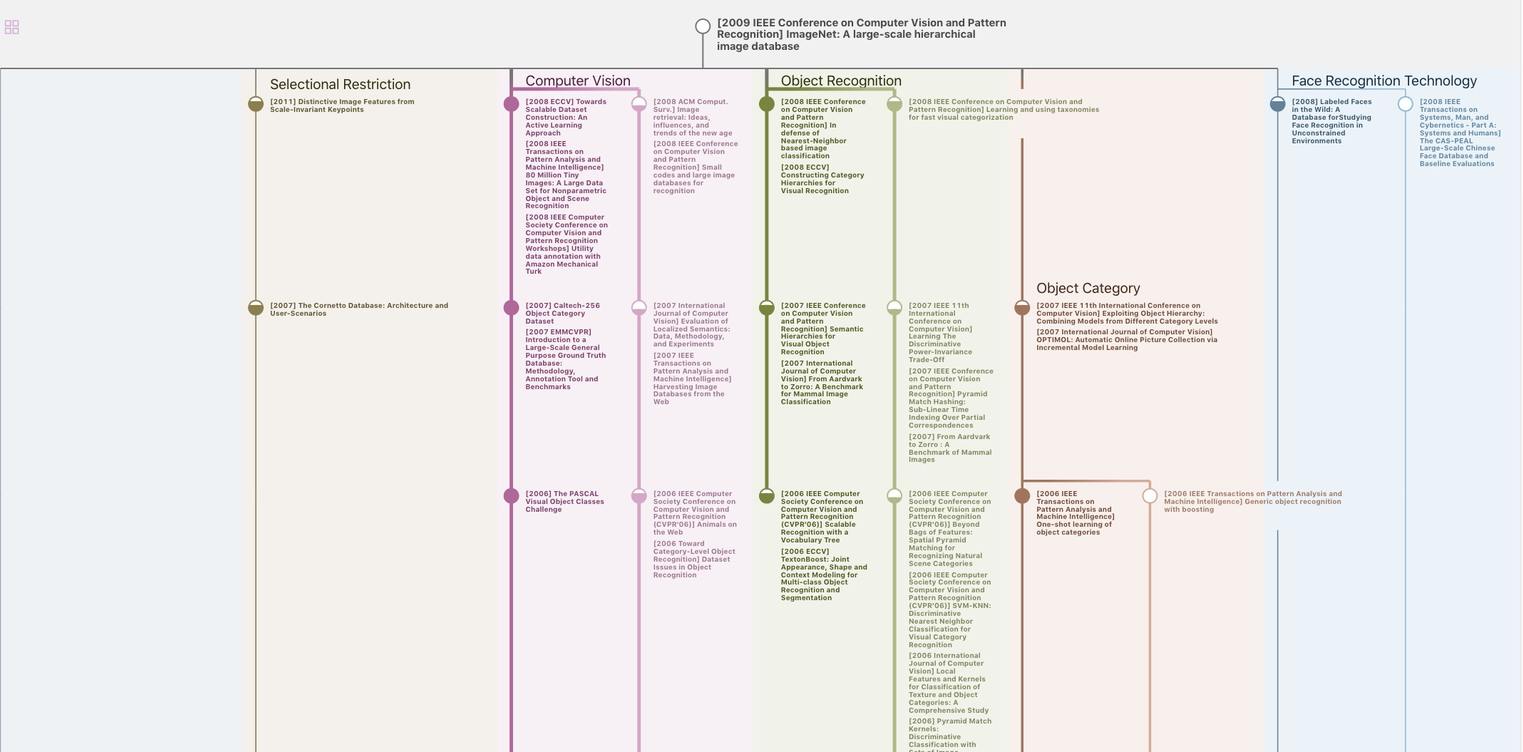
生成溯源树,研究论文发展脉络
Chat Paper
正在生成论文摘要