Fast, light, and scalable: harnessing data-mined line annotations for automated tumor segmentation on brain MRI
European Radiology(2023)
摘要
Objectives While fully supervised learning can yield high-performing segmentation models, the effort required to manually segment large training sets limits practical utility. We investigate whether data mined line annotations can facilitate brain MRI tumor segmentation model development without requiring manually segmented training data. Methods In this retrospective study, a tumor detection model trained using clinical line annotations mined from PACS was leveraged with unsupervised segmentation to generate pseudo-masks of enhancing tumors on T1-weighted post-contrast images (9911 image slices; 3449 adult patients). Baseline segmentation models were trained and employed within a semi-supervised learning (SSL) framework to refine the pseudo-masks. Following each self-refinement cycle, a new model was trained and tested on a held-out set of 319 manually segmented image slices (93 adult patients), with the SSL cycles continuing until Dice score coefficient (DSC) peaked. DSCs were compared using bootstrap resampling. Utilizing the best-performing models, two inference methods were compared: (1) conventional full-image segmentation, and (2) a hybrid method augmenting full-image segmentation with detection plus image patch segmentation. Results Baseline segmentation models achieved DSC of 0.768 (U-Net), 0.831 (Mask R-CNN), and 0.838 (HRNet), improving with self-refinement to 0.798, 0.871, and 0.873 (each p < 0.001), respectively. Hybrid inference outperformed full image segmentation alone: DSC 0.884 (Mask R-CNN) vs. 0.873 (HRNet), p < 0.001. Conclusions Line annotations mined from PACS can be harnessed within an automated pipeline to produce accurate brain MRI tumor segmentation models without manually segmented training data, providing a mechanism to rapidly establish tumor segmentation capabilities across radiology modalities. Key Points • A brain MRI tumor detection model trained using clinical line measurement annotations mined from PACS was leveraged to automatically generate tumor segmentation pseudo-masks . • An iterative self-refinement process automatically improved pseudo-mask quality, with the best-performing segmentation pipeline achieving a Dice score of 0.884 on a held-out test set . • Tumor line measurement annotations generated in routine clinical radiology practice can be harnessed to develop high-performing segmentation models without manually segmented training data, providing a mechanism to rapidly establish tumor segmentation capabilities across radiology modalities .
更多查看译文
关键词
Deep learning,Magnetic resonance imaging,Brain,Radiology,Neoplasms
AI 理解论文
溯源树
样例
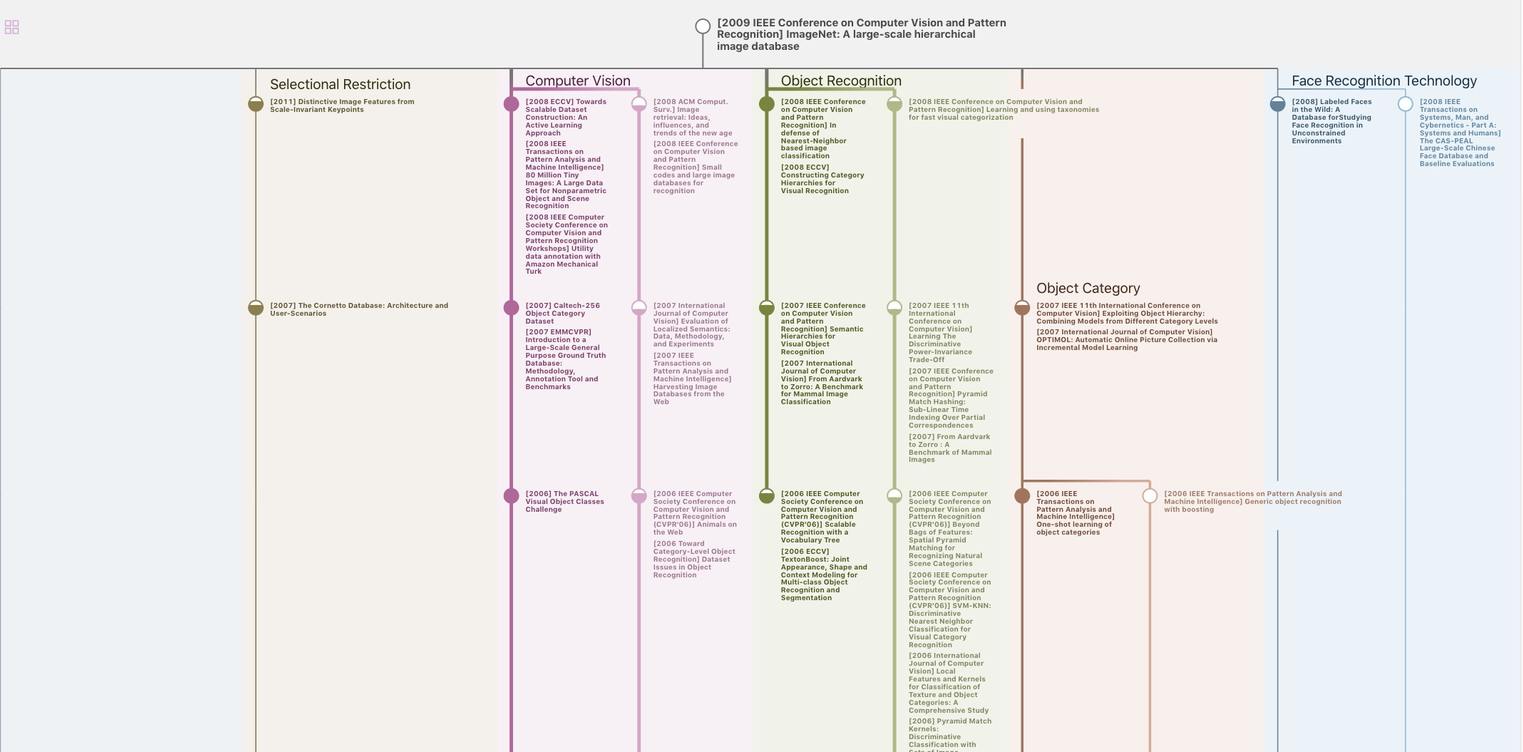
生成溯源树,研究论文发展脉络
Chat Paper
正在生成论文摘要