Piloting an automated clinical trial eligibility surveillance and provider alert system based on artificial intelligence and standard data models
BMC Medical Research Methodology(2023)
摘要
Background To advance new therapies into clinical care, clinical trials must recruit enough participants. Yet, many trials fail to do so, leading to delays, early trial termination, and wasted resources. Under-enrolling trials make it impossible to draw conclusions about the efficacy of new therapies. An oft-cited reason for insufficient enrollment is lack of study team and provider awareness about patient eligibility. Automating clinical trial eligibility surveillance and study team and provider notification could offer a solution. Methods To address this need for an automated solution, we conducted an observational pilot study of our TAES (TriAl Eligibility Surveillance) system. We tested the hypothesis that an automated system based on natural language processing and machine learning algorithms could detect patients eligible for specific clinical trials by linking the information extracted from trial descriptions to the corresponding clinical information in the electronic health record (EHR). To evaluate the TAES information extraction and matching prototype (i.e., TAES prototype), we selected five open cardiovascular and cancer trials at the Medical University of South Carolina and created a new reference standard of 21,974 clinical text notes from a random selection of 400 patients (including at least 100 enrolled in the selected trials), with a small subset of 20 notes annotated in detail. We also developed a simple web interface for a new database that stores all trial eligibility criteria, corresponding clinical information, and trial-patient match characteristics using the Observational Medical Outcomes Partnership (OMOP) common data model. Finally, we investigated options for integrating an automated clinical trial eligibility system into the EHR and for notifying health care providers promptly of potential patient eligibility without interrupting their clinical workflow. Results Although the rapidly implemented TAES prototype achieved only moderate accuracy (recall up to 0.778; precision up to 1.000), it enabled us to assess options for integrating an automated system successfully into the clinical workflow at a healthcare system. Conclusions Once optimized, the TAES system could exponentially enhance identification of patients potentially eligible for clinical trials, while simultaneously decreasing the burden on research teams of manual EHR review. Through timely notifications, it could also raise physician awareness of patient eligibility for clinical trials.
更多查看译文
关键词
Medical informatics [L01.313.500],Natural language processing (NLP) [L01.224.050.375.580],Data science [L01.305],Clinical trial enrollment/recruitment
AI 理解论文
溯源树
样例
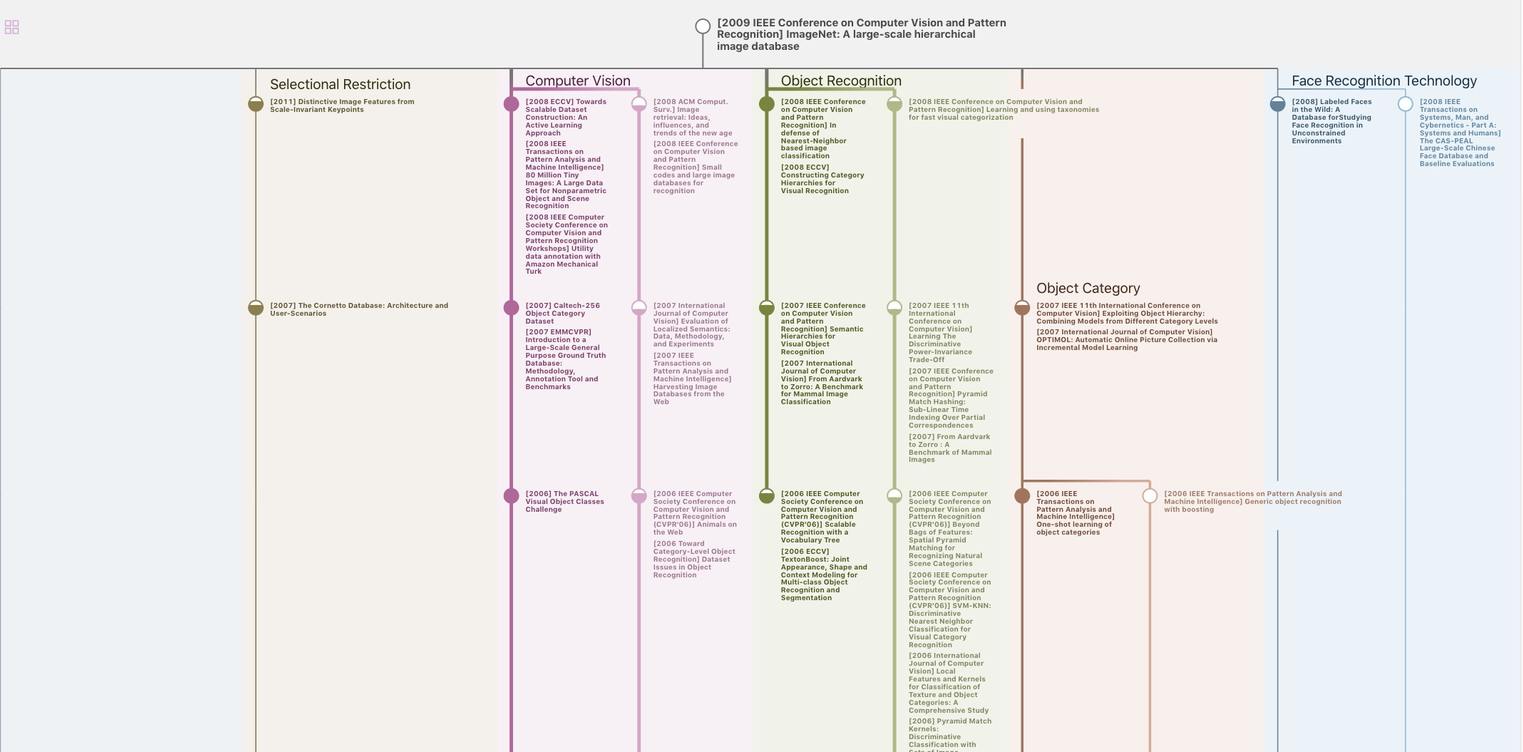
生成溯源树,研究论文发展脉络
Chat Paper
正在生成论文摘要