Boosted Prompt Ensembles for Large Language Models
CoRR(2023)
摘要
Methods such as chain-of-thought prompting and self-consistency have pushed the frontier of language model reasoning performance with no additional training. To further improve performance, we propose a prompt ensembling method for large language models, which uses a small dataset to construct a set of few shot prompts that together comprise a ``boosted prompt ensemble''. The few shot examples for each prompt are chosen in a stepwise fashion to be ``hard'' examples on which the previous step's ensemble is uncertain. We show that this outperforms single-prompt output-space ensembles and bagged prompt-space ensembles on the GSM8k and AQuA datasets, among others. We propose both train-time and test-time versions of boosted prompting that use different levels of available annotation and conduct a detailed empirical study of our algorithm.
更多查看译文
关键词
large language models,prompt ensembles
AI 理解论文
溯源树
样例
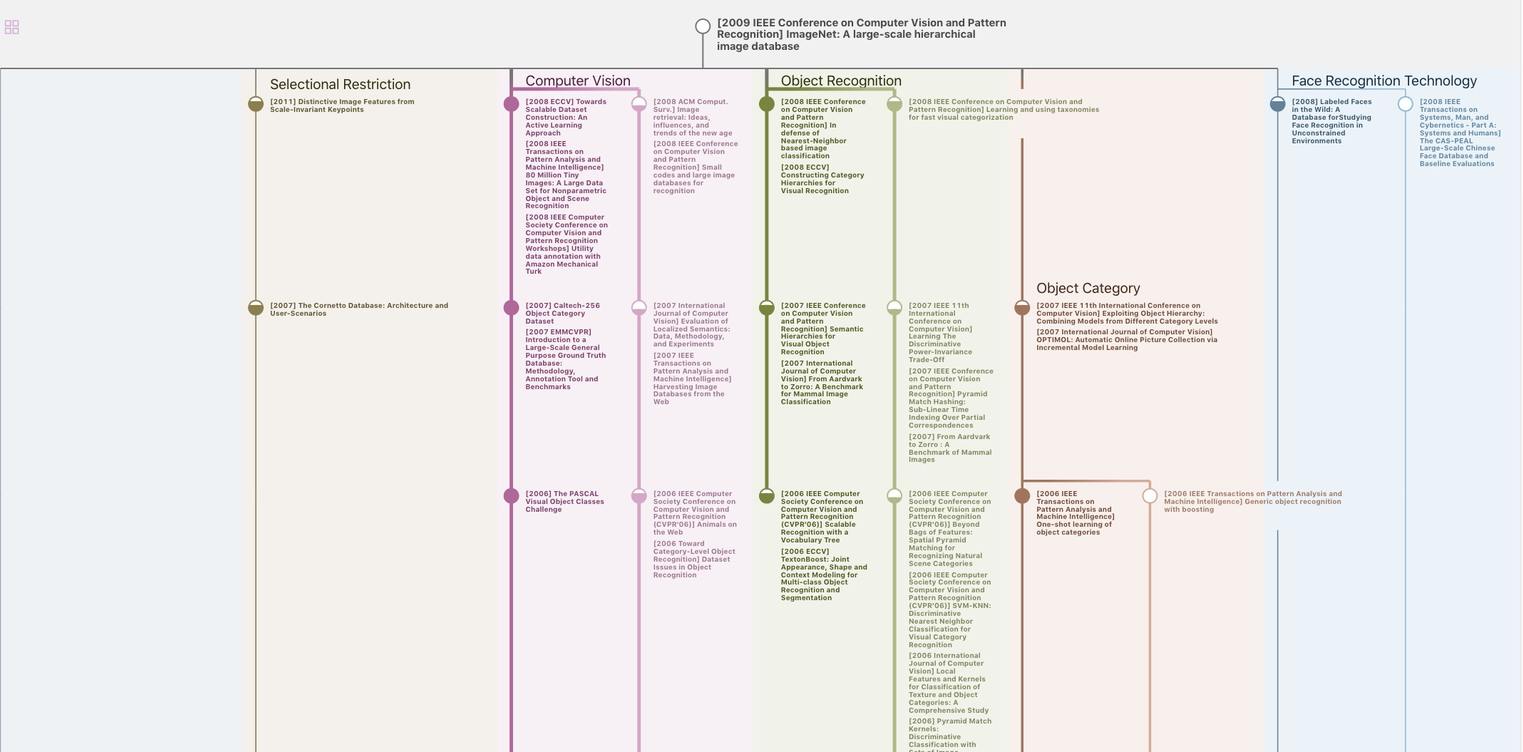
生成溯源树,研究论文发展脉络
Chat Paper
正在生成论文摘要