AutoRepair: Automated Repair for AI-Enabled Cyber-Physical Systems under Safety-Critical Conditions
CoRR(2023)
摘要
Cyber-Physical Systems (CPS) have been widely deployed in safety-critical domains such as transportation, power and energy. Recently, there comes an increasing demand in employing deep neural networks (DNNs) in CPS for more intelligent control and decision making in sophisticated industrial safety-critical conditions, giving birth to the class of DNN controllers. However, due to the inherent uncertainty and opaqueness of DNNs, concerns about the safety of DNN-enabled CPS are also surging. In this work, we propose an automated framework named AutoRepair that, given a safety requirement, identifies unsafe control behavior in a DNN controller and repairs them through an optimization-based method. Having an unsafe signal of system execution, AutoRepair iteratively explores the control decision space and searches for the optimal corrections for the DNN controller in order to satisfy the safety requirements. We conduct a comprehensive evaluation of AutoRepair on 6 instances of industry-level DNN-enabled CPS from different safety-critical domains. Evaluation results show that AutoRepair successfully repairs critical safety issues in the DNN controllers, and significantly improves the reliability of CPS.
更多查看译文
关键词
automated autorepair,ai-enabled,cyber-physical,safety-critical
AI 理解论文
溯源树
样例
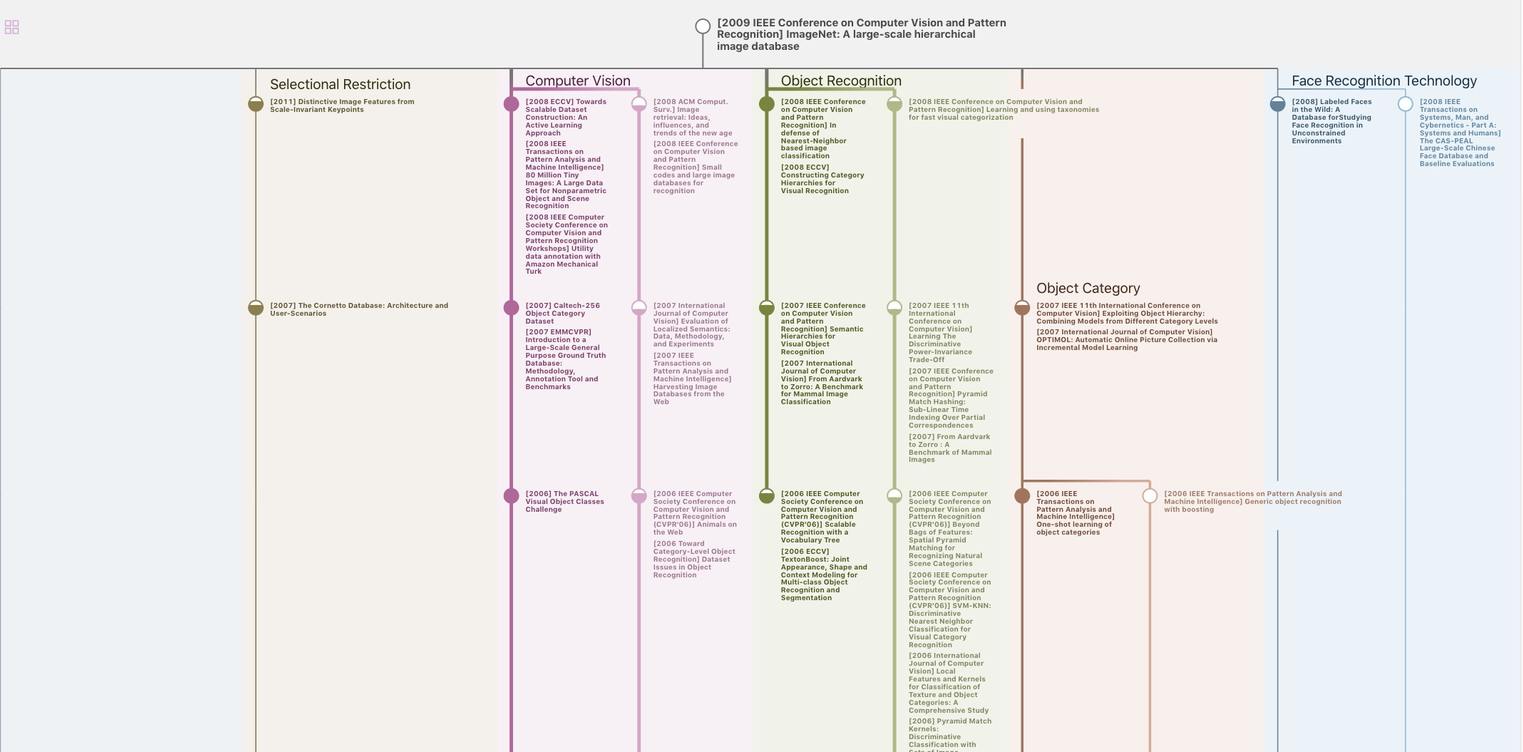
生成溯源树,研究论文发展脉络
Chat Paper
正在生成论文摘要