Computational Methods Summarizing Mutational Patterns in Cancer: Promise and Limitations for Clinical Applications
Cancers(2023)
摘要
Since the rise of next-generation sequencing technologies, the catalogue of mutations in cancer has been continuously expanding. To address the complexity of the cancer-genomic landscape and extract meaningful insights, numerous computational approaches have been developed over the last two decades. In this review, we survey the current leading computational methods to derive intricate mutational patterns in the context of clinical relevance. We begin with mutation signatures, explaining first how mutation signatures were developed and then examining the utility of studies using mutation signatures to correlate environmental effects on the cancer genome. Next, we examine current clinical research that employs mutation signatures and discuss the potential use cases and challenges of mutation signatures in clinical decision-making. We then examine computational studies developing tools to investigate complex patterns of mutations beyond the context of mutational signatures. We survey methods to identify cancer-driver genes, from single-driver studies to pathway and network analyses. In addition, we review methods inferring complex combinations of mutations for clinical tasks and using mutations integrated with multi-omics data to better predict cancer phenotypes. We examine the use of these tools for either discovery or prediction, including prediction of tumor origin, treatment outcomes, prognosis, and cancer typing. We further discuss the main limitations preventing widespread clinical integration of computational tools for the diagnosis and treatment of cancer. We end by proposing solutions to address these challenges using recent advances in machine learning.
更多查看译文
关键词
cancer genomics,mutation signatures,machine learning,bioinformatics,clinical predictors,cancer drivers
AI 理解论文
溯源树
样例
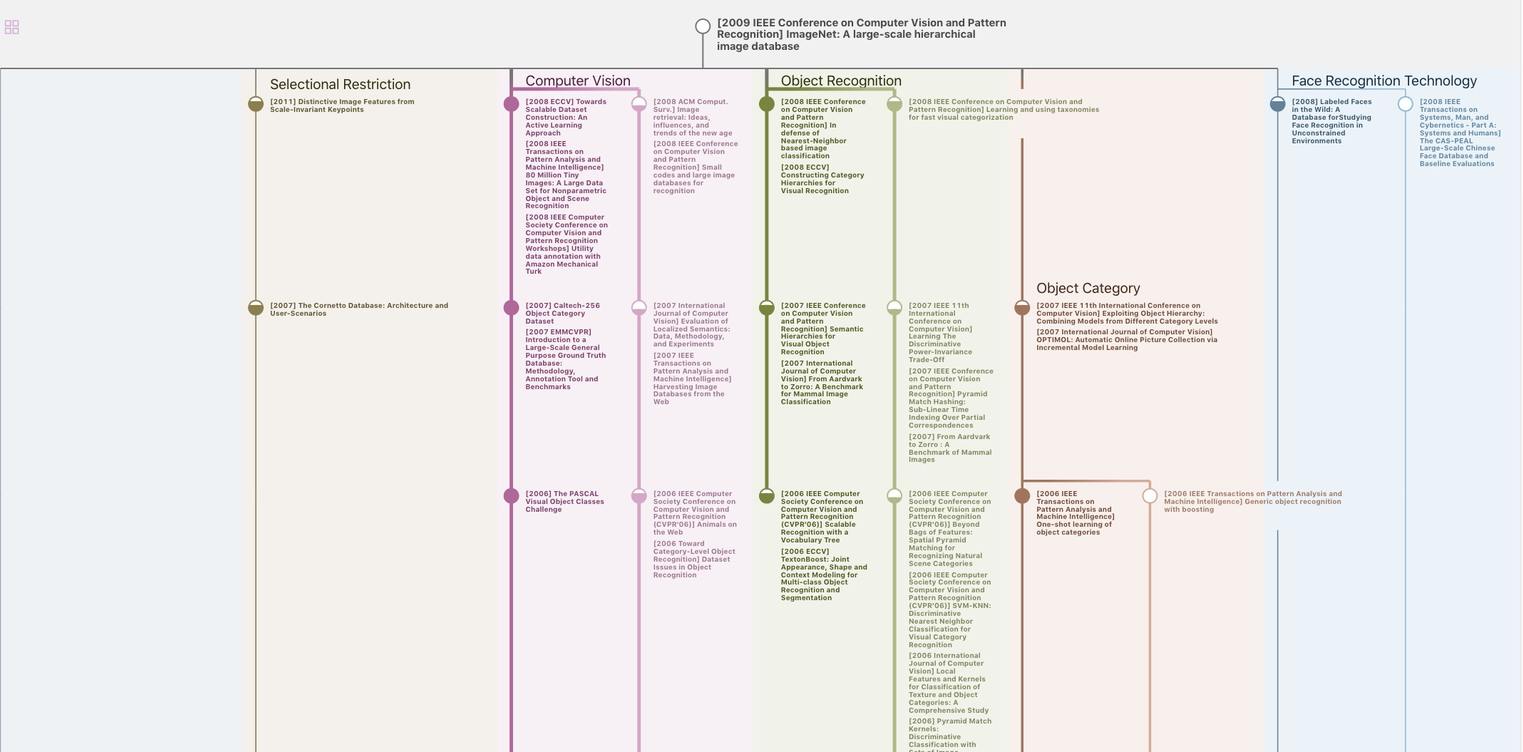
生成溯源树,研究论文发展脉络
Chat Paper
正在生成论文摘要