Machine Learning Analysis of Hyperspectral Images of Damaged Wheat Kernels
Sensors(2023)
摘要
Fusarium head blight (FHB) is a disease of small grains caused by the fungus Fusarium graminearum. In this study, we explored the use of hyperspectral imaging (HSI) to evaluate the damage caused by FHB in wheat kernels. We evaluated the use of HSI for disease classification and correlated the damage with the mycotoxin deoxynivalenol (DON) content. Computational analyses were carried out to determine which machine learning methods had the best accuracy to classify different levels of damage in wheat kernel samples. The classes of samples were based on the DON content obtained from Gas Chromatography–Mass Spectrometry (GC-MS). We found that G-Boost, an ensemble method, showed the best performance with 97% accuracy in classifying wheat kernels into different severity levels. Mask R-CNN, an instance segmentation method, was used to segment the wheat kernels from HSI data. The regions of interest (ROIs) obtained from Mask R-CNN achieved a high mAP of 0.97. The results from Mask R-CNN, when combined with the classification method, were able to correlate HSI data with the DON concentration in small grains with an R2 of 0.75. Our results show the potential of HSI to quantify DON in wheat kernels in commercial settings such as elevators or mills.
更多查看译文
关键词
deoxynivalenol (DON),fusarium head blight,hyperspectral imaging,machine learning,object detection
AI 理解论文
溯源树
样例
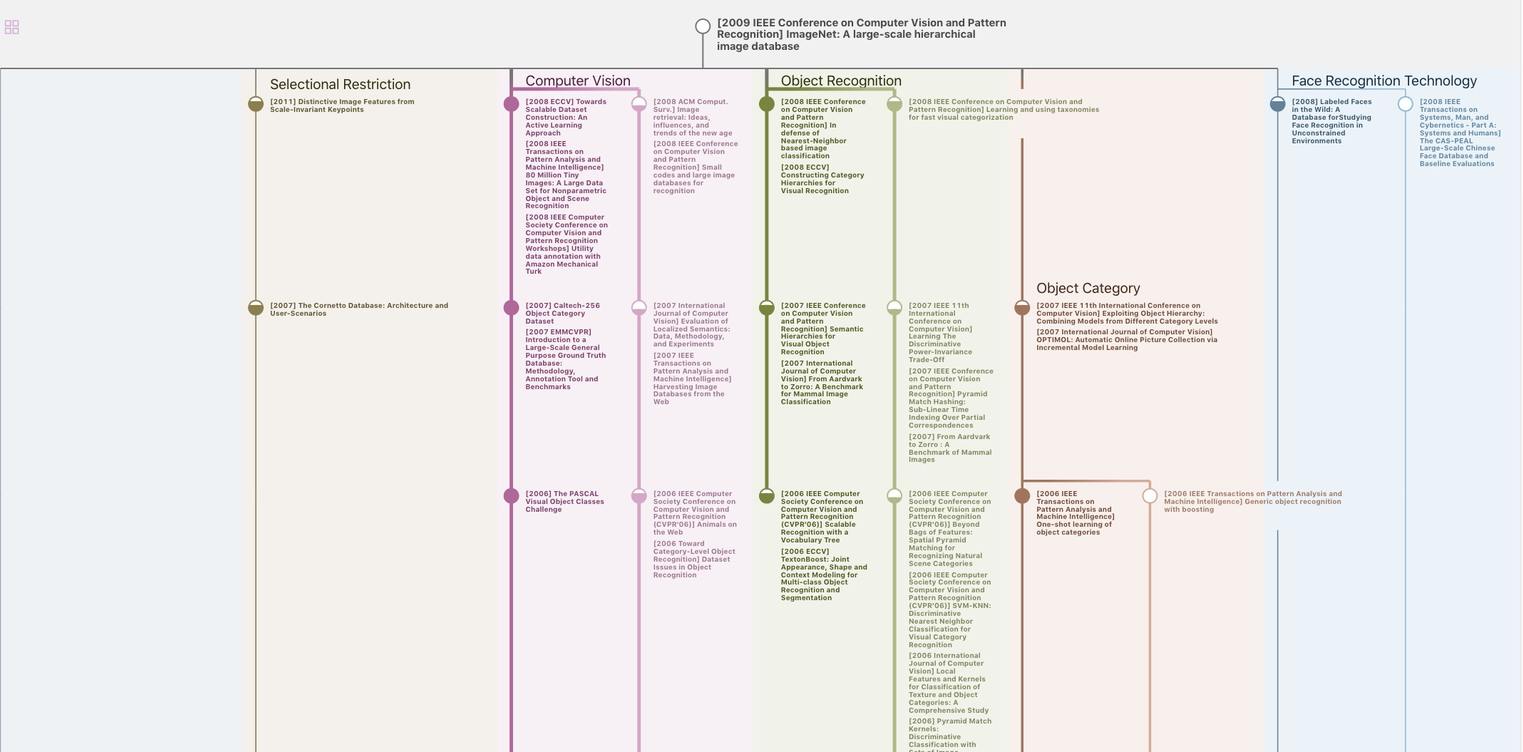
生成溯源树,研究论文发展脉络
Chat Paper
正在生成论文摘要