Investigating Imbalances Between SAR and Optical Utilization for Multi-Modal Urban Mapping
CoRR(2023)
摘要
Accurate urban maps provide essential information to support sustainable urban development. Recent urban mapping methods use multi-modal deep neural networks to fuse Synthetic Aperture Radar (SAR) and optical data. However, multi-modal networks may rely on just one modality due to the greedy nature of learning. In turn, the imbalanced utilization of modalities can negatively affect the generalization ability of a network. In this paper, we investigate the utilization of SAR and optical data for urban mapping. To that end, a dual-branch network architecture using intermediate fusion modules to share information between the uni-modal branches is utilized. A cut-off mechanism in the fusion modules enables the stopping of information flow between the branches, which is used to estimate the network's dependence on SAR and optical data. While our experiments on the SEN12 Global Urban Mapping dataset show that good performance can be achieved with conventional SAR-optical data fusion (F1 score = 0.682 $\pm$ 0.014), we also observed a clear under-utilization of optical data. Therefore, future work is required to investigate whether a more balanced utilization of SAR and optical data can lead to performance improvements.
更多查看译文
关键词
Remote sensing,deep learning,data fusion
AI 理解论文
溯源树
样例
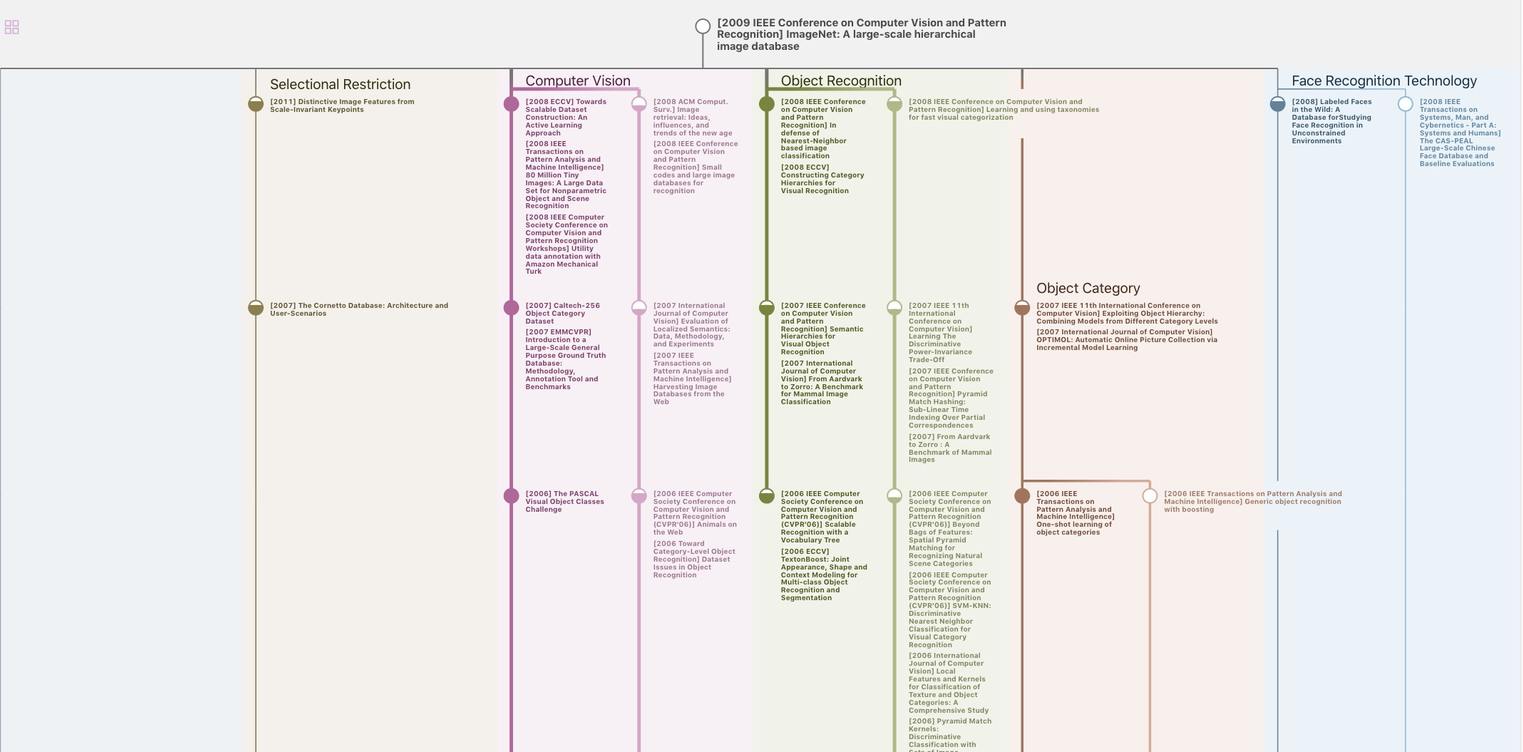
生成溯源树,研究论文发展脉络
Chat Paper
正在生成论文摘要