Adversarial Robustness of Phishing Email Detection Models
PROCEEDINGS OF THE 9TH ACM INTERNATIONAL WORKSHOP ON SECURITY AND PRIVACY ANALYTICS, IWSPA 2023(2023)
摘要
Developing robust detection models against phishing emails has long been the main concern of the cyber defense community. Currently, public phishing/legitimate datasets lack adversarial email examples which keeps the detection models vulnerable. To address this problem, we developed an augmented phishing/legitimate email dataset, utilizing different adversarial text attack techniques. Next, the models were retrained with the adversarial dataset. Results showed that accuracy and F1 score of the models improved under subsequent attacks. In another experiment, synthetic phishing emails were generated using a fine-tuned GPT-2 model. The detection model was retrained with a newly formed synthetic dataset. Subsequently, we observed that the accuracy and robustness of the model did not improve significantly under black box attack methods. In the last experiment, we proposed a defensive technique to classify adversarial examples to their true labels using a K-Nearest Neighbor approach with 94% accuracy in our prediction.
更多查看译文
关键词
phishing/legitimate dataset,adversarial attacks,data augmentation,model robustness,transformer models,deep learning,machine learning,generative AI,GPT-2
AI 理解论文
溯源树
样例
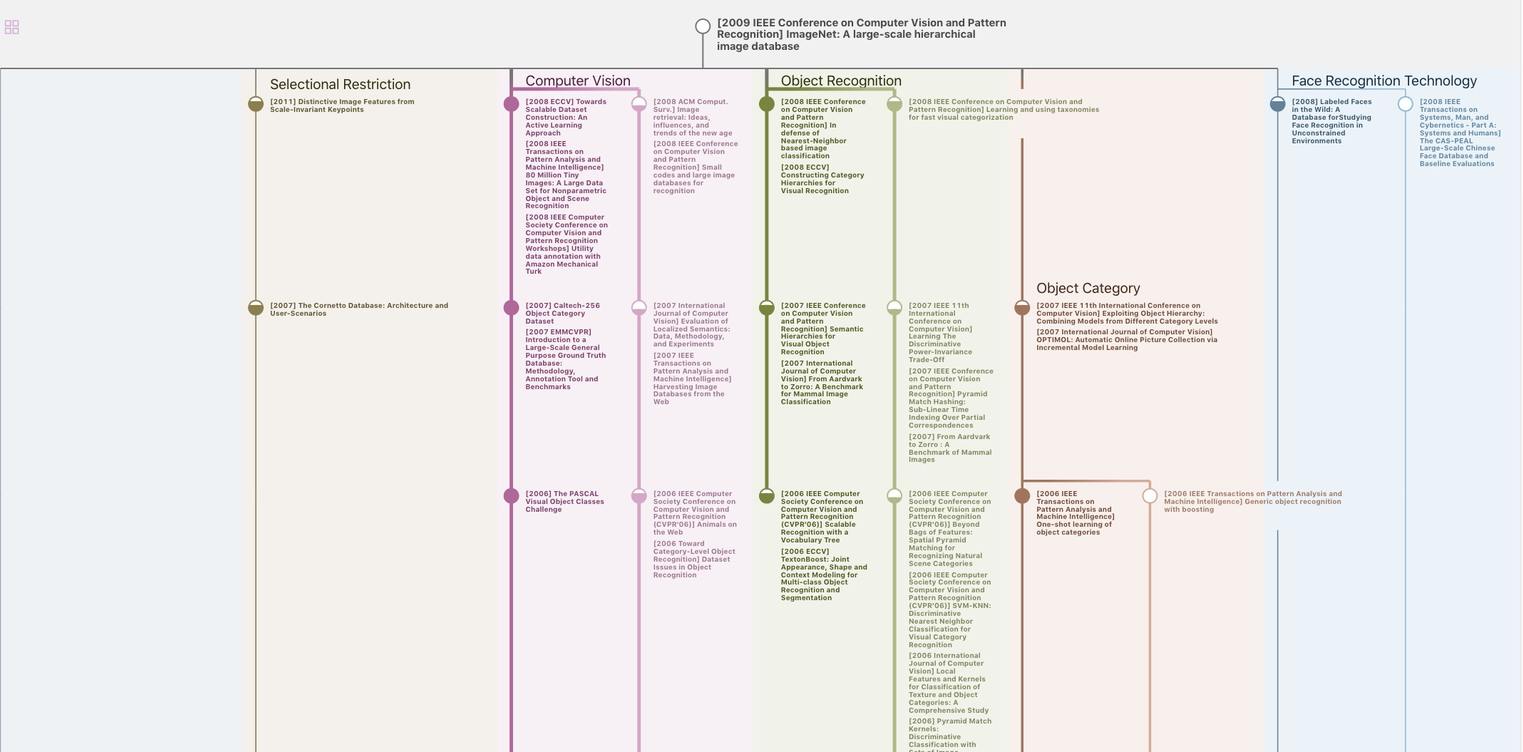
生成溯源树,研究论文发展脉络
Chat Paper
正在生成论文摘要