Active feature elicitation: An unified framework.
Frontiers in artificial intelligence(2023)
摘要
We consider the problem of active feature elicitation in which, given some examples with all the features (say, the full Electronic Health Record), and many examples with some of the features (say, demographics), the goal is to identify the set of examples on which more information (say, lab tests) need to be collected. The observation is that some set of features may be more expensive, personal or cumbersome to collect. We propose a classifier-independent, similarity metric-independent, general active learning approach which identifies examples that are dissimilar to the ones with the full set of data and acquire the complete set of features for these examples. Motivated by four real clinical tasks, our extensive evaluation demonstrates the effectiveness of this approach. To demonstrate the generalization capabilities of the proposed approach, we consider different divergence metrics and classifiers and present consistent results across the domains.
更多查看译文
关键词
active learning,classification,feature elicitation,healthcare,sample-efficiency
AI 理解论文
溯源树
样例
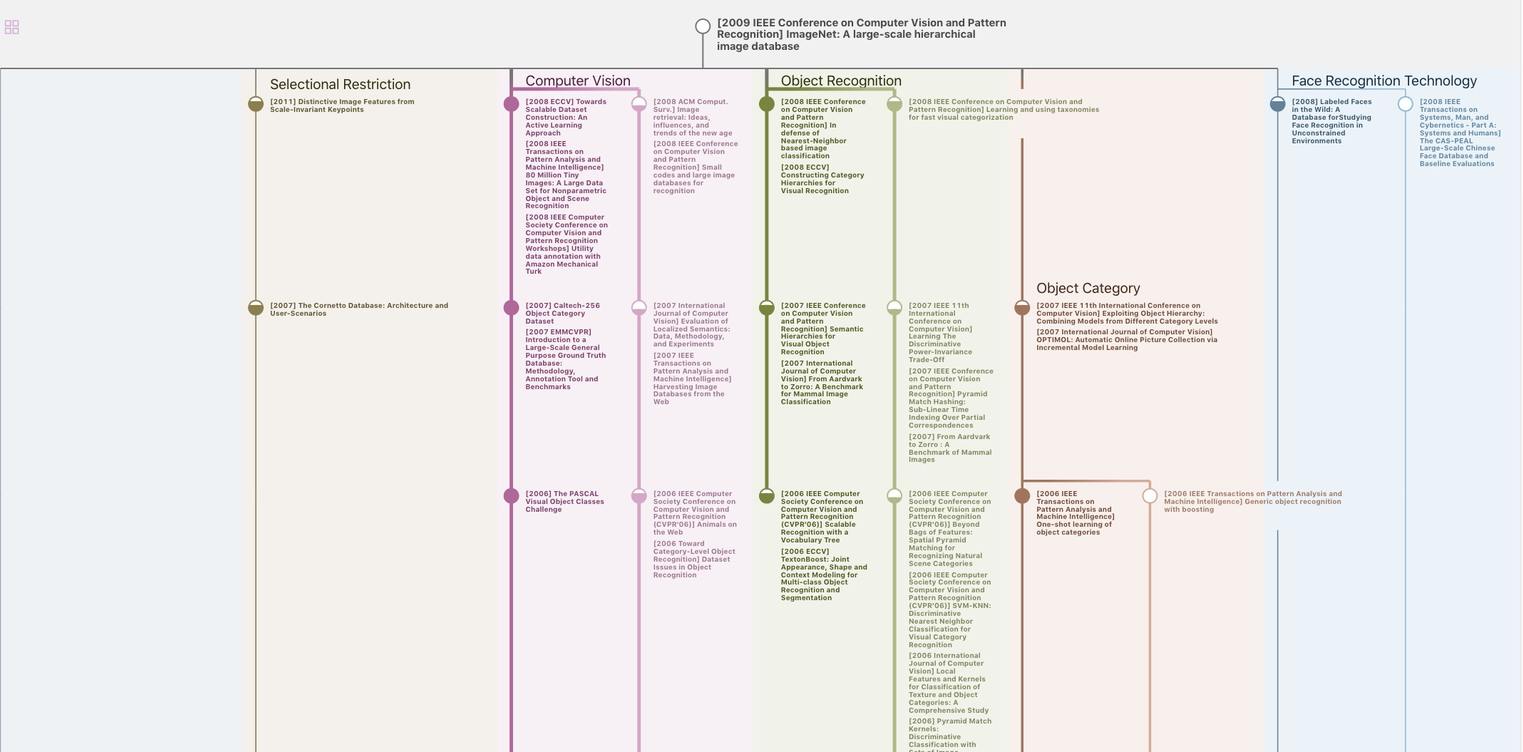
生成溯源树,研究论文发展脉络
Chat Paper
正在生成论文摘要