Best Arm Identification with Fairness Constraints on Subpopulations
2023 Winter Simulation Conference (WSC)(2023)
摘要
We formulate, analyze and solve the problem of best arm identification with fairness constraints on subpopulations (BAICS). Standard best arm identification problems aim at selecting an arm that has the largest expected reward where the expectation is taken over the entire population. The BAICS problem requires that an selected arm must be fair to all subpopulations (e.g., different ethnic groups, age groups, or customer types) by satisfying constraints that the expected reward conditional on every subpopulation needs to be larger than some thresholds. The BAICS problem aims at correctly identify, with high confidence, the arm with the largest expected reward from all arms that satisfy subpopulation constraints. We analyze the complexity of the BAICS problem by proving a best achievable lower bound on the sample complexity with closed-form representation. We then design an algorithm and prove that the algorithm's sample complexity matches with the lower bound in terms of order. A brief account of numerical experiments are conducted to illustrate the theoretical findings.
更多查看译文
关键词
Fairness Constraints,Arm Identification,Complex Problems,Lower Bound,Optimization Problem,Loss Of Generality,Proof Of Theorem,Research Problem,Kullback-Leibler,Feasible Set,Stopping Rule,Measure Of Confidence,Almost Surely,Exponential Family,Number Of Arms,Multi-armed Bandit
AI 理解论文
溯源树
样例
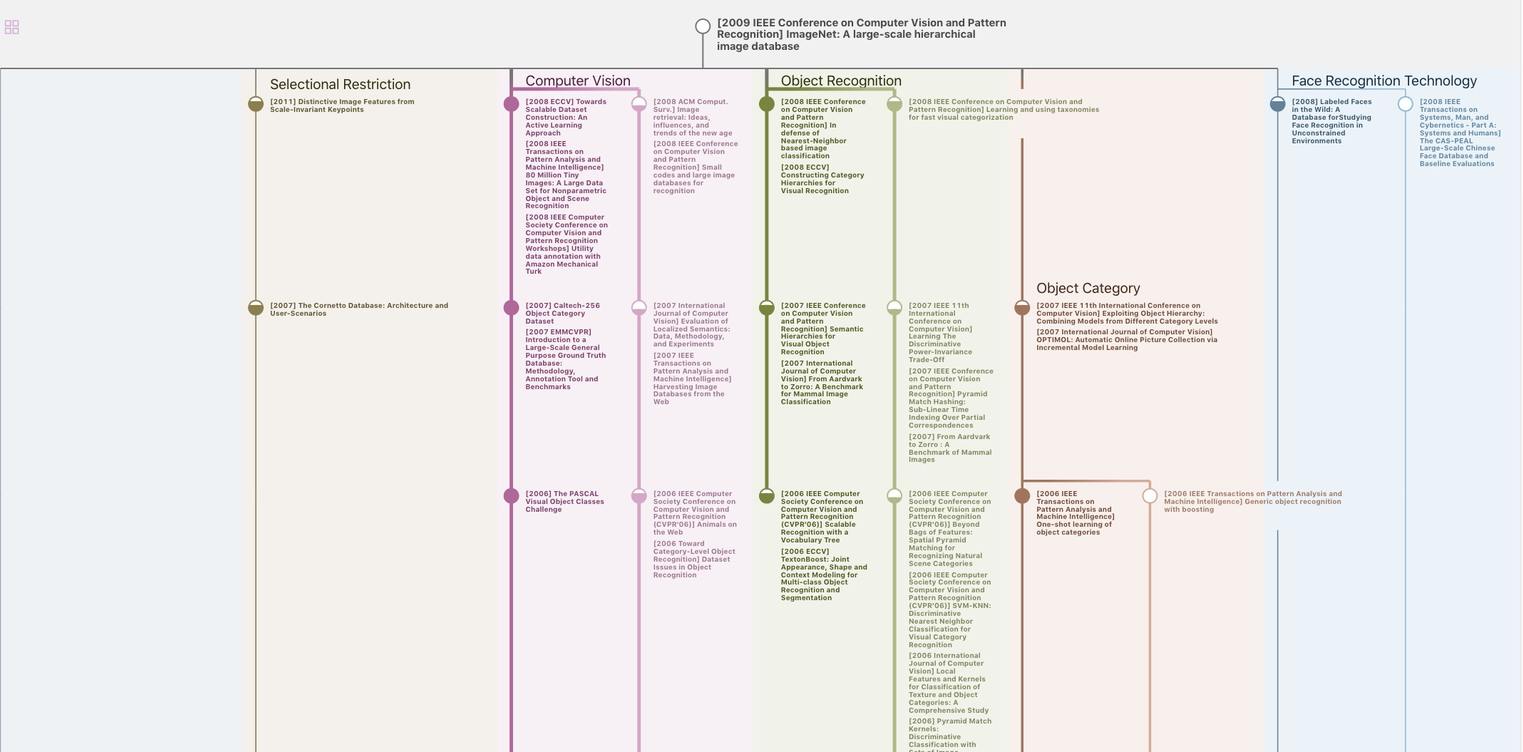
生成溯源树,研究论文发展脉络
Chat Paper
正在生成论文摘要