Improving Performance Insensitivity of Large-Scale Multiobjective Optimization via Monte Carlo Tree Search
IEEE TRANSACTIONS ON CYBERNETICS(2024)
摘要
The large-scale multiobjective optimization problem (LSMOP) is characterized by simultaneously optimizing multiple conflicting objectives and involving hundreds of decision variables. Many real-world applications in engineering can be modeled as LSMOPs; simultaneously, engineering applications require insensitivity in performance. This requirement typically means that the algorithm should not only produce good results in terms of performance for every run but also the performance of multiple runs should not fluctuate too much. However, existing large-scale multiobjective optimization algorithms often focus on improving algorithm performance, but pay little attention to improving the insensitivity characteristic of algorithms. This directly leads to substantial limitations when solving practical problems. In this work, we propose an evolutionary algorithm called large-scale multiobjective optimization algorithm via Monte Carlo tree search, which is based on the Monte Carlo tree search and aims to improve the performance and insensitivity of solving LSMOPs. The proposed method samples decision variables to construct new nodes on the Monte Carlo tree for optimization and evaluation, and it selects nodes with good evaluations for further searches in order to reduce the performance sensitivity caused by large-scale decision variables. We propose two metrics to measure the sensitivity of the algorithm and compare the proposed algorithm with several state-of-the-art designs on different benchmark functions and metrics. The experimental results confirm the effectiveness and performance insensitivity of the proposed design for solving LSMOPs.
更多查看译文
关键词
Optimization,Monte Carlo methods,Statistics,Sociology,Evolutionary computation,Search problems,Sensitivity,Evolutionary multiobjective optimization,insensitivity,large-scale optimization,Monte Carlo tree search (MCTS)
AI 理解论文
溯源树
样例
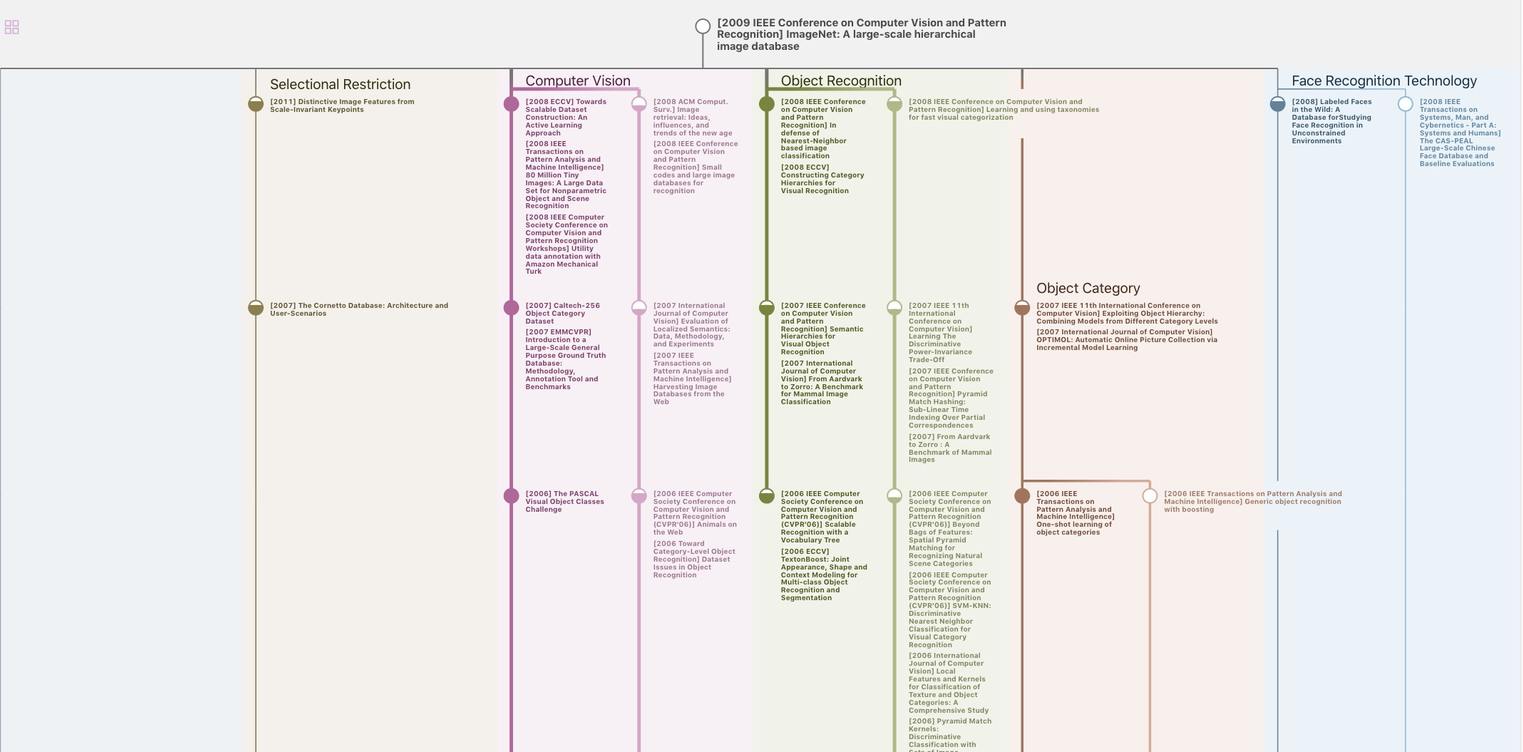
生成溯源树,研究论文发展脉络
Chat Paper
正在生成论文摘要