Training Instability and Disharmony Between ReLU and Batch Normalization
ICLR 2023(2023)
摘要
Deep neural networks based on batch normalization and ReLU-like activation functions experience instability during early stages of training owing to the high gradient induced by temporal gradient explosion. ReLU reduces the variance by more than the expected amount and batch normalization amplifies the gradient during its recovery. In this paper, we explain the explosion of a gradient mathematically while the forward propagation remains stable, and also the alleviation of the problem during training. Based on this, we propose a Layer-wise Asymmetric Learning rate Clipping (LALC) algorithm, which outperforms existing learning rate scaling methods in large batch training and can also be used to replace WarmUp in small batch training.
更多查看译文
关键词
Deep learning,Gradient Exploding,ReLU,Batch normalization,Training instability,LARS,WarmUp
AI 理解论文
溯源树
样例
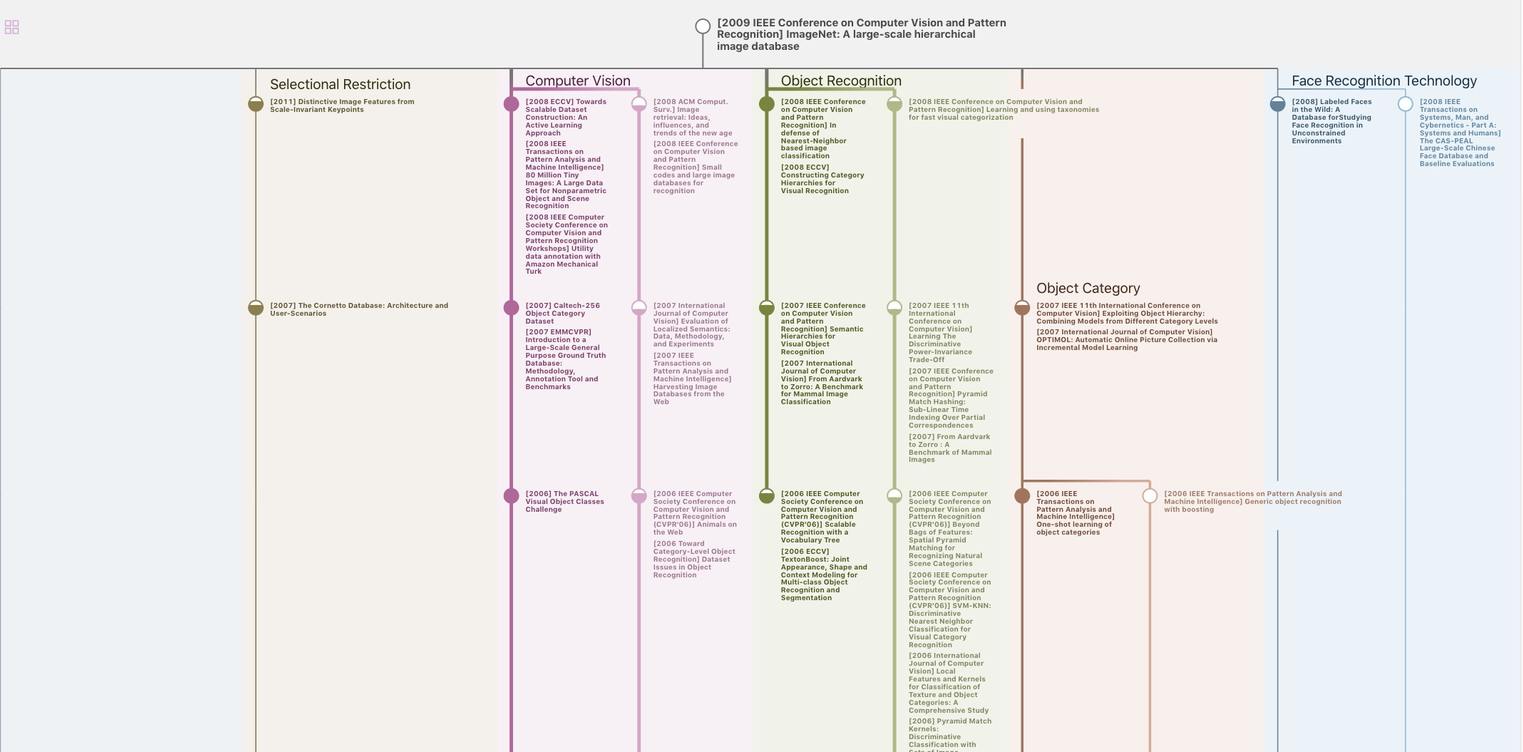
生成溯源树,研究论文发展脉络
Chat Paper
正在生成论文摘要