Graph Neural Bandits
KDD 2023(2023)
摘要
Contextual bandits algorithms aim to choose the optimal arm with the highest reward out of a set of candidates based on the contextual information. Various bandit algorithms have been applied to real-world applications due to their ability of tackling the exploitation-exploration dilemma. Motivated by online recommendation scenarios, in this paper, we propose a framework named Graph Neural Bandits (GNB) to leverage the collaborative nature among users empowered by graph neural networks (GNNs). Instead of estimating rigid user clusters as in existing works, we model the "fine-grained" collaborative effects through estimated user graphs in terms of exploitation and exploration respectively. Then, to refine the recommendation strategy, we utilize separate GNN-based models on estimated user graphs for exploitation and adaptive exploration. Theoretical analysis and experimental results on multiple real data sets in comparison with state-of-the-art baselines are provided to demonstrate the effectiveness of our proposed framework.
更多查看译文
关键词
Contextual Bandits,User Modeling,Graph Neural Networks
AI 理解论文
溯源树
样例
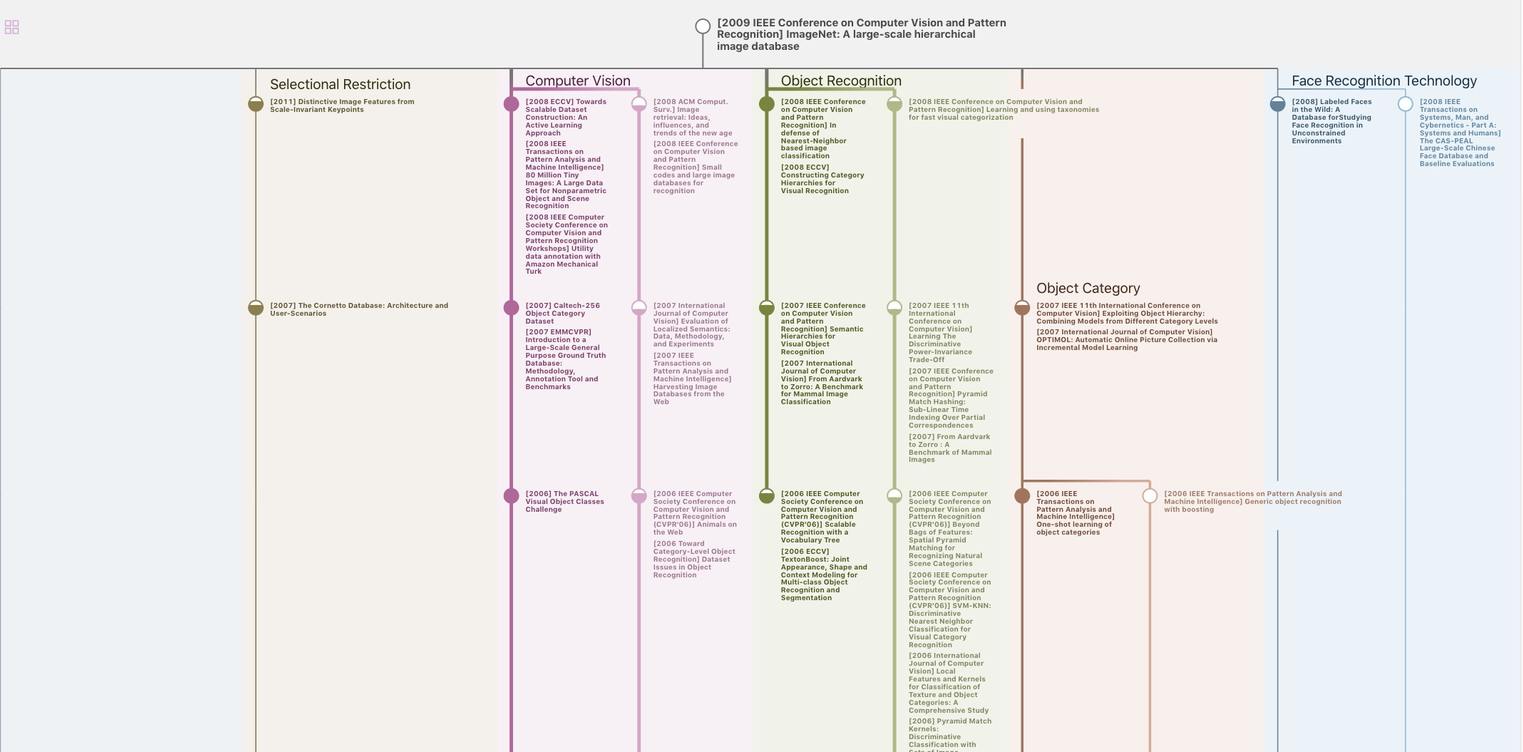
生成溯源树,研究论文发展脉络
Chat Paper
正在生成论文摘要