Improved Fully Quantized Training via Rectifying Batch Normalization
ICLR 2023(2023)
摘要
Quantization-aware Training (QAT) is able to reduce the training cost by quantizing neural network weights and activations in the forward pass and improve the speed at the inference stage. QAT can be extended to Fully-Quantized Training (FQT), which further accelerates the training by quantizing gradients in the backward pass as back-propagation typically occupies half of the training time. Unfortunately, gradient quantization is challenging as Stochastic Gradient Descent (SGD) based training is sensitive to the precision of the gradient signal. Particularly, the noise introduced by gradient quantization accumulates during backward pass, which causes the exploding gradient problem and results in unstable training and significant accuracy drop. Though Batch Normalization (BatchNorm) is a de-facto resort to stabilize training in regular full-precision scenario, we observe that it fails to prevent the gradient explosion when gradient quantizers are injected in the backward pass. Surprisingly, our theory shows that BatchNorm could amplify the noise accumulation, which in turn hastens the explosion of gradients. A BatchNorm rectification method is derived from our theory to suppress the amplification effect and bridge the performance gap between full-precision training and FQT. Adding this simple rectification loss to baselines generates better results than most prior FQT algorithms on various neural network architectures and datasets, regardless of the gradient bit-widths used (8,4, and 2 bits).
更多查看译文
关键词
Model Compression,Gradient Quantization,Convolution Neural Networks,Batch Normalization
AI 理解论文
溯源树
样例
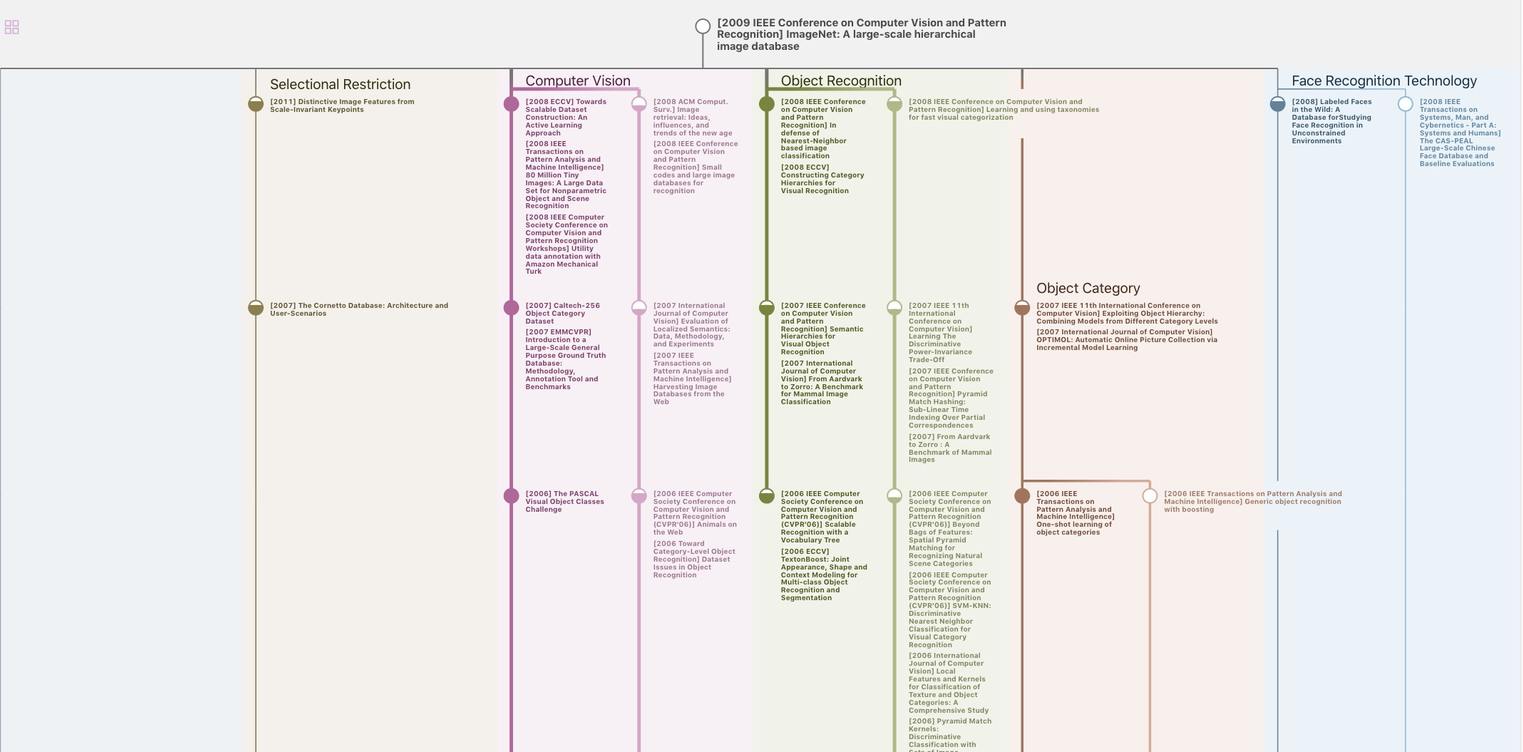
生成溯源树,研究论文发展脉络
Chat Paper
正在生成论文摘要