Structural Generalization of Visual Imitation Learning with Position-Invariant Regularization
ICLR 2023(2023)
摘要
How the visual imitation learning models can generalize to novel unseen visual observations is a highly challenging problem. Such a generalization ability is very crucial for their real-world applications. Since this generalization problem has many different aspects, we focus on one case called structural generalization, which refers to generalization to unseen task setup, such as a novel setup of object locations in the robotic manipulation problem. In this case, previous works observe that the visual imitation learning models will overfit to the absolute information (e.g., coordinates) rather than the relational information between objects, which is more important for decision making. As a result, the models will perform poorly in novel scenarios. Nevertheless, so far, it remains unclear how we can solve this problem effectively. Our insight into this problem is to explicitly remove the absolute information from the features learned by imitation learning models so that the models can use robust, relational information to make decisions. To this end, we propose a novel, position-invariant regularizer for generalization. The proposed regularizer will penalize the imitation learning model when its features contain absolute, positional information of objects. We carry out experiments on the MAGICAL and ProcGen benchmark, as well as a real-world robot manipulation problem. We find that our regularizer can effectively boost the structural generalization performance of imitation learning models. Through both qualitative and quantitative analysis, we verify that our method does learn robust relational representations.
更多查看译文
AI 理解论文
溯源树
样例
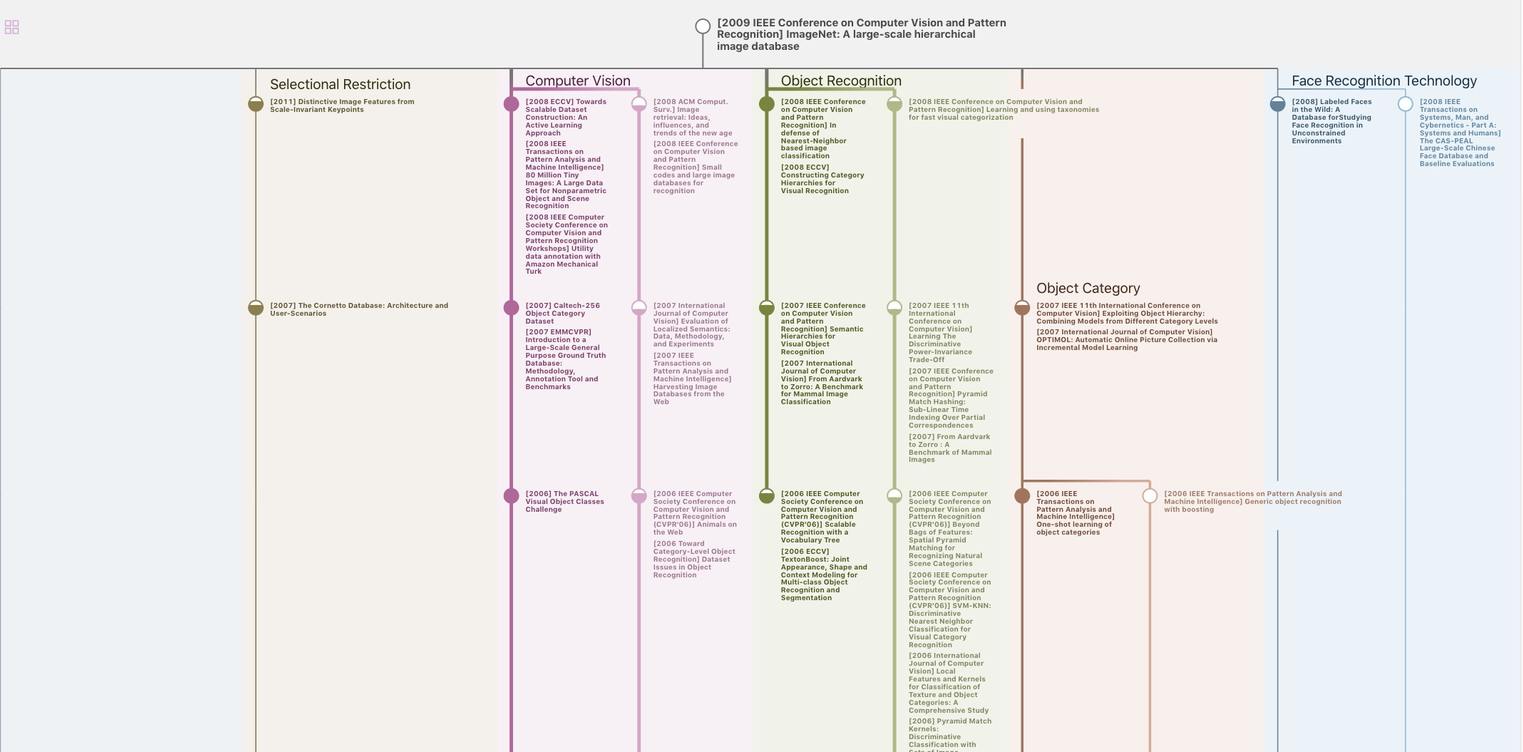
生成溯源树,研究论文发展脉络
Chat Paper
正在生成论文摘要