Communication-Efficient Federated Learning with Accelerated Client Gradient
ICLR 2023(2024)
摘要
Federated learning often suffers from slow and unstable convergence due to heterogeneous characteristics of participating client datasets.
Such a tendency is aggravated when the client participation ratio is low since the information collected from the clients is prone to have large variations.
To tackle this challenge, we propose a novel federated learning framework, which improves the consistency across clients and facilitates the convergence of the server model.
This is achieved by making the server broadcast a global model with a gradient acceleration.
By adopting the strategy, the proposed algorithm conveys the projective global update information to participants effectively with no extra communication cost and relieves the clients from storing the previous models.
We also regularize local updates by aligning each of the clients with the overshot global model to reduce bias and improve the stability of our algorithm.
We perform comprehensive empirical studies on real data under various settings and demonstrate remarkable performance gains of the proposed method in terms of accuracy and communication efficiency compared to the state-of-the-art methods, especially with low client participation rates.
We will release our code to facilitate and disseminate our work.
更多查看译文
关键词
Federated learning,Data heterogeneity,Deep Neural Networks,Distributed Optimization
AI 理解论文
溯源树
样例
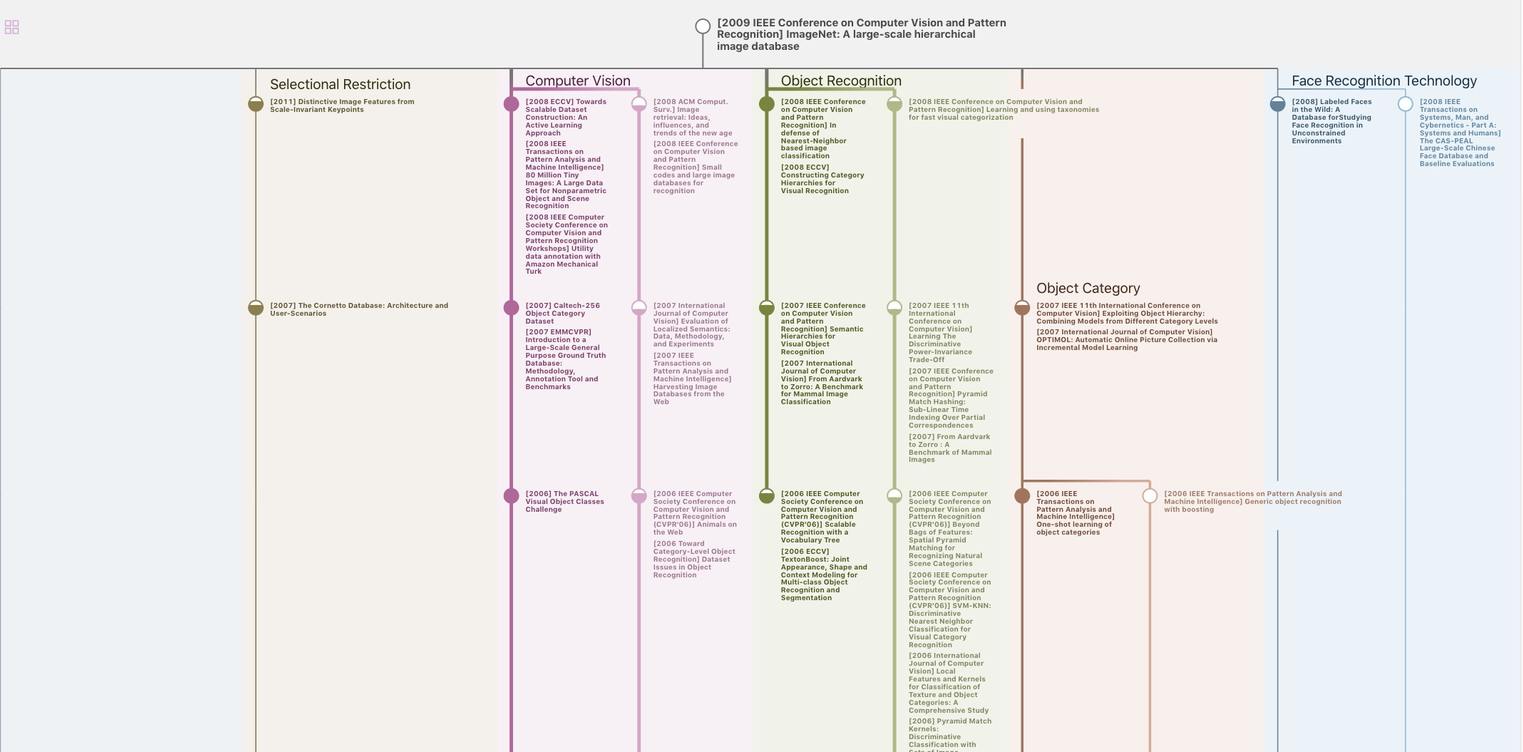
生成溯源树,研究论文发展脉络
Chat Paper
正在生成论文摘要