Learning Object Affordance with Contact and Grasp Generation
ICLR 2023(2023)
摘要
Understanding object affordance can help in designing better and more robust robotic grasping. Existing work in the computer vision community formulates the object affordance understanding as a grasping pose generation problem, which treats the problem as a black box by learning a mapping between objects and the distributions of possible grasping poses for the objects. On the other hand, in the robotics community, estimating object affordance represented by contact maps is of the most importance as localizing the positions of the possible affordance can help the planning of grasping actions. In this paper, we propose to formulate the object affordance understanding as both contacts and grasp poses generation. we factorize the learning task into two sequential stages, rather than the black-box strategy: (1) we first reason the contact maps by allowing multi-modal contact generation; (2) assuming that grasping poses are fully constrained given contact maps, we learn a one-to-one mapping from the contact maps to the grasping poses. Further, we propose a penetration-aware partial optimization from the intermediate contacts. It combines local and global optimization for the refinement of the partial poses of the generated grasps exhibiting penetration. Extensive validations on two public datasets show our method outperforms state-of-the-art methods regarding grasp generation on various metrics.
更多查看译文
关键词
Object Affordance,Hand Grasps Generation,Conditional Variational Autoencoder,DLearning
AI 理解论文
溯源树
样例
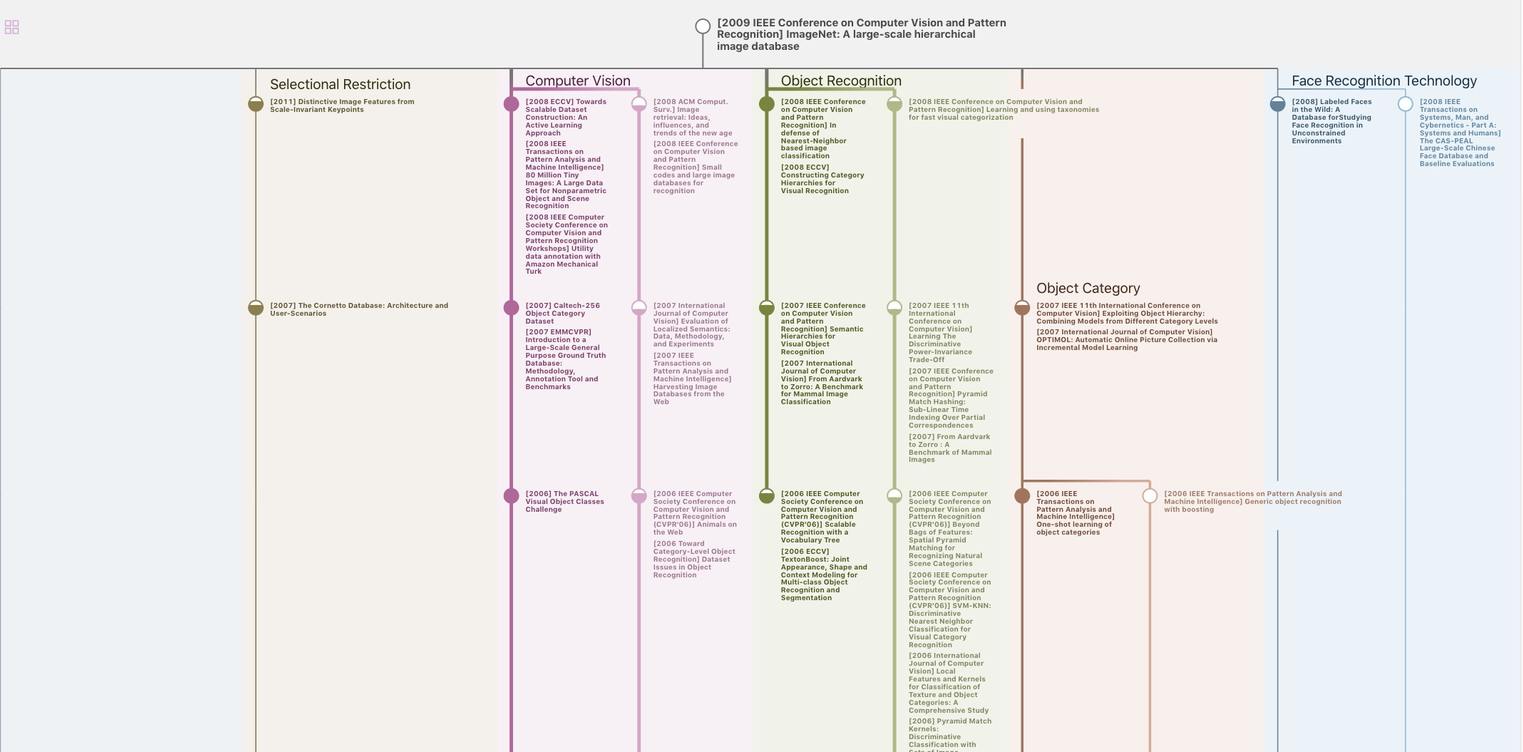
生成溯源树,研究论文发展脉络
Chat Paper
正在生成论文摘要