Dense Correlation Fields for Motion Modeling in Action Recognition
ICLR 2023(2023)
摘要
The challenge of action recognition is to capture reasoning motion information. Compared to spatial convolution for appearance, the temporal component provides an additional (and important) clue for motion modeling, as a number of actions can be reliably recognized based on the motion information. In this paper, we present an effective and interpretable module, Dense Correlation Fields (DCF), which builds up dense visual correlation volumes at the feature level to model different motion patterns explicitly. To achieve this goal, we rely on a spatially hierarchical architecture that preserves both fine local information provided in the lower layer and the high-level semantic information from the deeper layer. Our method fuses spatial hierarchical correlation and temporal long-term correlation, which is better suited for small objects and large displacements. This module is extensible and can be plugged into many backbone architectures to accurately predict object interactions in the video. DCF shows consistent improvements over 2D CNNs and 3D CNNs baseline networks with 3.7% and 3.0% gains respectively on the standard video action benchmark of SSV1.
更多查看译文
关键词
Action recognition,Motion modeling,Video understanding
AI 理解论文
溯源树
样例
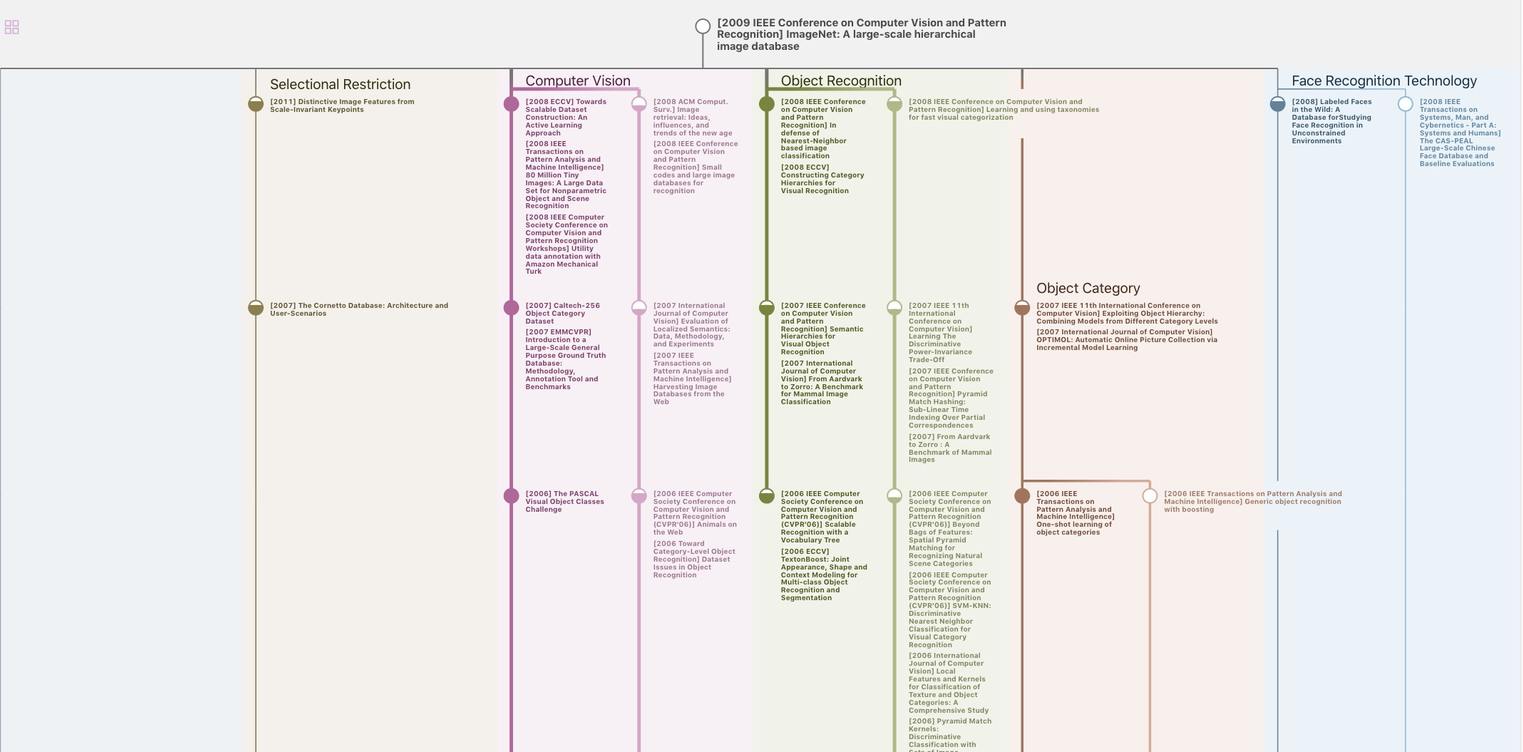
生成溯源树,研究论文发展脉络
Chat Paper
正在生成论文摘要