Learning to mine approximate network motifs
ICLR 2023(2023)
摘要
Frequent and structurally related subgraphs, also known as network motifs, are valuable features of many datasets. However, strong combinatorial bottlenecks have made it difficult to extract motifs and use them in learning tasks without strong constraints on the motif properties. In this work we propose a representation learning method based on learnable graph coarsening, MotiFiesta which is the first to be able to extract large and approximate motifs in a fully differentiable manner. We build benchmark datasets and evaluation metrics which test the ability our proposed and future models to capture different aspects of motif discovery where ground truth motifs are not known. Finally, explore the notion of exploiting learned motifs as an inductive bias in real-world datasets by showing competitive performance on motif-based featuresets with established real-world benchmark datasets against concurrent architectures.
更多查看译文
关键词
motif mining,combinatorics,unsupervised learning
AI 理解论文
溯源树
样例
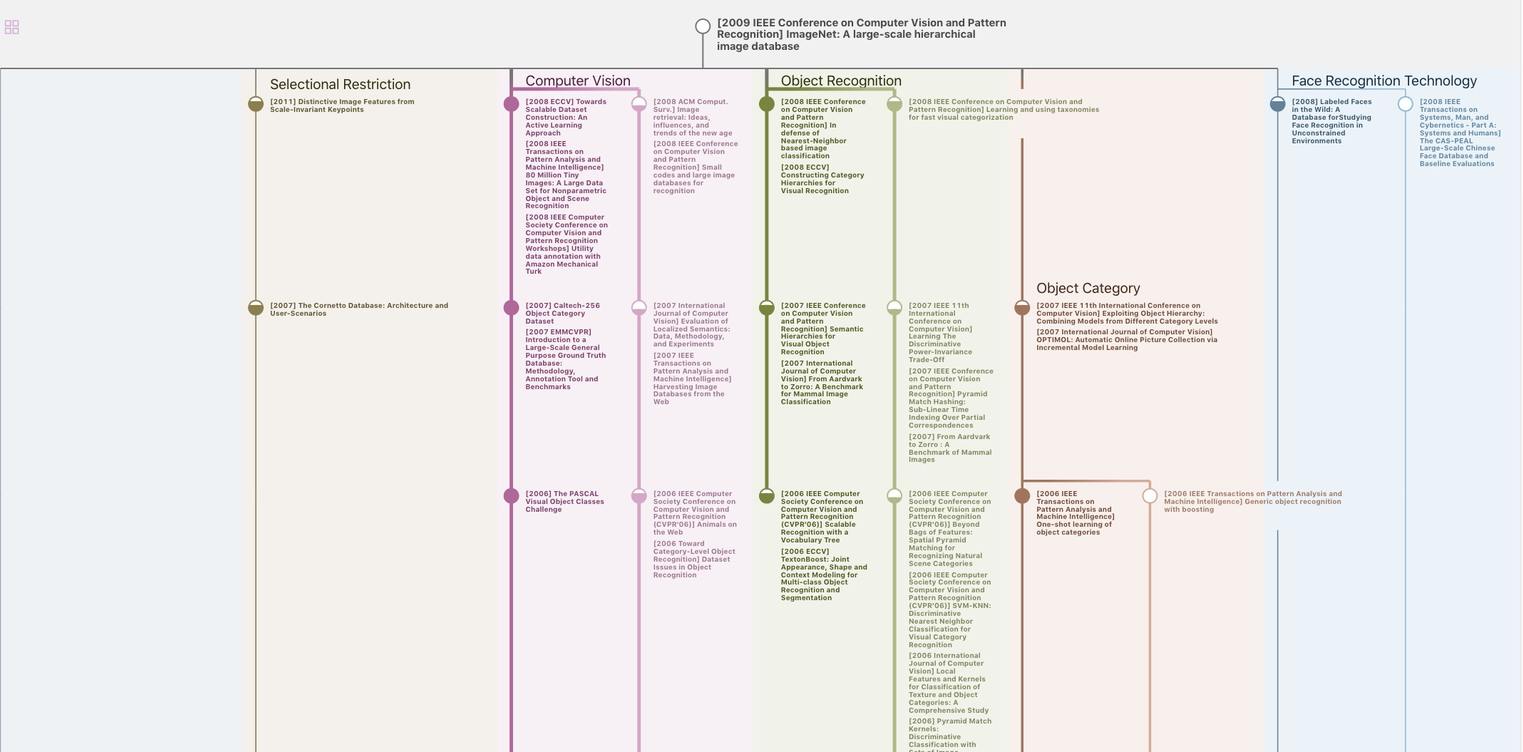
生成溯源树,研究论文发展脉络
Chat Paper
正在生成论文摘要