Multitask Reinforcement Learning by Optimizing Neural Pathways
ICLR 2023(2023)
摘要
Reinforcement learning (RL) algorithms have achieved great success in learning specific tasks, as evidenced by examples such as AlphaGo or fusion control. However, it is still difficult for an RL agent to learn how to solve multiple tasks. In this paper, we propose a novel multitask learning framework, in which multiple specialized pathways through a single network are trained simultaneously, with each pathway focusing on a single task. We show that this approach achieves competitive performance with existing multitask RL methods, while using only 5% of the number of neurons per task. We demonstrate empirically the success of our approach on several continuous control tasks, in both online and offline training.
更多查看译文
关键词
Multitask Learning,Online Reinforcement Learning,Offline Reinforcement Learning,Neural Pathways
AI 理解论文
溯源树
样例
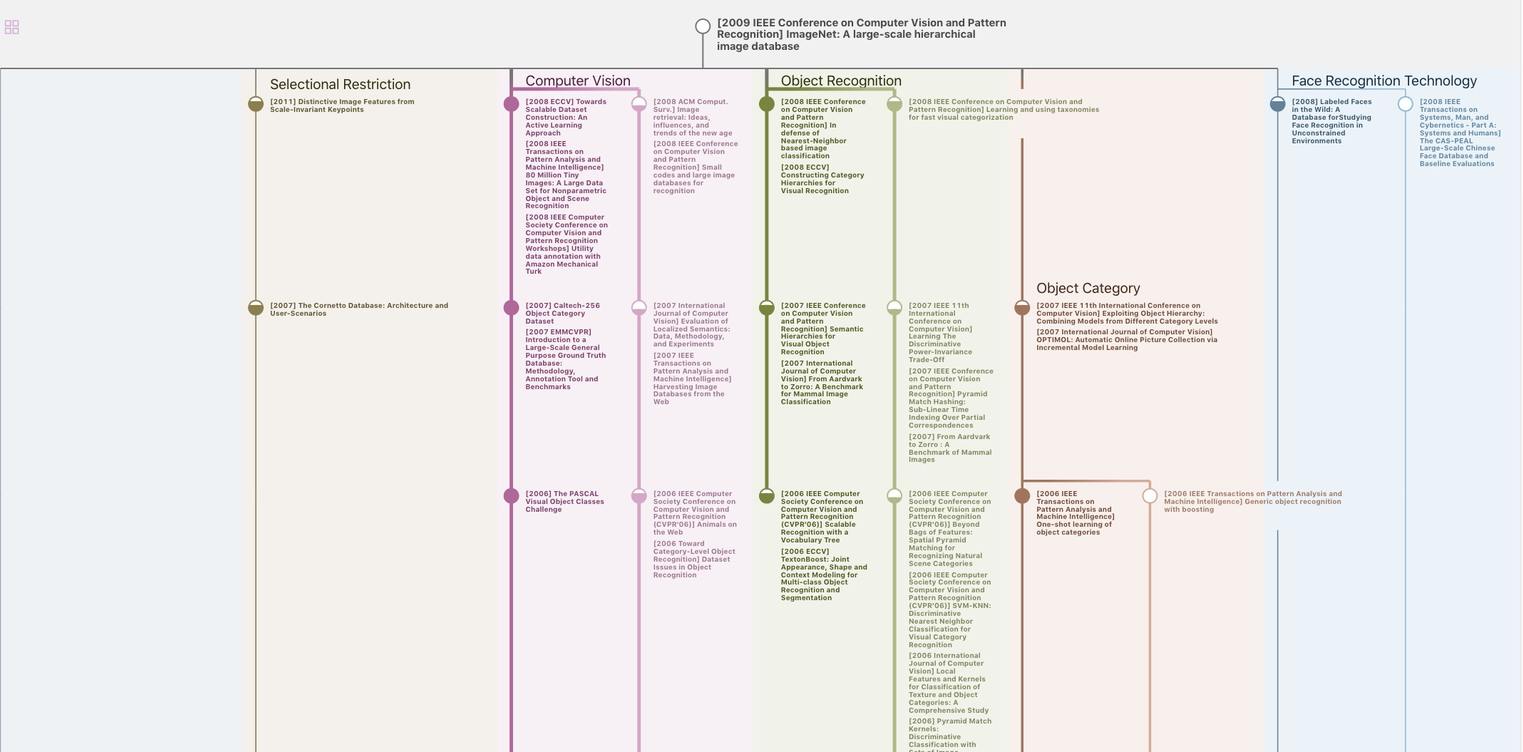
生成溯源树,研究论文发展脉络
Chat Paper
正在生成论文摘要