GOING BEYOND 1-WL EXPRESSIVE POWER WITH 1-LAYER GRAPH NEURAL NETWORKS
ICLR 2023(2023)
摘要
Graph neural networks have become the \textit{de facto} standard for representational learning in graphs, and have achieved SOTA in many graph-related tasks such as node classification, graph classification and link prediction. However, it has been shown that the expressive power is equivalent maximally to Weisfeiler-Lehman Test. Recently, there is a line of work aiming to enhance the expressive power of graph neural networks. In this work, we propose a more generalized variant of neural Weisfeiler-Lehman test to enhance structural representation for each node in a graph to uplift the expressive power of any graph neural network. It is shown theoretically our method is strictly more powerful than 1\&2-WL test. The Numerical experiments also demonstrate that our proposed method outperforms the standard GNNs on almost all the benchmark datasets by a large margin in most cases with significantly lower running time and memory consumption compared with other more powerful GNNs.
更多查看译文
关键词
graph neural networks,expressivity,memory-efficient
AI 理解论文
溯源树
样例
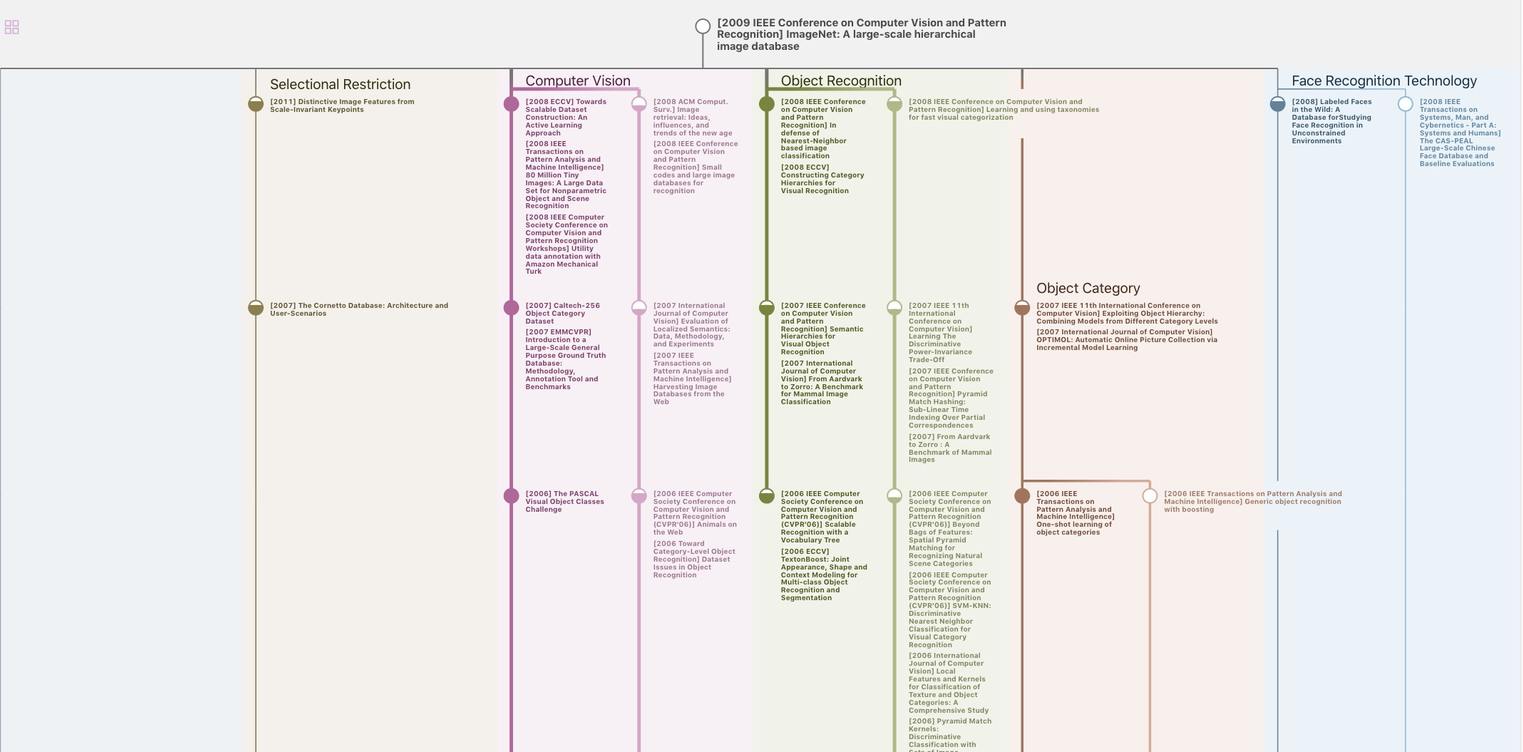
生成溯源树,研究论文发展脉络
Chat Paper
正在生成论文摘要