A Deep Learning Framework for Musical Acoustics Simulations
ICLR 2023(2023)
摘要
The acoustic modeling of musical instruments is a heavy computational process, often bound to the solution of complex systems of partial differential equations (PDEs). Numerical models can achieve a high level of accuracy, but they may take up to several hours to complete a full simulation, especially in the case of intricate musical mechanisms. The application of deep learning, and in particular of neural operators that learn mappings between function spaces, has the potential to revolutionize how acoustics PDEs are solved and noticeably speed up musical simulations. However, such operators require large datasets, capable of exemplifying the relationship between input parameters (excitation) and output solutions (acoustic wave propagation) per each target musical instrument/configuration. With this work, we present an open-access, open-source framework designed for the generation of numerical musical acoustics datasets and for the training/benchmarking of acoustics neural operators. We first describe the overall structure of the framework and the proposed data generation workflow. Then, we detail the first numerical models that were ported to the framework. Finally, we conclude by sharing some preliminary results obtained by means of training a state-of-the-art neural operator with a dataset generated via the framework. This work is a first step towards the gathering of a research community that focuses on deep learning applied to musical acoustics, and shares workflows and benchmarking tools.
更多查看译文
关键词
Datasets,acoustics simulation,numerical modeling,deep learning,neural operators,benchmarking
AI 理解论文
溯源树
样例
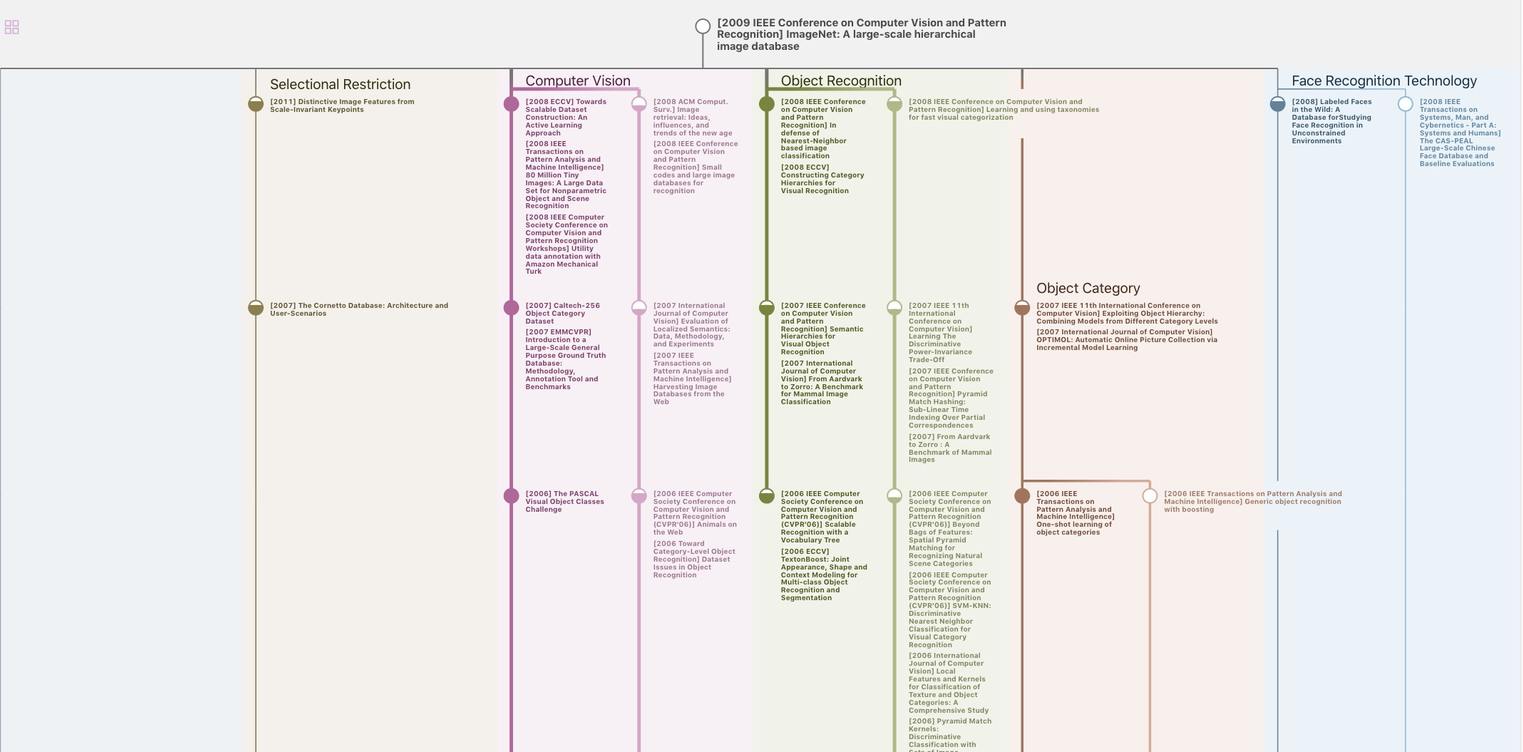
生成溯源树,研究论文发展脉络
Chat Paper
正在生成论文摘要