DeepGuiser: Learning to Disguise Neural Architectures for Impeding Adversarial Transfer Attacks
ICLR 2023(2023)
摘要
Security is becoming increasingly critical in deep learning applications. Recent researches demonstrate that NN models are vulnerable to adversarial attacks, which can mislead them with only small input perturbations. Moreover, adversaries who know the architecture of victim models can conduct more effective attacks. Unfortunately, the architectural knowledge can usually be stolen by the adversaries by exploiting the system-level hints through many side channels, which is referred to as the neural architecture extraction attack. Conventional countermeasures for neural architecture extraction can introduce large overhead, and different hardware platforms have diverse types of side-channel leakages such that many expert efforts are needed in developing hardware-specific countermeasures. In this paper, we propose DeepGuiser, an automatic, hardware-agnostic, and retrain-free neural architecture disguising method, to disguise the neural architectures to reduce the harm of neural architecture extraction attacks. In a nutshell, given a trained model, DeepGuiser outputs a deploy model that is functionally equivalent with the trained model but with a different (i.e., disguising) architecture. DeepGuiser can minimize the harm of the follow-up adversarial transfer attacks to the deploy model, even if the disguising architecture is completely stolen by the architecture extraction attack. Experiments demonstrate that DeepGuiser can effectively disguise diverse architectures and impede the adversarial transferability by 13.87% ∼ 32.59%, while only introducing 10% ∼ 40% extra inference latency.
更多查看译文
关键词
Neural architecture extraction attack,neural architecture disguising,adversarial robustness,transferability predictor,policy learning
AI 理解论文
溯源树
样例
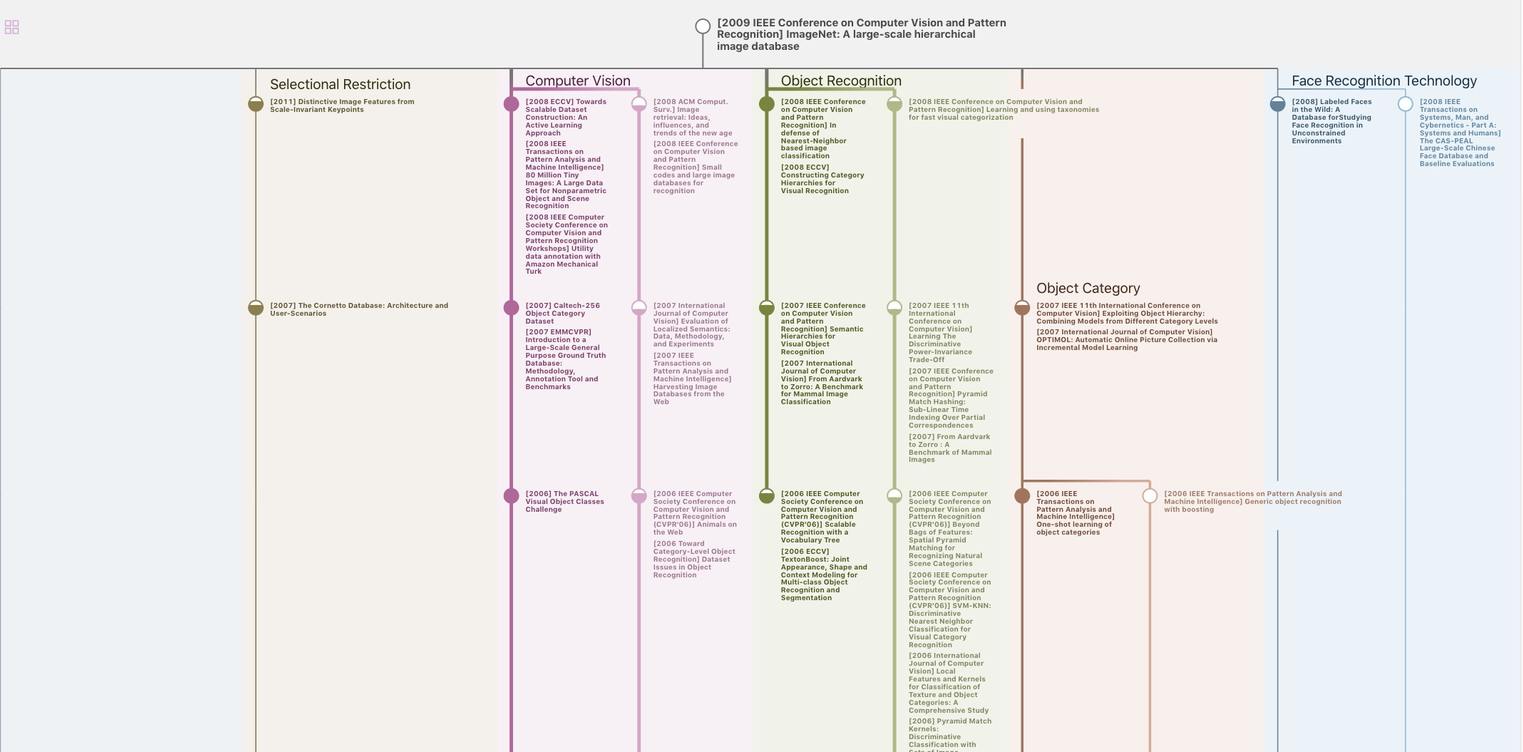
生成溯源树,研究论文发展脉络
Chat Paper
正在生成论文摘要