Dynamical Signatures of Learning in Recurrent Networks
ICLR 2023(2023)
摘要
Recurrent neural networks (RNNs) are powerful computational tools that operate best near the edge of chaos, where small perturbations in neuronal firing are transmitted between neurons with minimal amplification or loss. In this article, we depart from the observation that both stimulus and noise can be seen as perturbations to the intrinsic dynamics of a recurrent network, however stimulus information must be reliably preserved, while noise must be discarded. First, we show that self-organizing recurrent networks (SORNs) that learn the spatio-temporal structure of their inputs, increase their recurrent memory by preferentially propagating the relevant stimulus-specific structured signals, while becoming more robust to random perturbation. We find that the computational advantages gained through self-supervised learning are accompanied by a shift from critical to ordered dynamics, and that this dynamical shift varies with the structure of the stimulus. Next, we show that SORNs with subcritical dynamics can outperform their random RNNs counterparts with critical dynamics, on a range of tasks, including a temporal MNIST and a sequential shape-rotation task. Interestingly, when a shape is rotated, both the invariant (shape) and the variant (motion direction) aspects of the stimulus sequence are improved through learning in the subcritical SORNs. We propose that the shift in criticality is a signature of specialization and we expect it to be found in all cases in which general-purpose recurrent networks acquire self-correcting properties by internalizing the statistical structure of their inputs.
更多查看译文
关键词
RNNs,self-organization,criticality,spatio-temporal dynamics
AI 理解论文
溯源树
样例
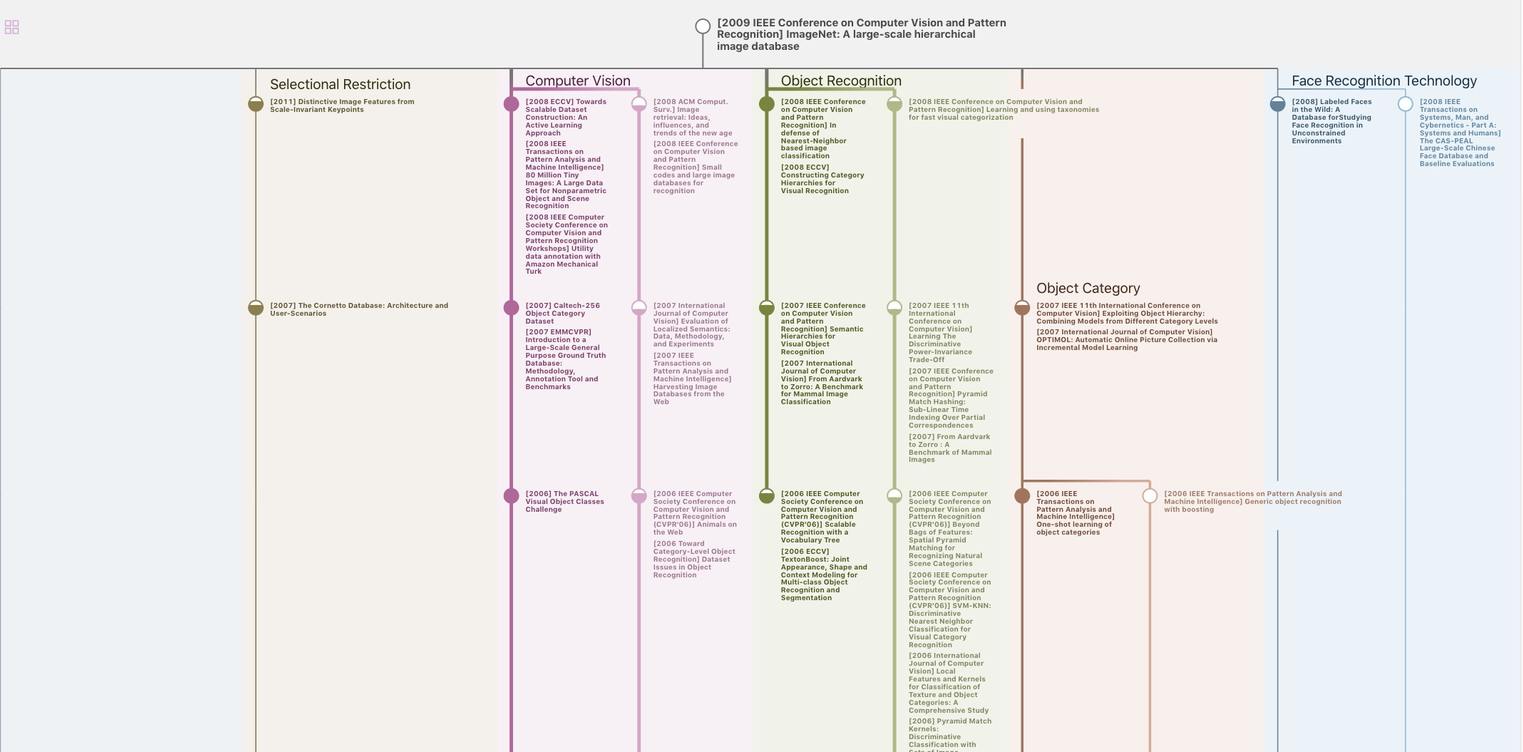
生成溯源树,研究论文发展脉络
Chat Paper
正在生成论文摘要