Lmser-pix2seq: Learning stable sketch representations for sketch healing
COMPUTER VISION AND IMAGE UNDERSTANDING(2024)
摘要
Sketch healing aims to recreate a complete sketch from the corrupted one. Sketches are abstract and sparse, making it difficult for neural networks to learn high -quality representations of sketches that include colors, textures, and other details. This presents a significant challenge for sketch healing. The features extracted from the corrupted sketch may be inconsistent with the ones from the corresponding full sketch. In this paper, we present Lmser-pix2seq to learn stable sketch representations against the missing information by employing a Least mean square error reconstruction (Lmser) block, which falls into encoder-decoder paradigm. Taking as input a corrupted sketch, the Lmser encoder computes the embeddings of structural patterns of the input, while the decoder reconstructs the complete sketch from the embeddings. We build bi-directional skip connections between the encoder and the decoder in our Lmser block. The feedback connections enable recurrent paths to receive more information about the reconstructed sketch produced by the decoder, which helps the encoder extract stable sketch features. The features captured by the Lmser block are eventually fed into a recurrent neural network decoder to recreate the sketches. We also find that compared with the vanilla convolutional neural networks, our gated multilayer perceptron (gMLP) block based network captures the long-range dependence of different regions in the sketch image, automatically learns the relationship between patches and extracts the individual -specific features from the sketch more effectively. Experimental results show that our Lmser-pix2seq outperforms the state-of-the-art methods in sketch healing, especially when the sketches are heavily masked or corrupted.
更多查看译文
关键词
Sketch healing,Lmser,Stable sketch representations,Bi-directional skip connections
AI 理解论文
溯源树
样例
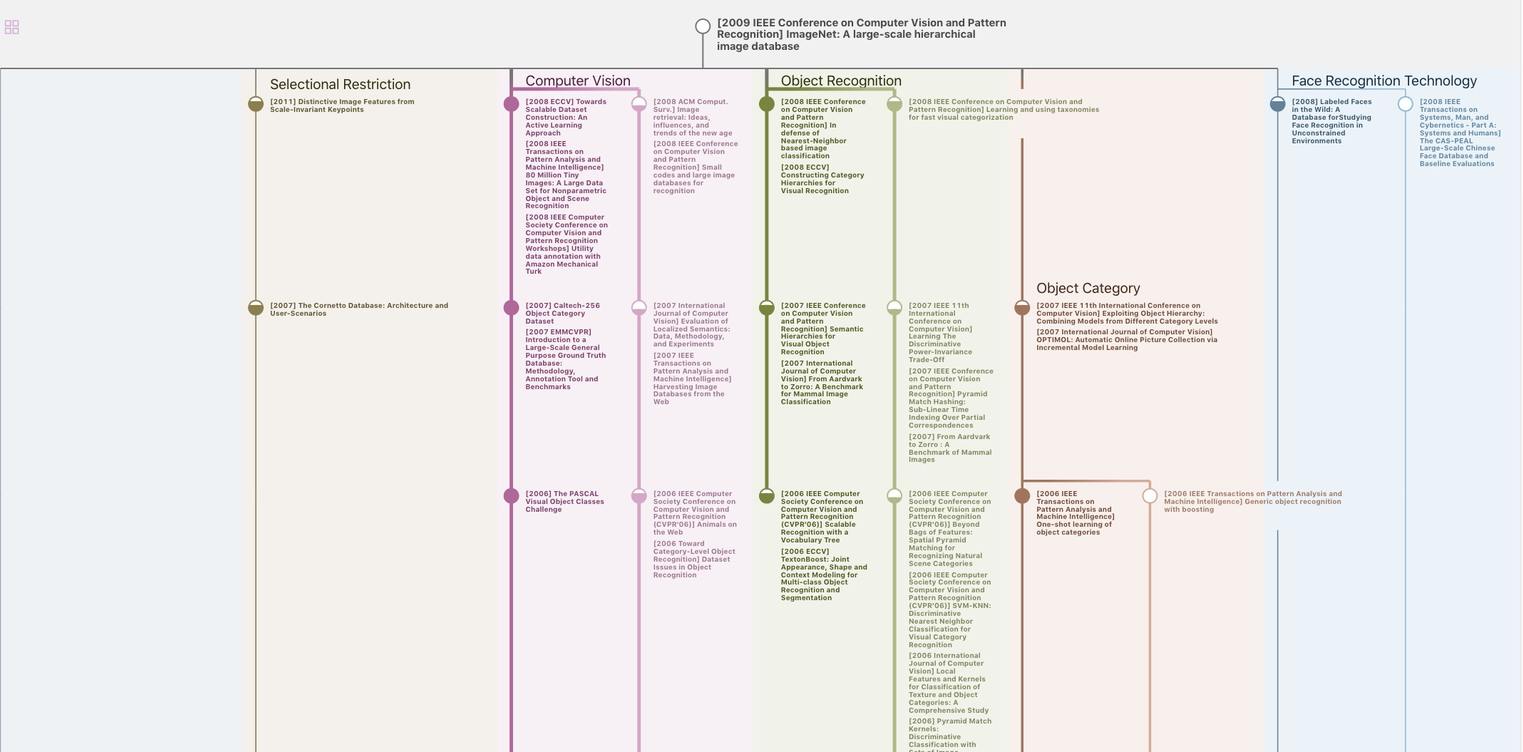
生成溯源树,研究论文发展脉络
Chat Paper
正在生成论文摘要