Vector Quantization and Shifting: Exploiting Latent Properties to Optimize Neural Codecs
ICLR 2023(2023)
摘要
End-to-end image/video codecs are getting competitive compared to traditional compression techniques that have been developed through decades of manual engineering efforts. These trainable codecs have many advantages over traditional techniques such as easy adaptation on perceptual distortion metrics and high performance on specific domains thanks to their learning ability. However, state of the art neural codecs do not take advantage of vector quantization technique and existence of gradient of entropy in decoding device. In this research, we propose some theoretical insights about these two properties (quantization and entropy gradient), and show that this can improve the performances of many off-the-shelf codecs. First, we prove that non-uniform quantization map on neural codec's latent is not necessary. Thus, we improve the performance by using a predefined optimal uniform vector quantization map. Secondly, we theoretically show that gradient of entropy (available at decoder side) is correlated with the gradient of the reconstruction error (which is not available at decoder side). Thus, we use the former as a proxy in order to improve the compression performance. According to our results, we save between 2-4\% of rate for the same quality with this proposal, for various pre-trained methods.
更多查看译文
关键词
Neural Image Compression,Uniform Vector Quantization,Gradient of Entropy
AI 理解论文
溯源树
样例
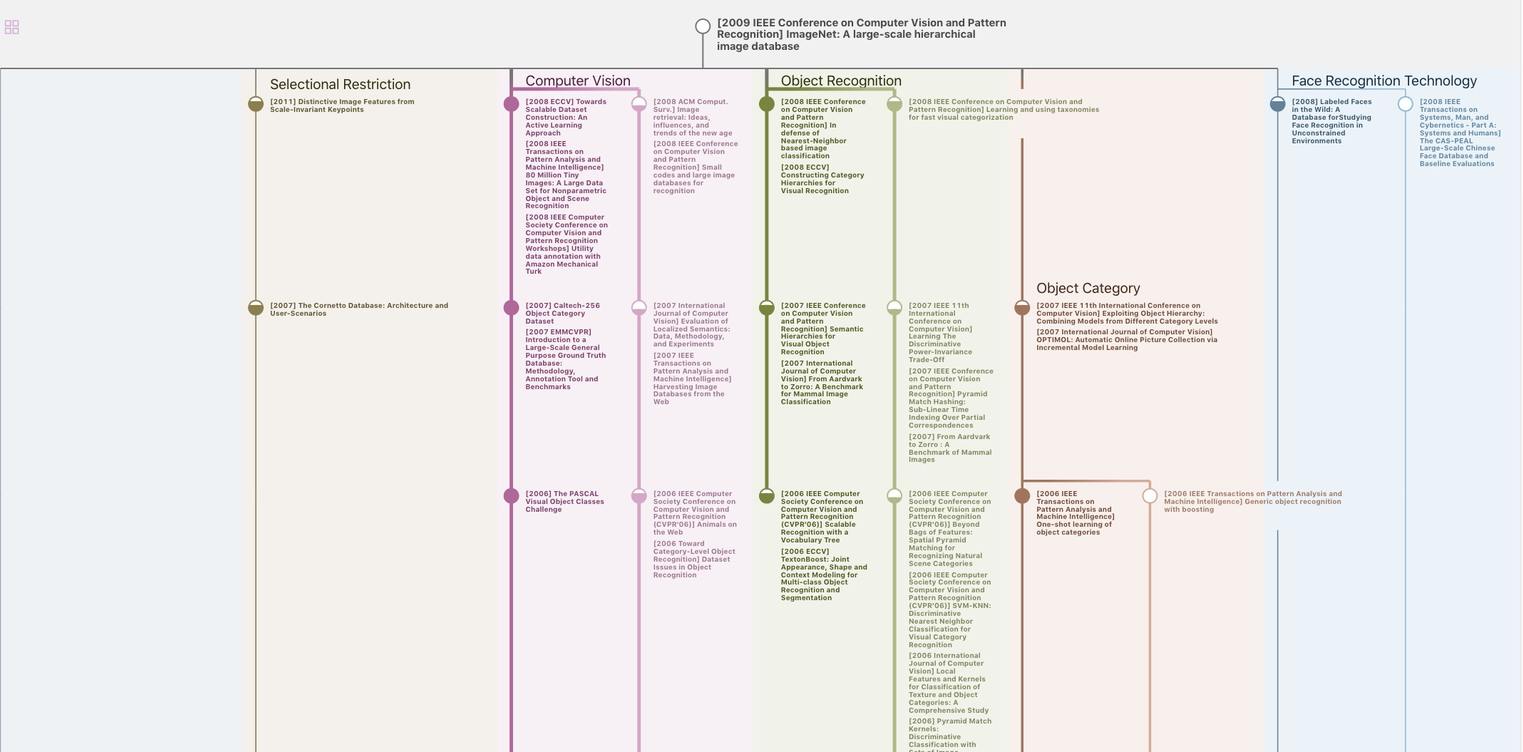
生成溯源树,研究论文发展脉络
Chat Paper
正在生成论文摘要