Multi-Behavior Dynamic Contrastive Learning for Recommendation
ICLR 2023(2023)
摘要
Dynamic behavior modeling has become an essential task in personalized recommender systems for learning the time-evolving user preference in online platforms. However, most next-item recommendation methods follow the single type behavior learning manner, which notably limits their user representation performance in reality, since the user-item relationships are often multi-typed in real-life applications (e.g., click, tag-as-favorite, review and purchase). To offer better recommendations, this work proposes Evolving Graph Contrastive Memory Network (EGCM) to model dynamic interaction heterogeneity for multi-behavior sequential recommendation. Specifically, we first develop a multi-behavior graph encoder to capture the short-term preference heterogeneity, and preserve the dedicated relation semantics for different types of user-item interactions. In addition, we design a dynamic cross-relational memory network, empowering EGCM to distill the long-term multi-behavior preference of users and the underlying evolving cross-type behavior dependencies over time. To enhance the user representation with multi-behavior commonality and diversity, we design a multi-behavior contrastive learning paradigm with heterogeneous short- and long-term interest modeling. Experiments on several real-world datasets show the superiority of our recommender system over various state-of-the-art baselines.
更多查看译文
关键词
Multi-Behavior Recommendation,Dynamic Contrastive Learning
AI 理解论文
溯源树
样例
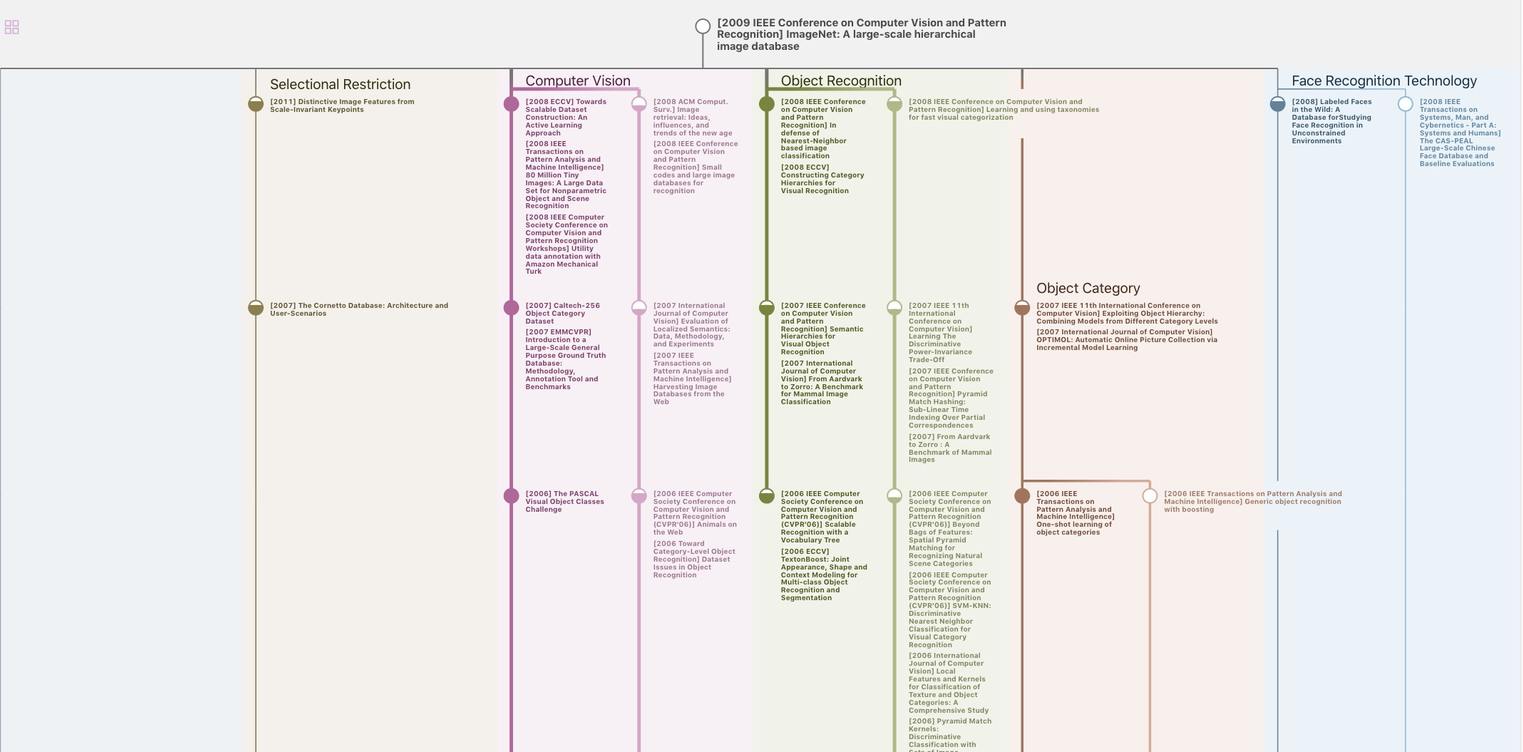
生成溯源树,研究论文发展脉络
Chat Paper
正在生成论文摘要