Graph Contrastive Learning with Model Perturbation
ICLR 2023(2023)
摘要
Graph contrastive learning (GCL) has achieved great success in pre-training graph neural networks (GNN) without ground-truth labels. The performance of GCL mainly rely on designing high quality contrastive views via data augmentation. However, finding desirable augmentations is difficult and requires cumbersome efforts due to the diverse modalities in graph data. In this work, we study model perturbation to perform efficient contrastive learning on graphs without using data augmentation. Instead of searching for the optimal combination among perturbing nodes, edges or attributes, we propose to conduct perturbation on the model architectures (i.e., GNNs). However, it is non-trivial to achieve effective perturbations on GNN models without performance dropping compared with its data augmentation counterparts. This is because data augmentation 1) makes complex perturbation in the graph space, so it is hard to mimic its effect in the model parameter space with a fixed noise distribution, and 2) has different disturbances even on the same nodes between two views owning to the randomness. Motivated by this, we propose a novel model perturbation framework -- \textsc{PerturbGCL} to pre-train GNN encoders. We focus on perturbing two key operations in a GNN, including message propagation and transformation. Specifically, we propose \emph{weightPrune} to create a dynamic perturbed model to contrast with the target one by pruning its transformation weights according to their magnitudes. Contrasting the two models will lead to adaptive mining of the perturbation distribution from the data. Furthermore, we present \emph{randMP} to disturb the steps of message propagation in two contrastive models. By randomly choosing the propagation steps during training, it helps to increase local variances of nodes between the contrastive views. Despite the simplicity, coupling the two strategies together enable us to perform effective contrastive learning on graphs with model perturbation. We conduct extensive experiments on 15 benchmarks. The results demonstrate the superiority of \textsc{PerturbGCL}: it can achieve competitive results against strong baselines across both node-level and graph-level tasks, while requiring shorter computation time. The code is available at \url{https://anonymous.4open.science/r/PerturbGCL-F17D}.
更多查看译文
关键词
Graph Contrastive Learning,Model Perturbation,Graph Augmentation
AI 理解论文
溯源树
样例
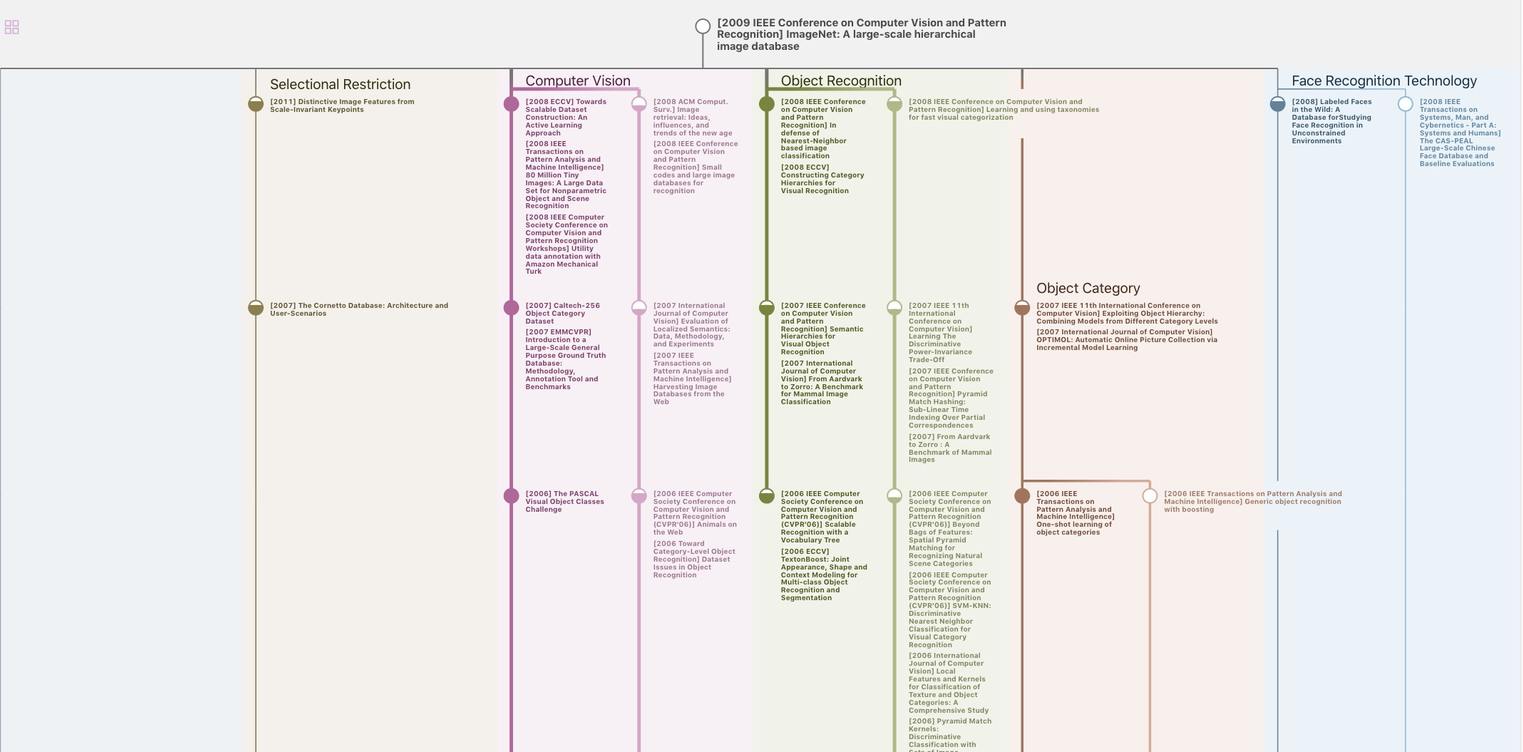
生成溯源树,研究论文发展脉络
Chat Paper
正在生成论文摘要