Fourier PINNs: From Strong Boundary Conditions to Adaptive Fourier Bases
ICLR 2023(2023)
摘要
Interest in Physics-Informed Neural Networks (PINNs) is rising as a mesh-free alternative to traditional numerical solvers for partial differential equations (PDEs). While successful, PINNs often struggle to learn high-frequency and multi-scale target solutions—which, according to prior analysis, might arise from competition during optimization between the weakly enforced boundary loss and residual loss terms. By creatively modifying the neural network architecture, some simple boundary conditions (BCs) can be satisfied exactly without jointly optimizing an additional loss term, thus avoiding the aforementioned competition altogether. Motivated by this analysis, we first study a strong BC version of PINNs for Dirichlet BCs and observe a consistent improvement compared to the standard PINNs. We conducted a Fourier analysis and found that strong BC PINNs can better learn the amplitudes of high-frequency components of the target solutions. While BC PINNs provide a promising improvement, constructing these unique architectures is an intricate process made difficult (if not impossible) by certain BCs and domain geometries. Enlightened by our analysis, we propose Fourier PINNs—a simple, general, yet powerful method that augments PINNs with pre-specified, dense Fourier bases. Our proposed architecture likewise better learns high-frequency components but places no restrictions on the particular BCs. We developed an adaptive learning and basis selection algorithm based on alternating NN basis optimization, Fourier and NN basis coefficient estimations, and coefficient truncation. This schema can flexibly identify the significant frequencies while weakening the nominal to better capture the target solution's power spectrum. We show the advantage of our approach in learning high-frequency and multi-scale solutions in a set of systematic experiments.
更多查看译文
关键词
Physics Informed Machine Learning,Fourier Analysis,Scientific Machine Learning,Partial Differential Equations
AI 理解论文
溯源树
样例
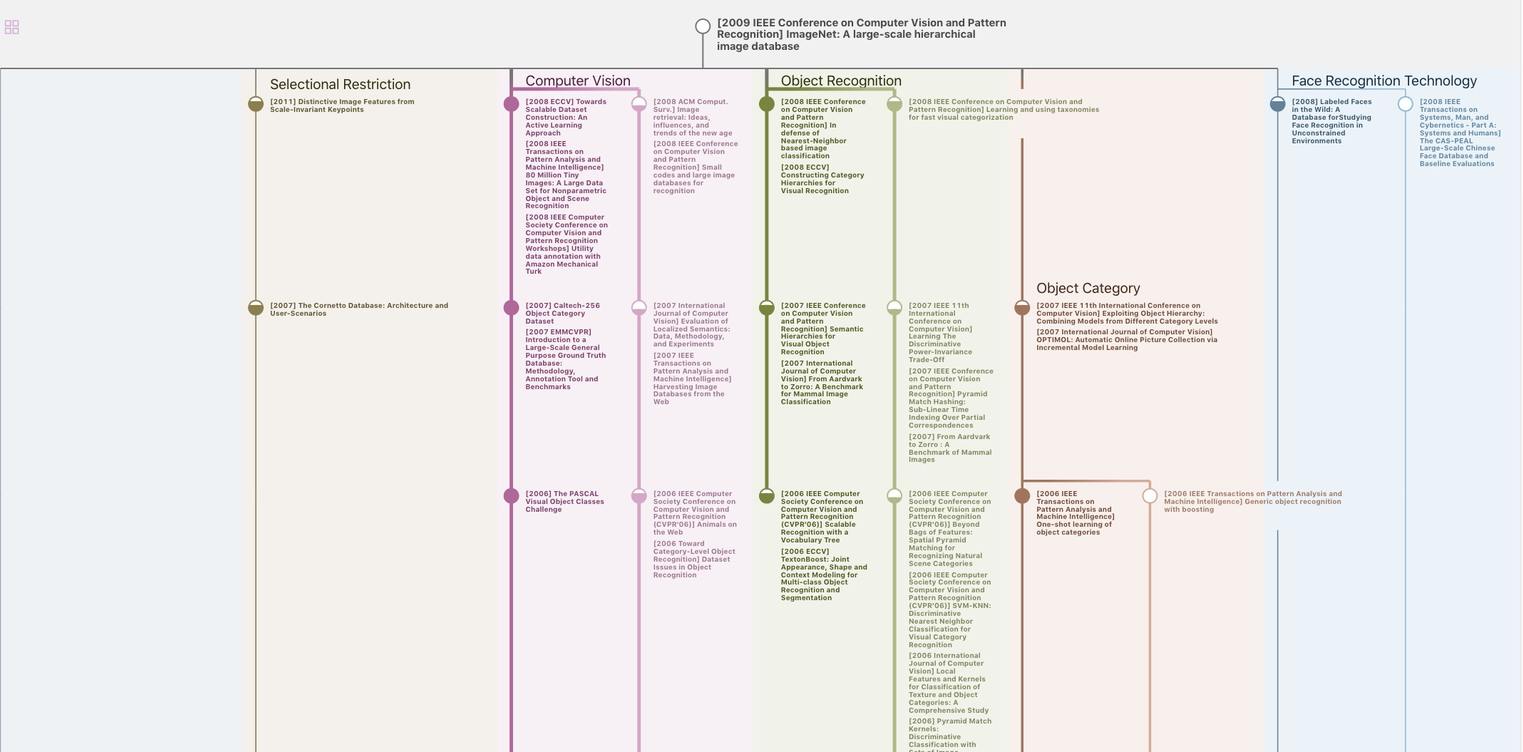
生成溯源树,研究论文发展脉络
Chat Paper
正在生成论文摘要