Why Did This Model Forecast This Future? Information-Theoretic Temporal Saliency for Counterfactual Explanations of Probabilistic Forecasts
ICLR 2023(2023)
摘要
Probabilistic forecasting of multivariate time series is significant to several research domains where multiple futures exist for a single observed sequence. Identifying the observations on which a well-performing model bases its forecasts can enable domain experts to form data-driven hypotheses about the causal relationships between features. Consequently, we begin by revisiting the question: what constitutes a causal explanation? One hurdle in the landscape of explainable artificial intelligence is that what constitutes an explanation is not well-grounded. We build upon Miller's framework of explanations derived from research in multiple social science disciplines, and establish a conceptual link between counterfactual reasoning and saliency-based explanation techniques. However, the complication is a lack of a consistent and principled notion of saliency. Also, commonly derived saliency maps may be inconsistent with the data generation process and the underlying model. We therefore leverage a unifying definition of information-theoretic saliency grounded in preattentive human visual cognition and extend it to forecasting settings. In contrast to existing methods that require either explicit training of the saliency mechanism or access to the internal parameters of the underlying model, we obtain a closed-form solution for the resulting saliency map for commonly used density functions in probabilistic forecasting. To empirically evaluate our explainability framework in a principled manner, we construct a synthetic dataset of conversation dynamics and demonstrate that our method recovers the true salient timesteps for a forecast given a well-performing underlying model.
更多查看译文
关键词
probabilistic forecasting,saliency,explainability
AI 理解论文
溯源树
样例
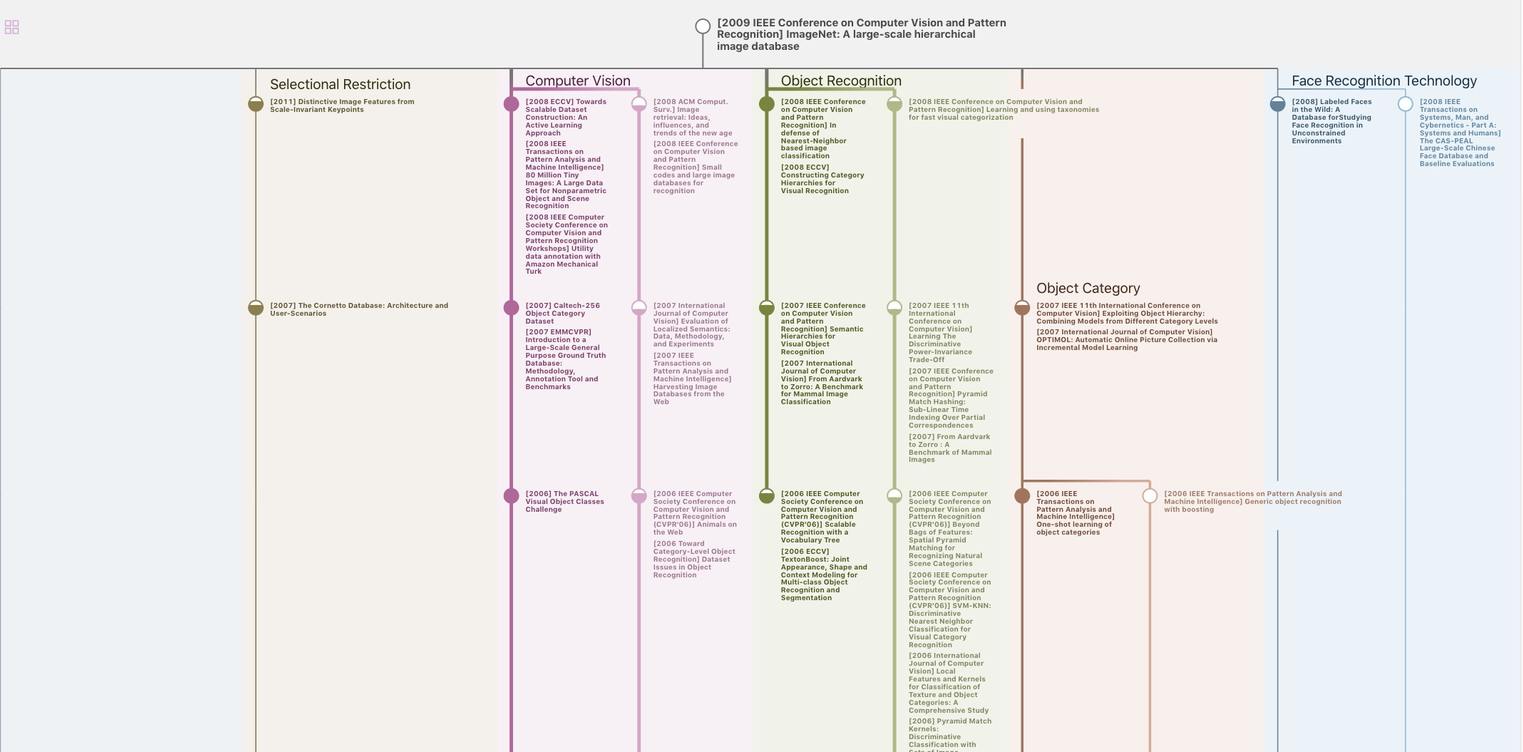
生成溯源树,研究论文发展脉络
Chat Paper
正在生成论文摘要