Training Equilibria in Reinforcement Learning
ICLR 2023(2023)
摘要
In partially observable environments, reinforcement learning algorithms such as policy gradient and Q-learning may have multiple equilibria---policies that are stable under further training---and can converge to policies that are strictly suboptimal.
Prior work blames insufficient exploration, but suboptimal equilibria can arise despite full exploration and other favorable circumstances like a flexible policy parametrization.
We show theoretically that the core problem is that in partially observed environments, an agent's past actions induce a distribution on hidden states.
Equipping the policy with memory helps it model the hidden state and leads to convergence to a higher reward equilibrium, \emph{even when there exists a memoryless optimal policy}.
Experiments show that
policies with insufficient memory tend to learn to use the environment as auxiliary memory,and parameter noise helps policies escape suboptimal equilibria.
更多查看译文
关键词
theory,reinforcement learning,learning dynamics,partial observability,MDP,POMDP,markov decision processes
AI 理解论文
溯源树
样例
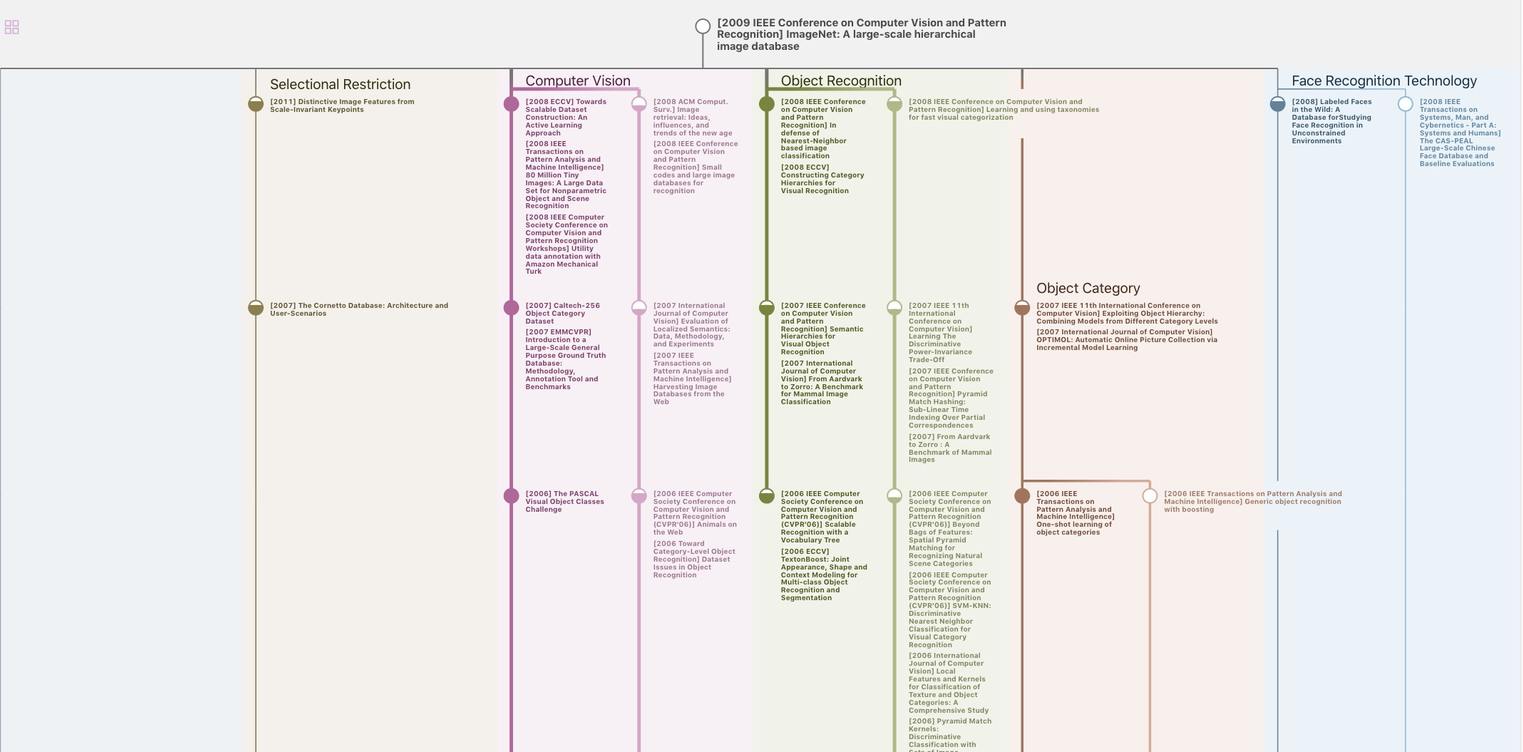
生成溯源树,研究论文发展脉络
Chat Paper
正在生成论文摘要