Speed Up Iterative Non-Autoregressive Transformers by Distilling Multiple Steps
ICLR 2023(2023)
摘要
The computational benefits of iterative non-autoregressive transformers decrease as the number of decoding steps increases. As a remedy, we introduce Distill Multiple Steps (DiMS), a simple yet effective distillation technique to decrease the number of required steps to reach a certain translation quality. The distilled model enjoys the computational benefits of early iterations while preserving the enhancements from several iterative steps. DiMS relies on two models namely student and teacher. The student is optimized to predict the output of the teacher after multiple decoding steps while the teacher follows the student via a slow-moving average. The moving average keeps the teacher's knowledge updated and enhances the quality of the labels provided by the teacher. During inference, the student is used for translation and no additional computation is added. We verify the effectiveness of DiMS on various models obtaining 7 and 12.9 BLEU points improvements on distilled and raw versions of WMT'14 De-En, respectively.
更多查看译文
关键词
non-autoregressive machine translation,knowledge distillation
AI 理解论文
溯源树
样例
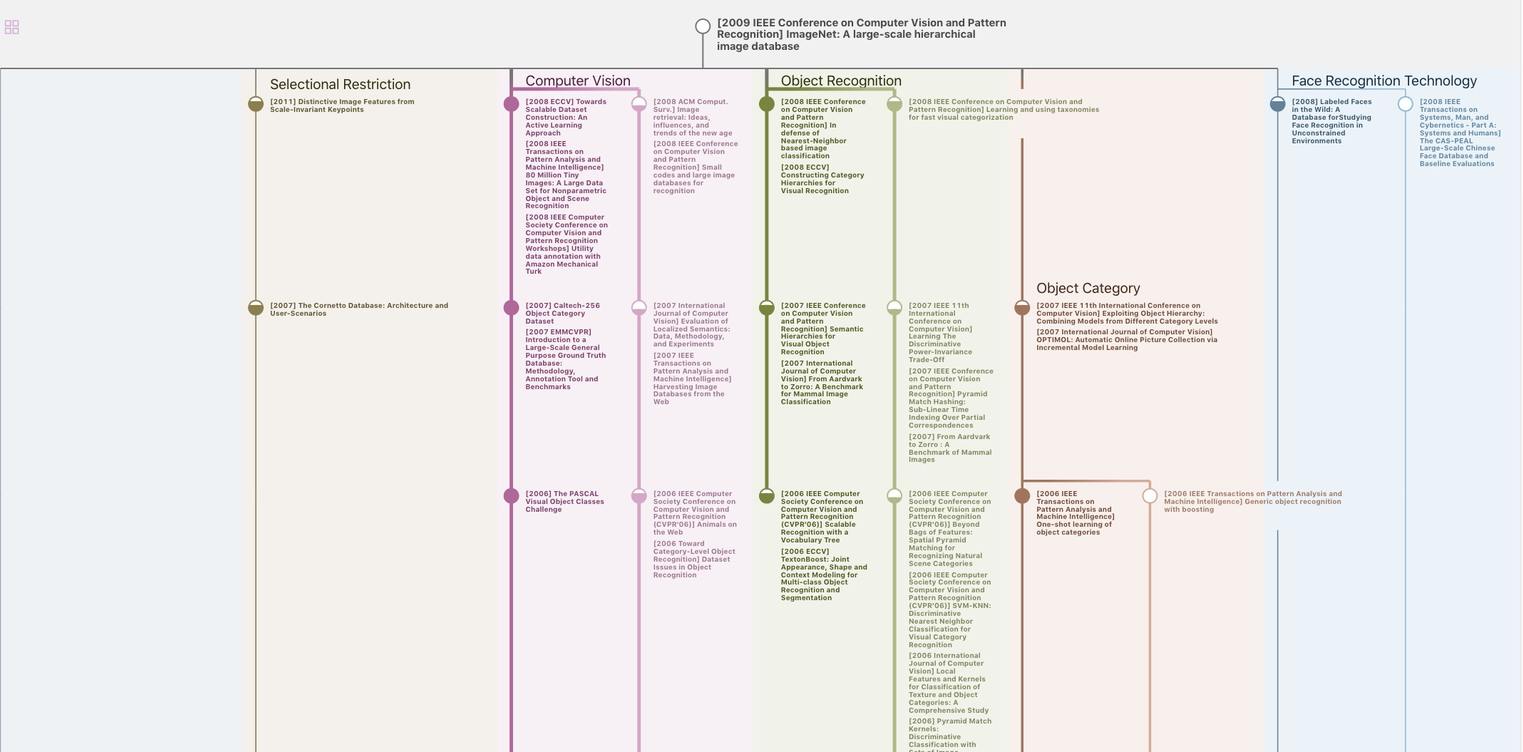
生成溯源树,研究论文发展脉络
Chat Paper
正在生成论文摘要