Robustness for Free: Adversarially Robust Anomaly Detection Through Diffusion Model
ICLR 2023(2023)
摘要
Deep learning-based anomaly detection models have achieved remarkably high accuracy on commonly used benchmark datasets. However, the robustness of those models may not be satisfactory due to the existence of adversarial examples, which pose significant threats to the practical deployment of deep anomaly detectors. To tackle this issue, we propose an adversarially robust anomaly detector based on the diffusion model. There are two things that make diffusion models a perfect match for our task: 1) the diffusion model itself is a reconstruction-based modeling method whose reconstruction error can serve as a natural indicator of the anomaly score; 2) previous studies have shown that diffusion models can help purify the data for better adversarial robustness. In this work, we highlight that our diffusion model based method gains the adversarial robustness for free: the diffusion model will act both as an anomaly detector and an adversarial defender, thus no extra adversarial training or data purification is needed as in standard robust image classification tasks. We also extend our proposed method for certified robustness to $l_2$ norm bounded perturbations. Through extensive experiments, we show that our proposed method exhibits outstanding (certified) adversarial robustness while also maintaining equally strong anomaly detection performance on par with the state-of-the-art anomaly detectors on benchmark datasets.
更多查看译文
AI 理解论文
溯源树
样例
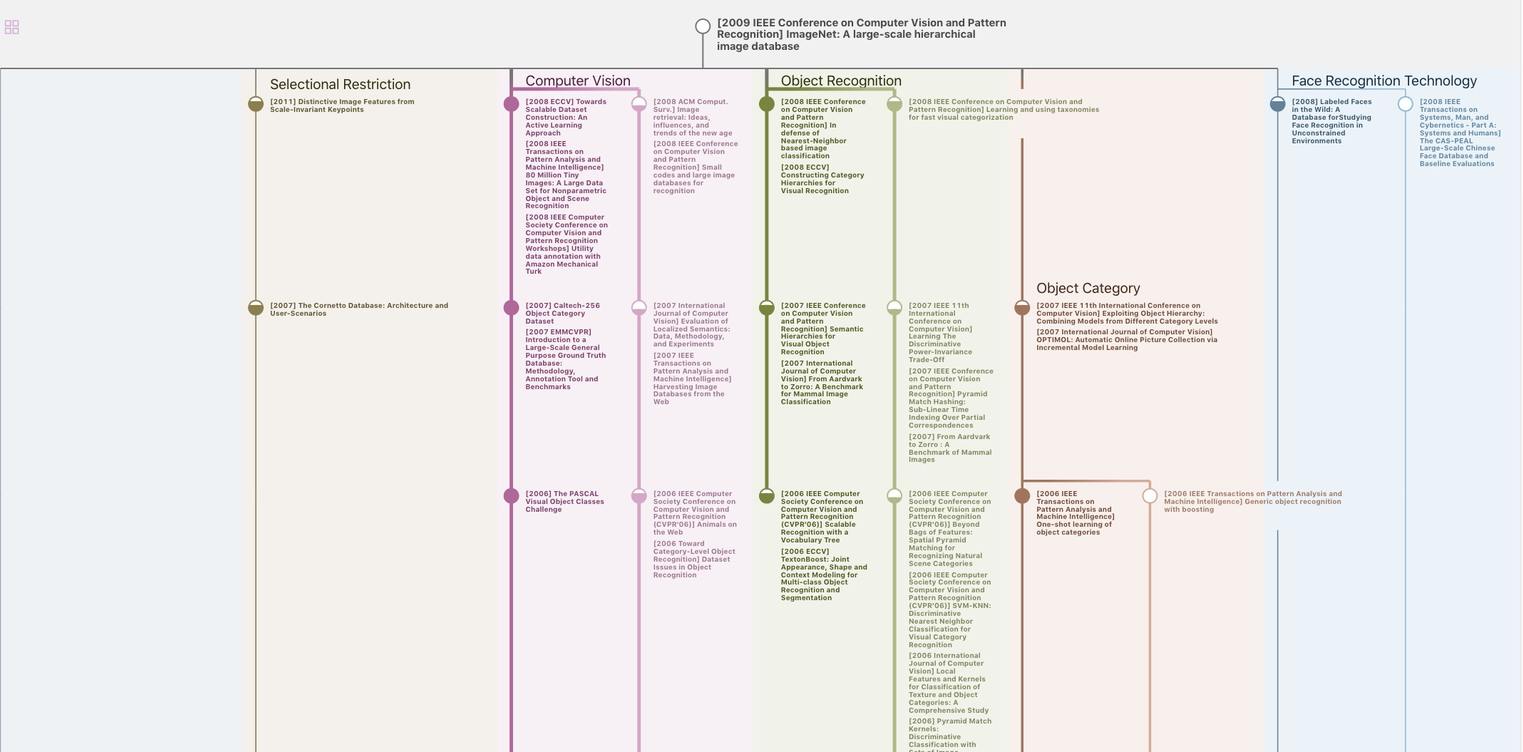
生成溯源树,研究论文发展脉络
Chat Paper
正在生成论文摘要