Connecting representation and generation via masked vision-language transformer
ICLR 2023(2023)
摘要
Recently, there has been great progress in the self-supervised pre-training of multimodal representation models that understand image and language jointly. One particularly popular application of such models is text-to-image generation, which is typically obtained via a two-stage process: in the first stage, a representation model is trained via self-supervised objectives; then in the second stage, a conditional generative decoder is trained on top of the representation to generate natural images. In this work, we aim at bringing representation learning and conditional generation together by unifying the two stages into a single model and training objective. We present UPGen, a unified pre-trained model for both representation learning and generation. UPGen is trained with a simple masked token prediction objective on a flexible mixture of image and language data. We use a pre-trained VQGAN image tokenizer to convert images into discrete tokens, then train a masked token prediction model on both paired image-text datasets and unpaired language datasets, using randomly sampled mask ratios. We show that this masked token prediction model can be directly used to generate images and language by iteratively re-masking and predicting the masked tokens. We demonstrate empirically that UPGen serves as both a good representation learning model and a generative model for both image and language.
更多查看译文
关键词
Representation Learning,Pre-training,Generative Model,Conditional Generation
AI 理解论文
溯源树
样例
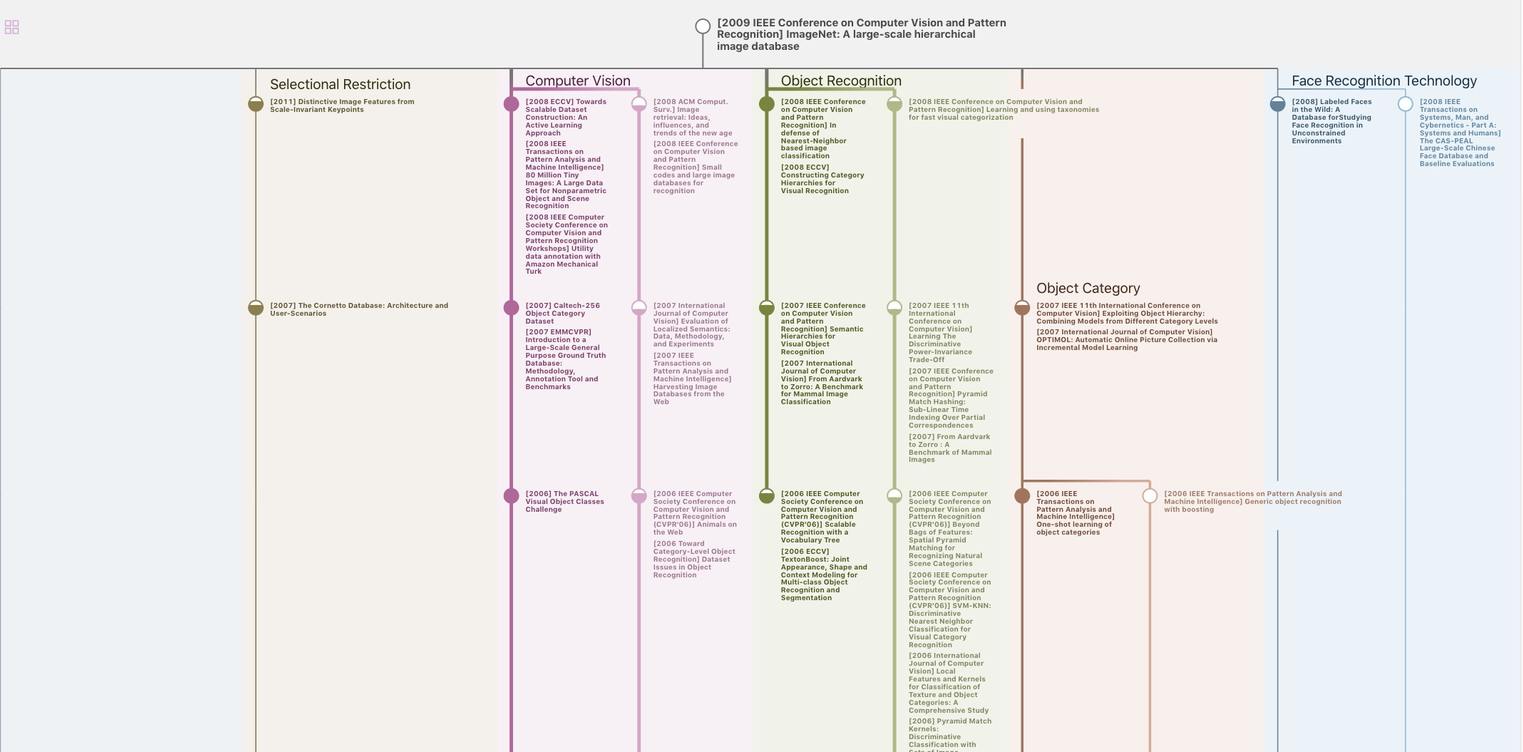
生成溯源树,研究论文发展脉络
Chat Paper
正在生成论文摘要