Elicitation Inference Optimization for Multi-Principal-Agent Alignment
ICLR 2023(2023)
摘要
In multi-principal-agent alignment scenarios spanning governance, markets, diplomacy, and AI, it is infeasible to elicit every principal's view on all perspectives relevant to agent decisions. Elicitation inference optimization (EIO) aims to minimize the $n$ elicitations needed to approximate $N$ principal's views across $K$ perspectives. In this work, we demonstrate an EIO approach where data efficiency ($NK/n$) increases with scale. We introduce STUMP: an elicitation inference model which integrates an LLM with a latent factor model to enable learning transfer across samples, contexts, and languages. Then, we characterize STUMP's performance on a set of elicitation primitives from which scalable elicitation (sampling) protocols can be constructed. Building from these results, we design and demonstrate two scalable elicitation protocols for STUMP where data efficiency grows boundlessly, scaling like $O(n)$ in the number of elicitations $n$. This makes it possible to obtain complex, high-dimensional preference signals spanning principal populations at any scale.
更多查看译文
关键词
alignment,large language models,LLMs,NLP,transfer learning,human-centered AI,LLMs,preference modeling
AI 理解论文
溯源树
样例
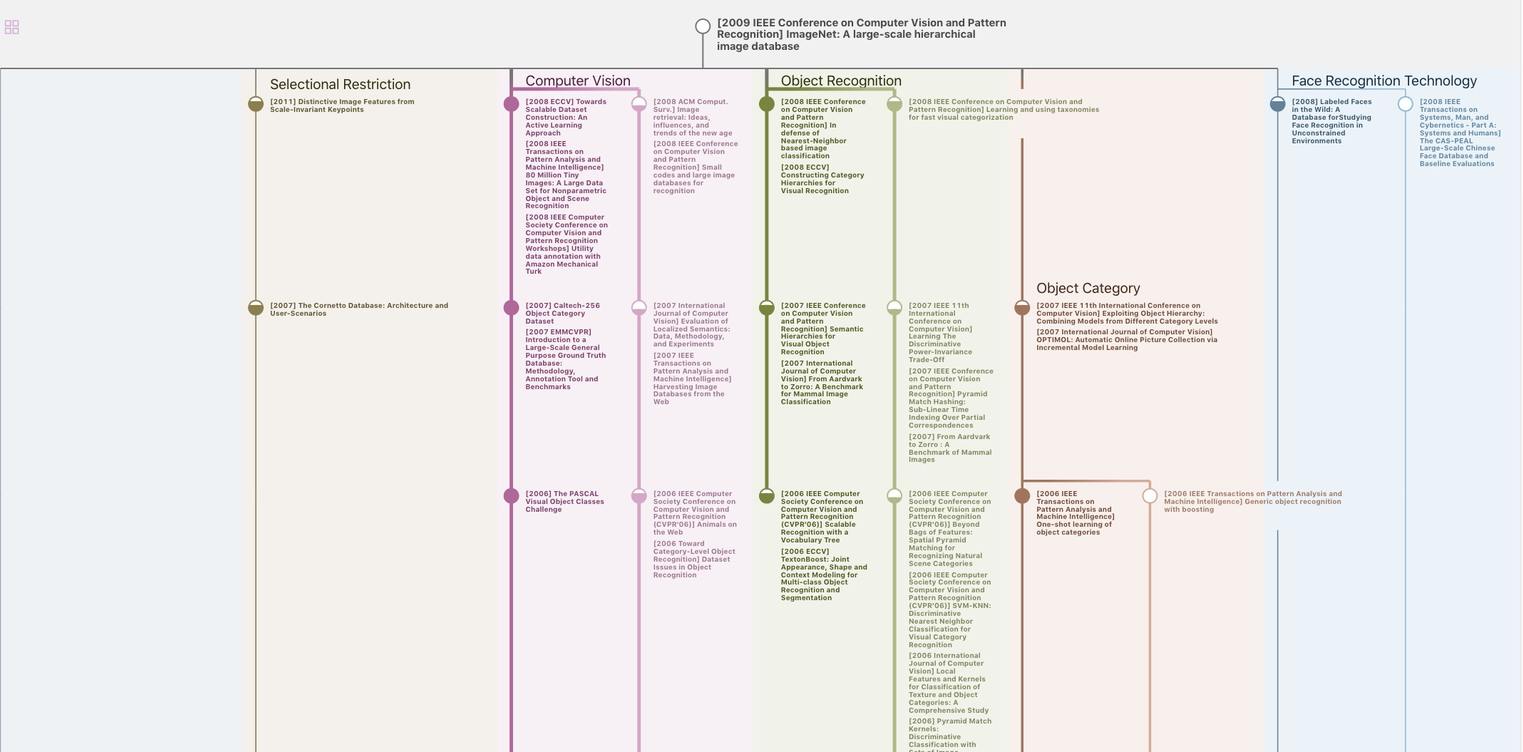
生成溯源树,研究论文发展脉络
Chat Paper
正在生成论文摘要