Functional Risk Minimization
ICLR 2023(2023)
摘要
In this work, we break the classic assumption of data coming from a single function $f_{\theta^*}(x)$ followed by some noise in output space $p(y|f_{\theta^*}(x))$. Instead, we model each data point $(x_i,y_i)$ as coming from its own function $f_{\theta_i}$. We show that this model subsumes Empirical Risk Minimization for many common loss functions, and provides an avenue for more realistic noise processes. We derive Functional Risk Minimization~(FRM), a general framework for scalable training objectives which results in better performance in small experiments in regression and reinforcement learning. We also show that FRM can be seen as finding the simplest model that memorizes the training data, providing an avenue towards understanding generalization in the over-parameterized regime.
更多查看译文
关键词
learning framework,theory,meta-learning,supervised learning
AI 理解论文
溯源树
样例
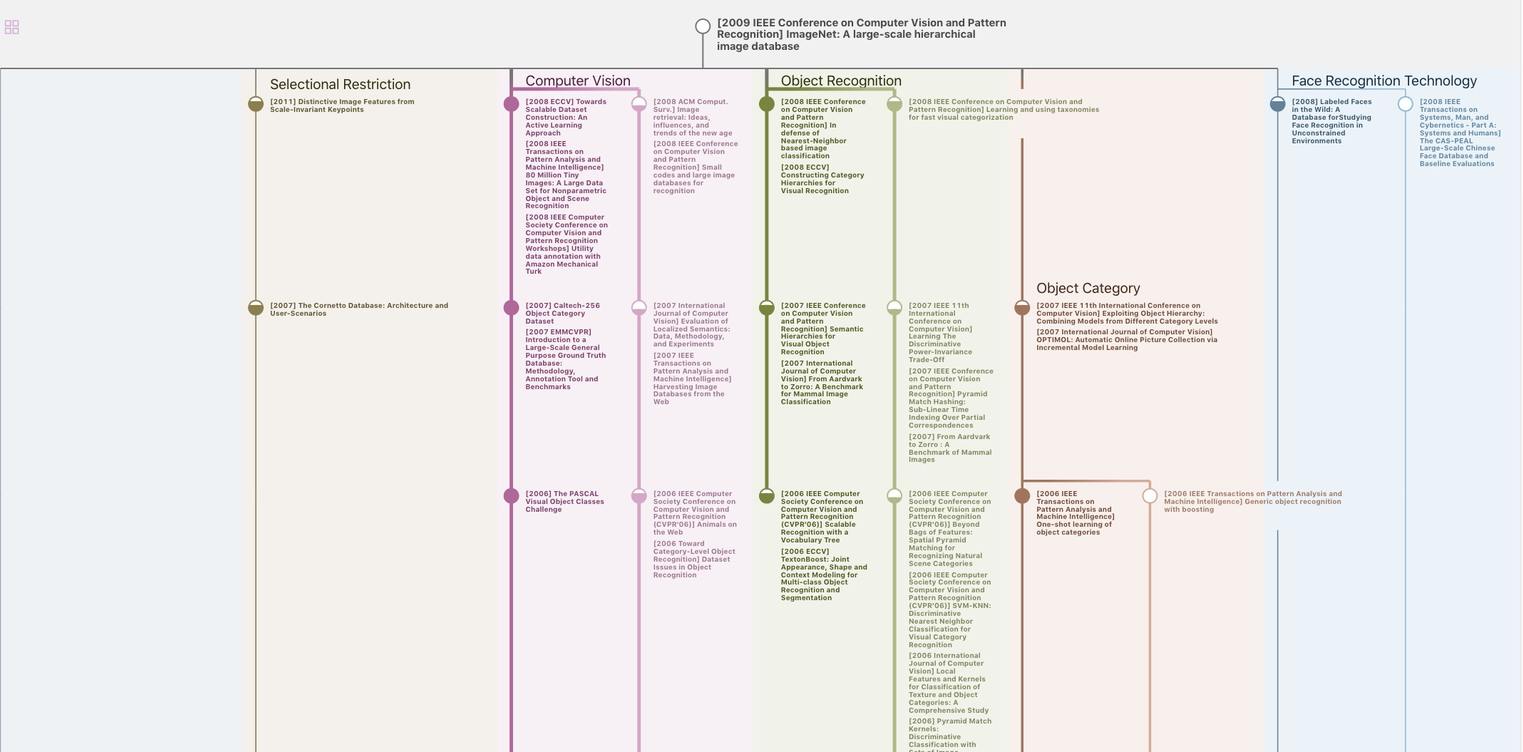
生成溯源树,研究论文发展脉络
Chat Paper
正在生成论文摘要