SE-shapelets: Semi-supervised Clustering of Time Series Using Representative Shapelets
EXPERT SYSTEMS WITH APPLICATIONS(2024)
摘要
Shapelets that discriminate time series using local features (subsequences) are promising for time series clustering. Existing time series clustering methods may fail to capture representative shapelets because they discover shapelets from a large pool of uninformative subsequences, and thus result in low clustering accuracy. This paper proposes a Semi-supervised Clustering of Time Series Using Representative Shapelets (SE-Shapelets) method, which utilizes a small number of labeled and propagated pseudo-labeled time series to help discover representative shapelets, thereby improving the clustering accuracy. In SE-Shapelets, we propose two techniques to discover representative shapelets for the effective clustering of time series. (1) A salient subsequence chain (SSC) that can extract salient subsequences (as candidate shapelets) of a labeled/pseudo-labeled time series, which helps remove massive uninformative subsequences from the pool. (2) A linear discriminant selection (LDS) algorithm to identify shapelets that can capture representative local features of time series in different classes, for convenient clustering. Experiments on UCR time series datasets demonstrate that SE-shapelets discovers representative shapelets and achieves higher clustering accuracy than counterpart semi-supervised time series clustering methods.
更多查看译文
关键词
Time series clustering,Shapelet discovery,Linear discriminant selection
AI 理解论文
溯源树
样例
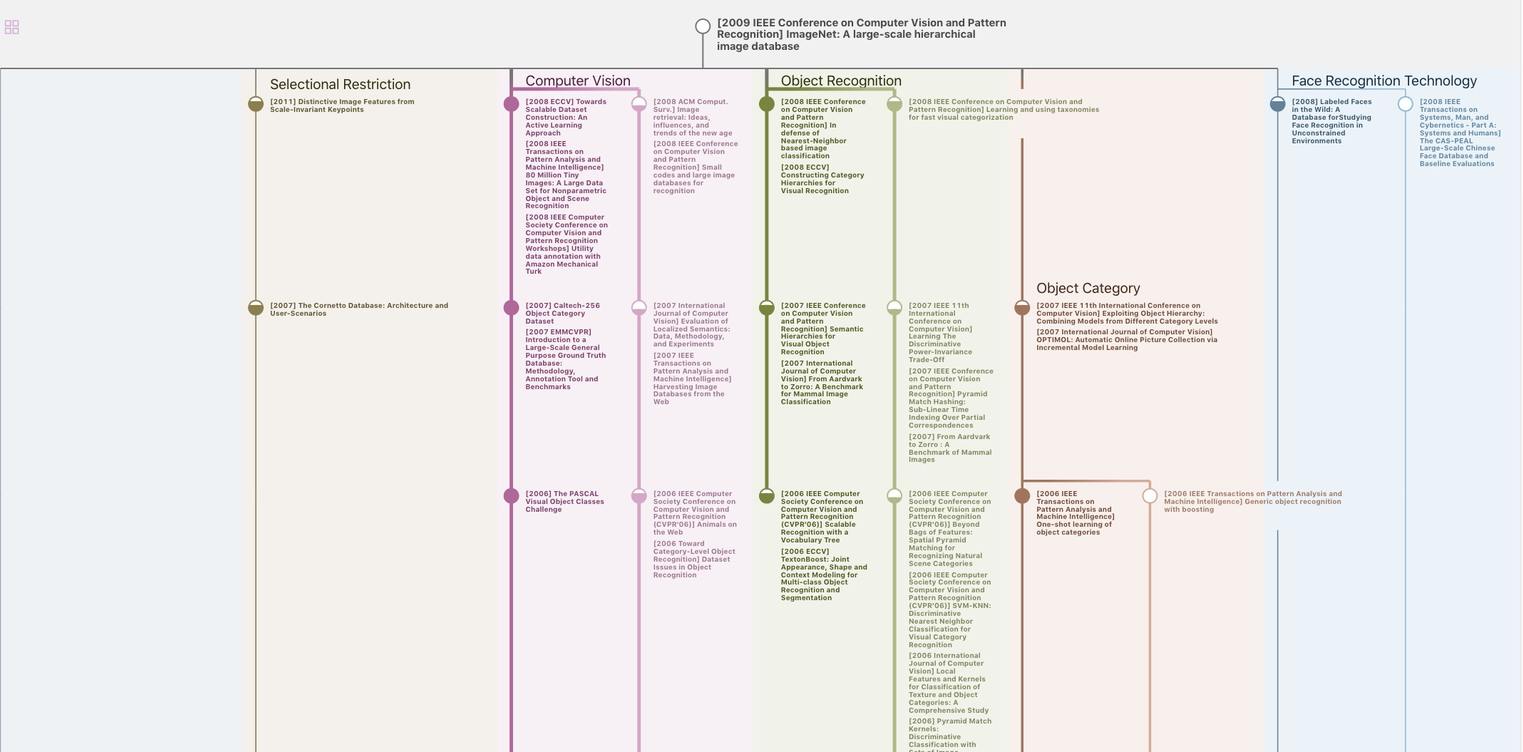
生成溯源树,研究论文发展脉络
Chat Paper
正在生成论文摘要