Deep Citywide Multisource Data Fusion-Based Air Quality Estimation
IEEE TRANSACTIONS ON CYBERNETICS(2024)
摘要
With the increasingly serious air pollution, people are paying more and more attention to air quality. However, air quality information is not available for all regions, as the number of air quality monitoring stations in a city is limited. Existing air quality estimation methods only consider the multisource data of partial regions and separately estimate the air qualities of all regions. In this article, we propose a deep citywide multisource data fusion-based air quality estimation (FAIRY) method. FAIRY considers the citywide multisource data and estimates the air qualities of all regions at a time. Specifically, FAIRY constructs images from the citywide multisource data (i.e., meteorology, traffic, factory air pollutant emission, point of interest, and air quality) and uses SegNet to learn the multiresolution features from these images. The features with the same resolution are fused by the self-attention mechanism to provide multisource feature interactions. To get a complete air quality image with high resolution, FAIRY refines low-resolution fused features by employing high-resolution fused features through residual connections. In addition, the Tobler's first law of geography is used to constrain the air qualities of adjacent regions, which can fully use the air quality relevance of nearby regions. Extensive experimental results demonstrate that FAIRY achieves the state-of-the-art performance on the Hangzhou city dataset, outperforming the best baseline by 15.7% on MAE.
更多查看译文
关键词
Air quality estimation,deep learning,multisource data fusion,multitask learning
AI 理解论文
溯源树
样例
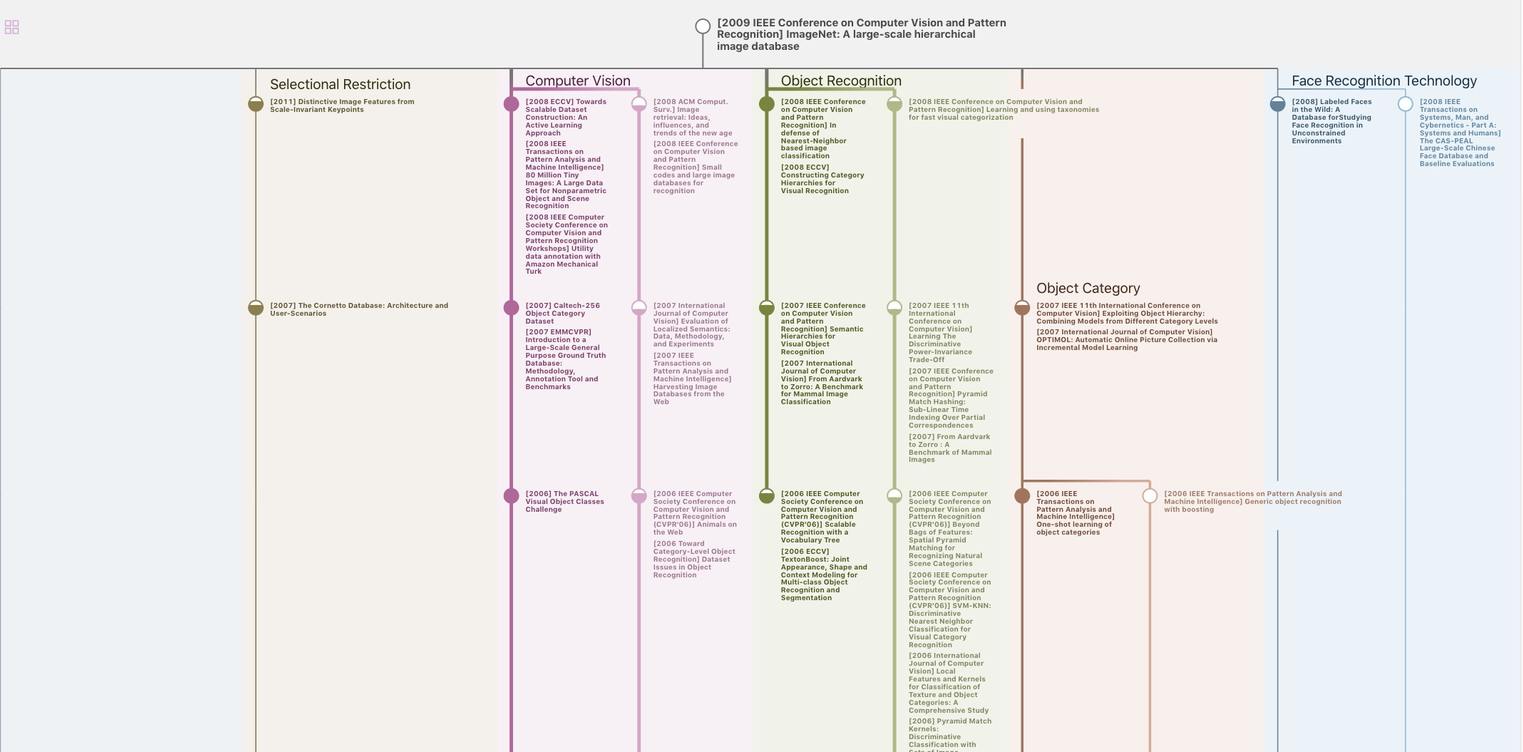
生成溯源树,研究论文发展脉络
Chat Paper
正在生成论文摘要