Accurate Registration of Cross-Modality Geometry via Consistent Clustering.
IEEE transactions on visualization and computer graphics(2023)
摘要
The registration of unitary-modality geometric data has been successfully explored over past decades. However, existing approaches typically struggle to handle cross-modality data due to the intrinsic difference between different models. To address this problem, in this paper, we formulate the cross-modality registration problem as a consistent clustering process. First, we study the structure similarity between different modalities based on an adaptive fuzzy shape clustering, from which a coarse alignment is successfully operated. Then, we optimize the result using fuzzy clustering consistently, in which the source and target models are formulated as clustering memberships and centroids, respectively. This optimization casts new insight into point set registration, and substantially improves the robustness against outliers. Additionally, we investigate the effect of fuzzier in fuzzy clustering on the cross-modality registration problem, from which we theoretically prove that the classical Iterative Closest Point (ICP) algorithm is a special case of our newly defined objective function. Comprehensive experiments and analysis are conducted on both synthetic and real-world cross-modality datasets. Qualitative and quantitative results demonstrate that our method outperforms state-of-the-art approaches with higher accuracy and robustness. Our code is publicly available at https://github.com/zikai1/CrossModReg.
更多查看译文
关键词
Cross-Modality Geometry,Point Cloud Registration,3D Reconstruction,Adaptive Fuzzy Clustering,CAD
AI 理解论文
溯源树
样例
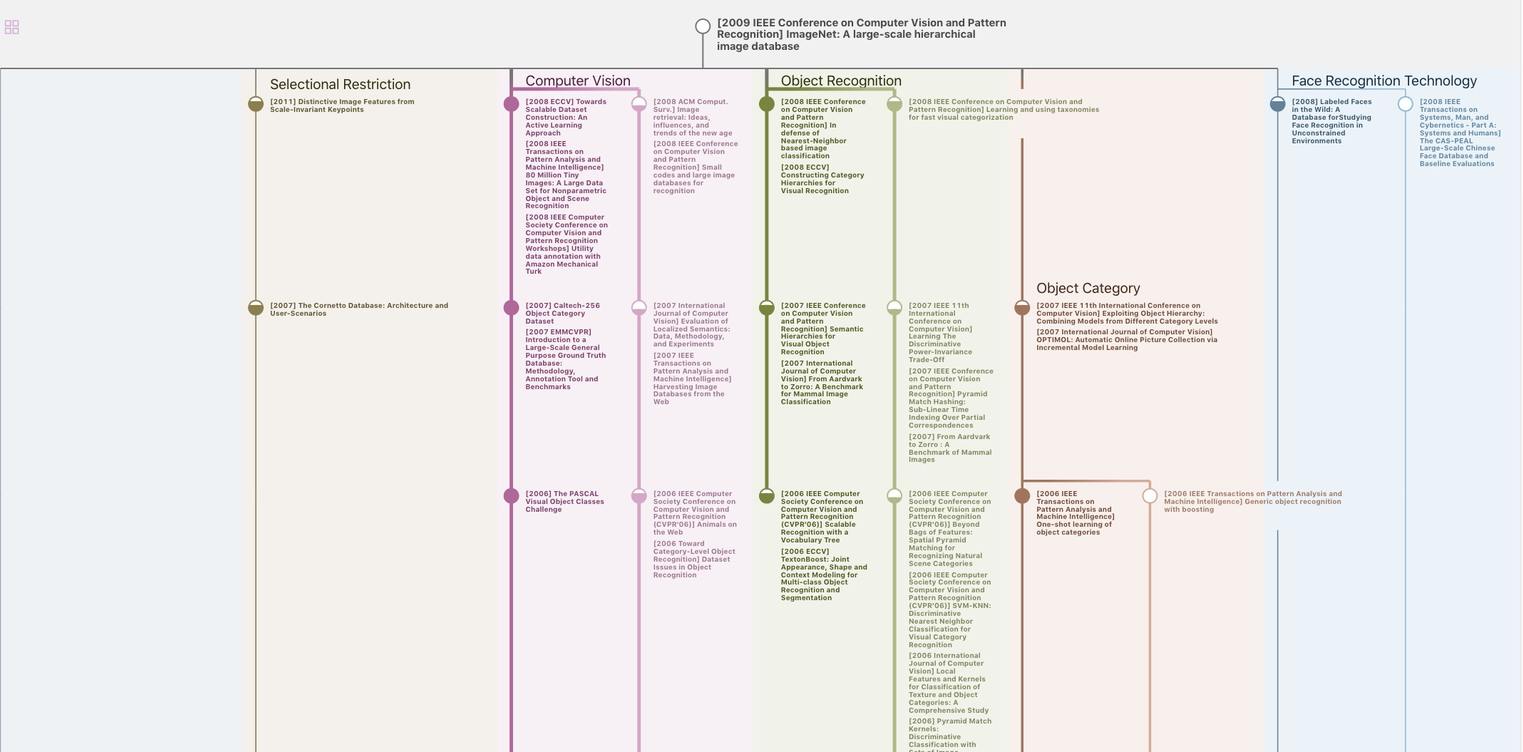
生成溯源树,研究论文发展脉络
Chat Paper
正在生成论文摘要