Inverse Optimal Adaptive Neural Control for State-Constrained Nonlinear Systems.
IEEE transactions on neural networks and learning systems(2023)
摘要
Optimizing a performance objective during control operation while also ensuring constraint satisfactions at all times is important in practical applications. Existing works on solving this problem usually require a complicated and time-consuming learning procedure by employing neural networks, and the results are only applicable for simple or time-invariant constraints. In this work, these restrictions are removed by a newly proposed adaptive neural inverse approach. In our approach, a new universal barrier function, which is able to handle various dynamic constraints in a unified manner, is proposed to transform the constrained system into an equivalent one with no constraint. Based on this transformation, a switched-type auxiliary controller and a modified criterion for inverse optimal stabilization are proposed to design an adaptive neural inverse optimal controller. It is proven that optimal performance is achieved with a computationally attractive learning mechanism, and all the constraints are never violated. Besides, improved transient performance is obtained in the sense that the bound of the tracking error could be explicitly designed by users. An illustrative example verifies the proposed methods.
更多查看译文
关键词
Optimal control,Nonlinear systems,Transforms,Neural networks,Lyapunov methods,Learning systems,Costs,Adaptive control,inverse optimal control,neural network,state constraint,strict-feedback nonlinear system
AI 理解论文
溯源树
样例
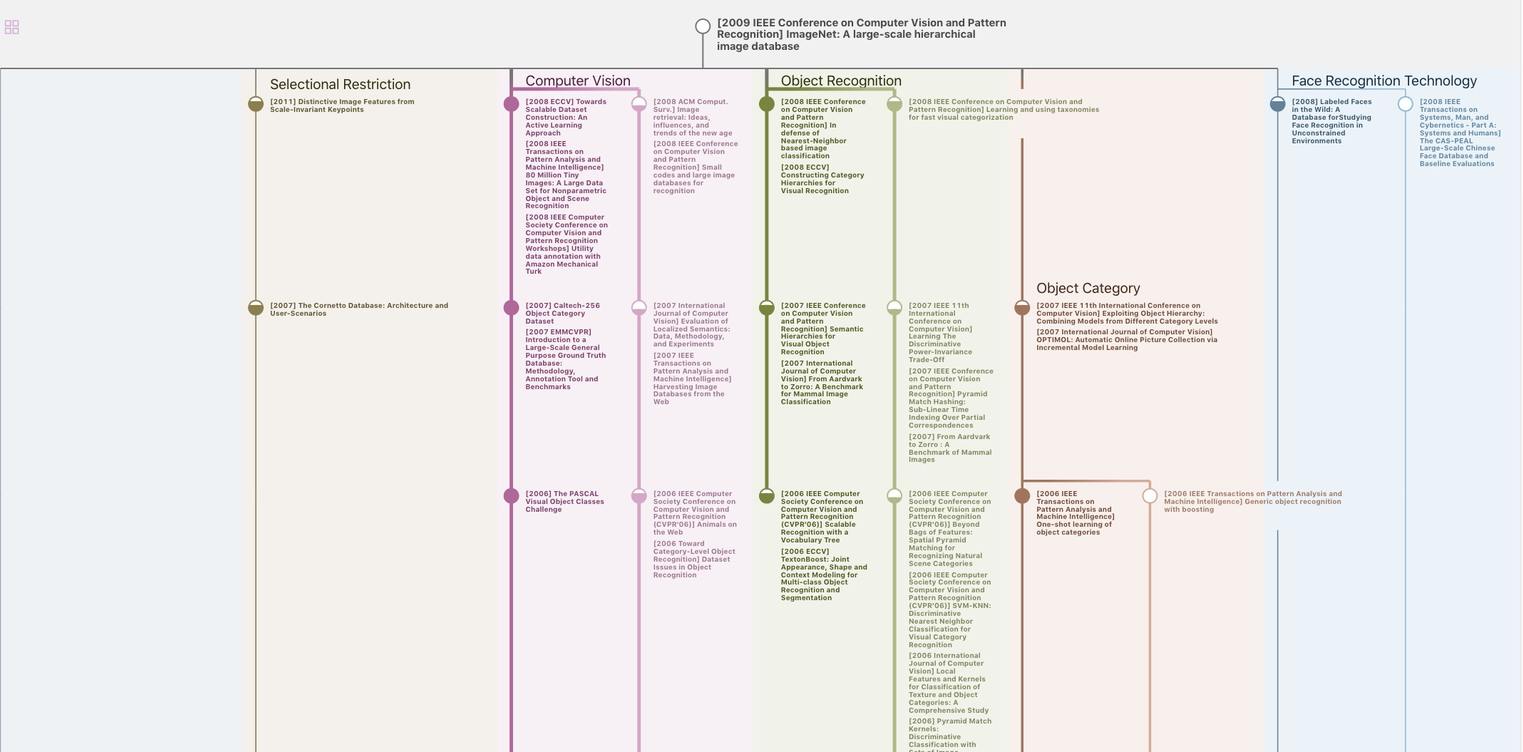
生成溯源树,研究论文发展脉络
Chat Paper
正在生成论文摘要