Instruction Tuning with GPT-4.
arXiv (Cornell University)(2023)
摘要
Prior work has shown that finetuning large language models (LLMs) using machine-generated instruction-following data enables such models to achieve remarkable zero-shot capabilities on new tasks, and no human-written instructions are needed. In this paper, we present the first attempt to use GPT-4 to generate instruction-following data for LLM finetuning. Our early experiments on instruction-tuned LLaMA models show that the 52K English and Chinese instruction-following data generated by GPT-4 leads to superior zero-shot performance on new tasks to the instruction-following data generated by previous state-of-the-art models. We also collect feedback and comparison data from GPT-4 to enable a comprehensive evaluation and reward model training. We make our data generated using GPT-4 as well as our codebase publicly available.
更多查看译文
关键词
tuning,instruction
AI 理解论文
溯源树
样例
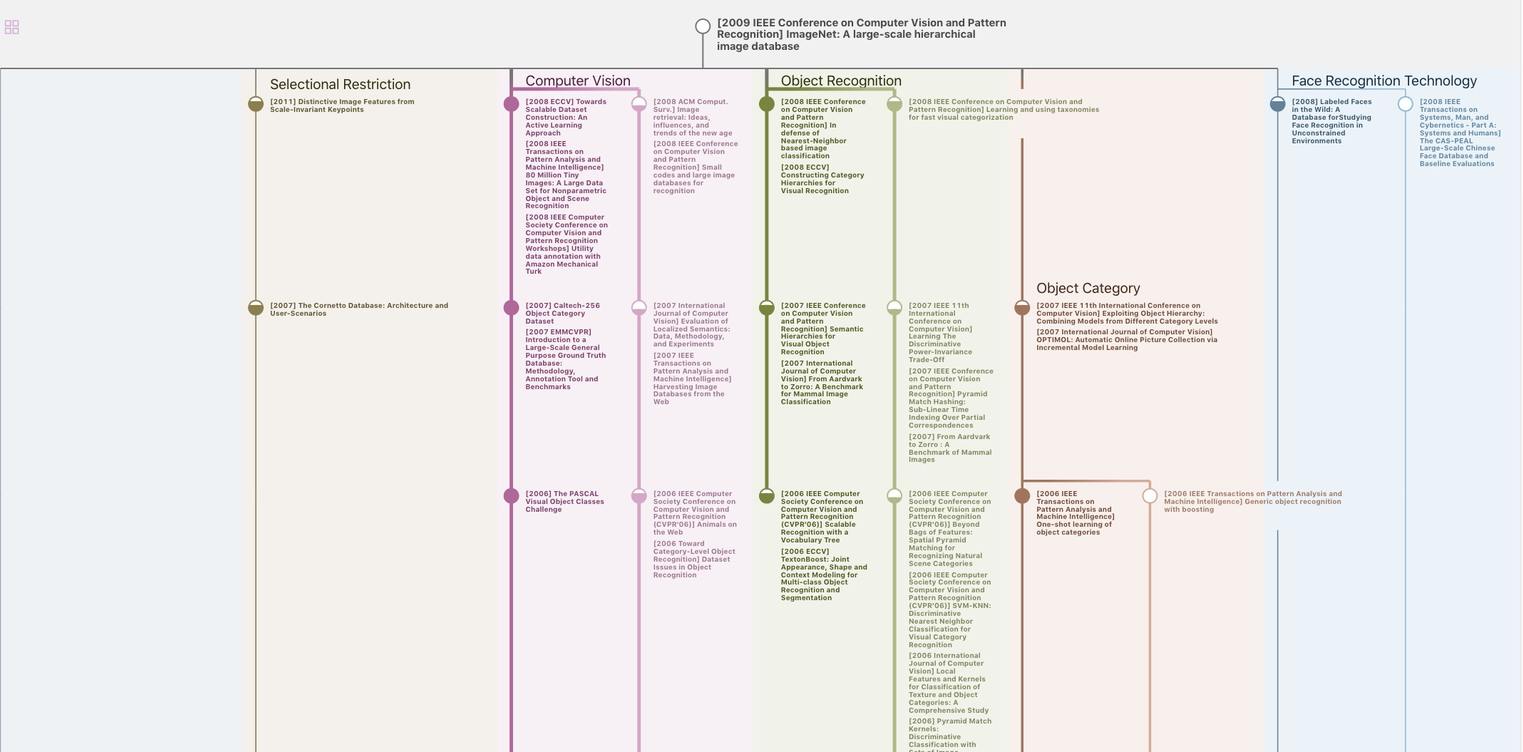
生成溯源树,研究论文发展脉络
Chat Paper
正在生成论文摘要