A Performance Efficient Approach of Global Training in Federated Learning.
ICAIIC(2023)
摘要
Federated learning is a novel approach of training the global model on the server by utilizing the personal data of the end users while data privacy is preserved. The users called clients are required to perform the local training using their local datasets and forward those trained local models to the server, in which the local models are aggregated to update the global model. This process of global training is carried out for several rounds until the convergence. Practically, the clients' data is non-independent and identically distributed (Non-IID). Hence, the updated local model of each client may vary from every other client due to heterogeneity among them. Hence, the process of aggregating the diversified local models of clients has a huge impact on the performance of global training. This article proposes a performance efficient aggregation approach for federated learning, which considers the data heterogeneity among clients before aggregating the received local models. The proposed approach is compared with the conventional federated learning methods, and it achieves improved performance.
更多查看译文
关键词
Federated learning,heterogeneous networks,deep learning
AI 理解论文
溯源树
样例
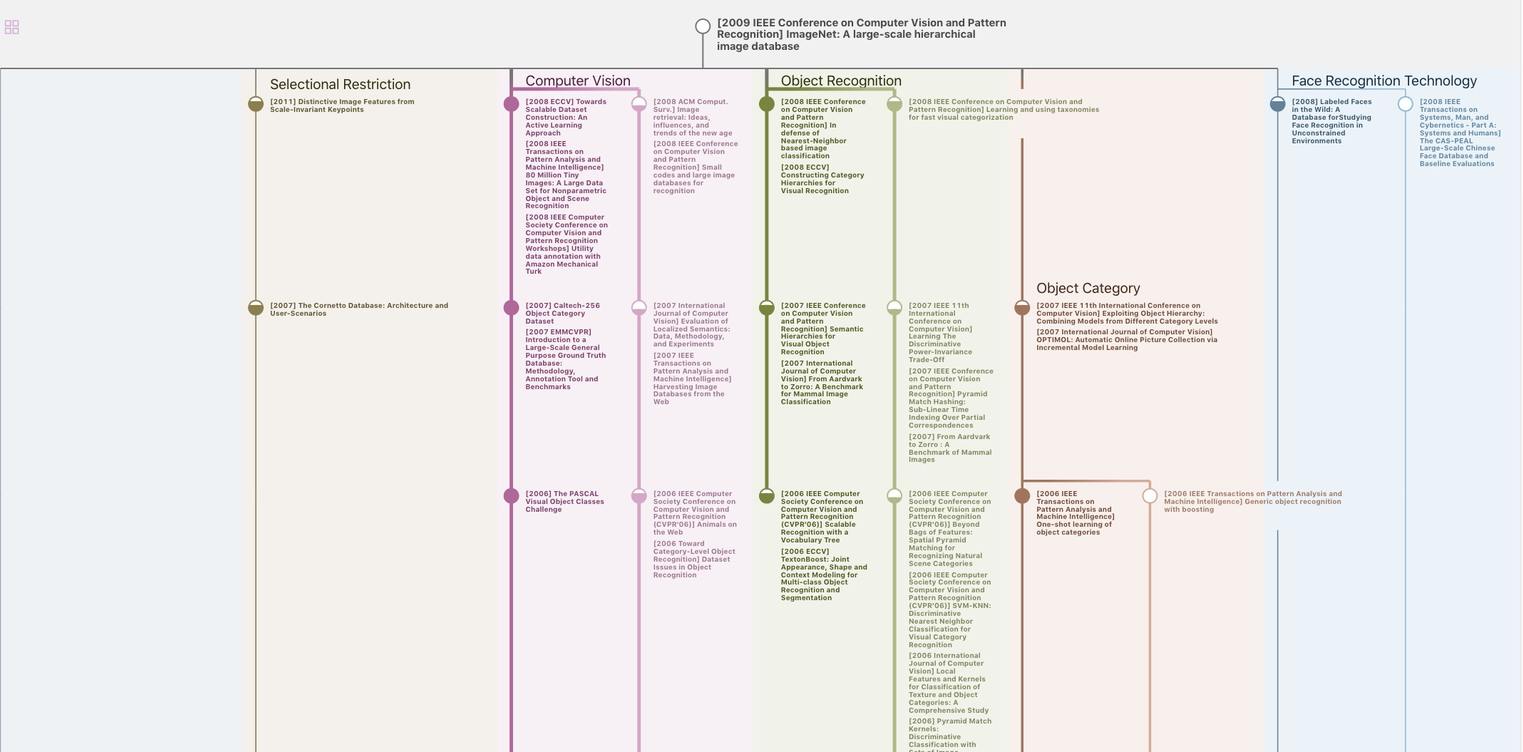
生成溯源树,研究论文发展脉络
Chat Paper
正在生成论文摘要