Identifying Important Features for Clinical Diagnosis of Thyroid Disorder.
ASONAM(2022)
摘要
Abnormal production of thyroid hormones in our body causes thyroid disorders such as hypothyroidism, hyper-thyroidism, Hashimoto's disease, Graves' disease, and thyroid nodules. Undiagnosed thyroid disorders can affect the quality of life of an individual both physically and mentally. Thyroid disorders are common but sometimes become difficult to diagnose since the symptoms can be easily associated with other health conditions. Clinicians identify thyroid disorders by measuring the levels of thyroid hormones in our blood stream. This work aims to help clinicians by carefully investigating if thyroid diagnosis improves when all important features (a complete thyroid panel) is measured as opposed to a select few. Much of previous work has focused on the performance of classifiers, supervised and unsupervised, for the prediction of this disorder. Departing from this tradition, we focus on the concept of feature importance and its clinical implications. We identify the top-4 important features that predict the presence of thyroid disorder and show that these can be measured by clinicians cost-effectively. We also identify the pitfalls of current clinical practice of not checking the entire thyroid panel, prevalent in many countries with universal health care. Finally, we show that our results are quite robust and are unlikely to change with the choice of classifier or due to the inherent nature of a dataset in hand like imbalance.
更多查看译文
关键词
thyroid disease,data mining,machine learning,feature importance
AI 理解论文
溯源树
样例
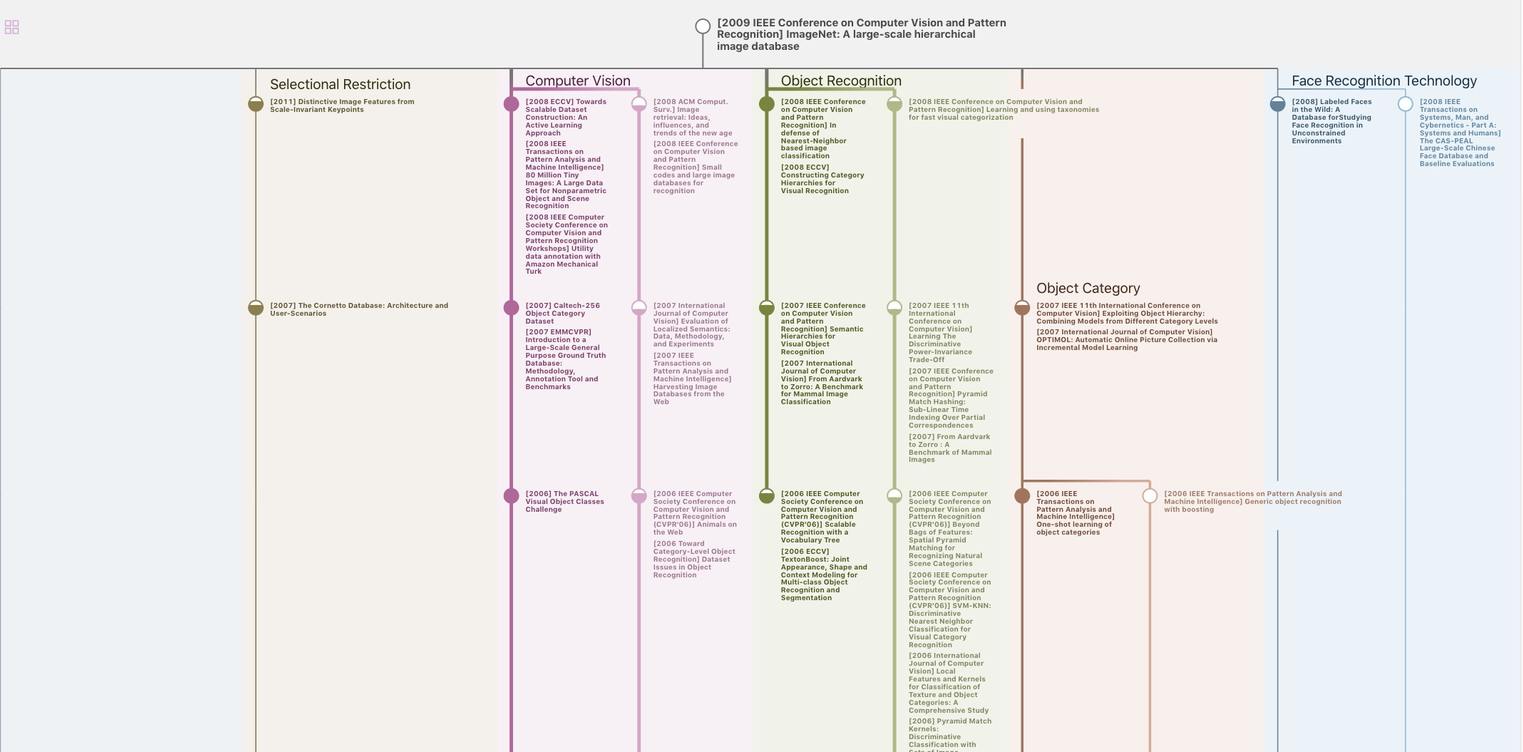
生成溯源树,研究论文发展脉络
Chat Paper
正在生成论文摘要