Interpretable Classification of Pneumonia Infection Using eXplainable AI (XAI-ICP).
IEEE Access(2023)
摘要
Open-box models in the medical domain have high acceptance and demand by many medical examiners. Even though the accuracy predicted by most of convolutional neural network (CNN) models is high, it is still not convincing as the detailed discussion regarding the outcome is semi-transparent in the functioning process. Pneumonia is known as one of the top contagious infections that makes most of the population affected due to low immunity. Therefore, the goal of this paper is to implement an interpretable classification of pneumonia infection using eXplainable AI (XAI-ICP). Thus, XAI-ICP is the highly efficient system designed to solve this challenge by adapting to the recent population health conditions. The aim is to design an interpretable deep classification and transfer learning based evaluation for pneumonia infection classification. The model is primarily pre-trained using the open Chest X-Ray (CXR) dataset from National Institutes of Health (NIH). Whereas, the training input and testing given to this system is Taichung Veterans General Hospital (TCVGH) for independent learning, Taiwan + VinDr open dataset for transfer learning of pneumonia affected patients with labeled CXR images possessing three features of infiltrate, cardiomegaly and effusion. The data labeling is performed by the medical examiners with the XAI human-in-the-loop approach. XAI-ICP demonstrates the XAI based reconfigurable DCNN with human-in-the-loop as a novel approach. The interpretable deep classification provides detailed transparency analysis and transfer learning for competitive accuracy. The purpose of this work, to design a re-configurable model that can continuously improve itself by using a feedback system and provide feasibility for the model deployment across multiple countries to provide an efficient system for the pneumonia infection classification. The designed model then provides detailed decisions taken at each step as transparency and features used within the algorithm for the pneumonia classification during the hospitalization. Thus, the scope can be given as explainable AI usage for the diagnosis classification using data preprocessing and interpretable deep convolutional neural network by the CXR evaluation. The accuracy achieved by using independent learning classification is 92.14% and is further improved based on successive transfer learning based evaluation is 93.29%. The XAI-ICP model adapts to the different populations by using transfer learning, while providing competitive results to the affected conditions.
更多查看译文
关键词
Pulmonary diseases, Transfer learning, Artificial intelligence, Solid modeling, Medical diagnostic imaging, Convolutional neural networks, Data models, Medical XAI, XAI pnuemonia, transfer learning, pneumonia infection
AI 理解论文
溯源树
样例
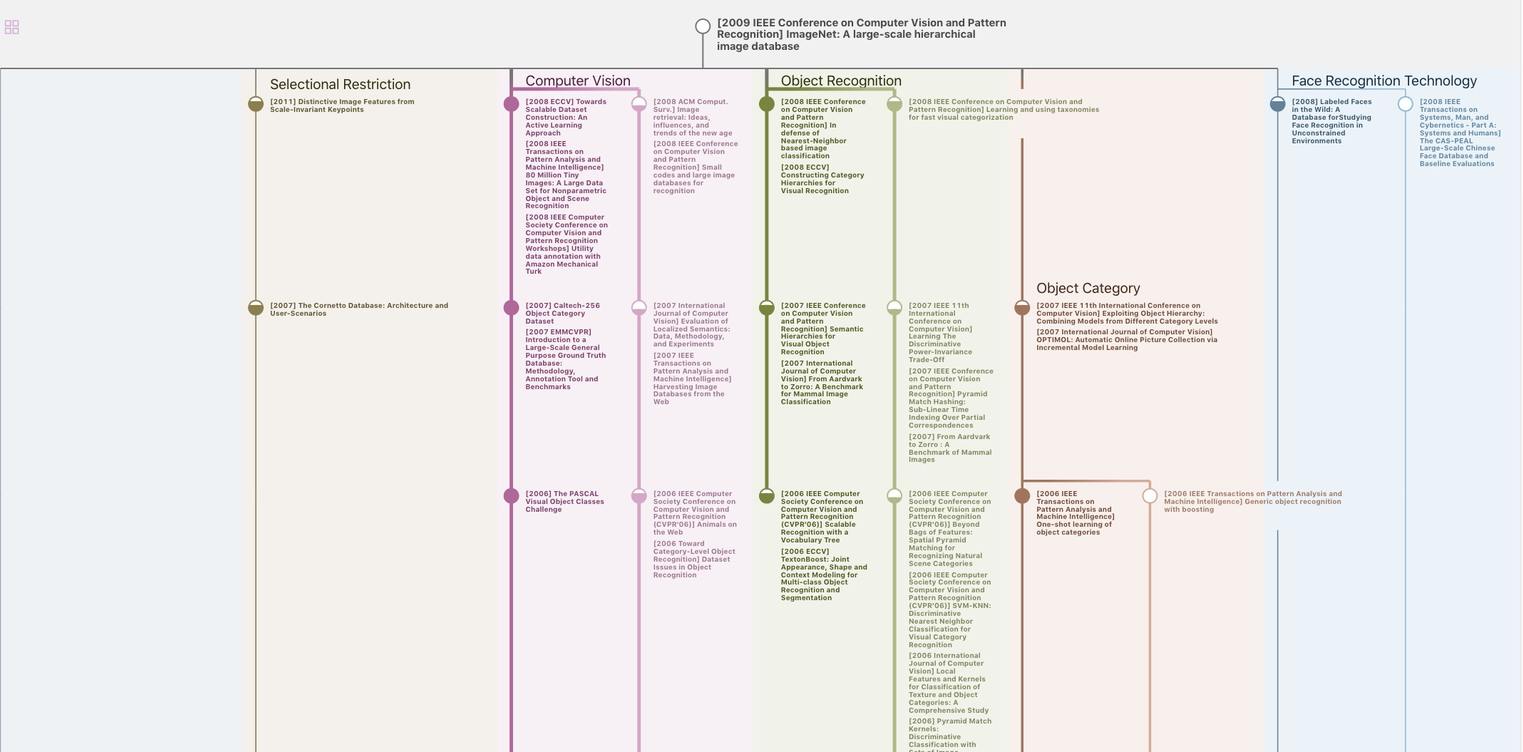
生成溯源树,研究论文发展脉络
Chat Paper
正在生成论文摘要