Reinforcement Learning Enhanced PicHunter for Interactive Search.
MMM (1)(2023)
摘要
With the tremendous increase in video data size, search performance could be impacted significantly. Specifically, in an interactive system, a real-time system allows a user to browse, search and refine a query. Without a speedy system quickly, the main ingredient to engage a user to stay focused, an interactive system becomes less effective even with a sophisticated deep learning system. This paper addresses this challenge by leveraging approximate search, Bayesian inference, and reinforcement learning. For approximate search, we apply a hierarchical navigable small world, which is an efficient approximate nearest neighbor search algorithm. To quickly prune the search scope, we integrate PicHunter, one of the most popular engines in Video Browser Showdown, with reinforcement learning. The integration enhances PicHunter with the ability of systematic planning. Specifically, PicHunter performs a Bayesian update with a greedy strategy to select a small number of candidates for display. With reinforcement learning, the greedy strategy is replaced with a policy network that learns to select candidates that will result in the minimum number of user iterations, which is analytically defined by a reward function. With these improvements, the interactive system only searches a subset of video datasets relevant to a query while being able to quickly perform Bayesian updates with systematic planning to recommend the most probable candidates that can potentially lead to minimum iteration rounds.
更多查看译文
关键词
Reinforcement learning, Bayesian method, Relevance feedback, Interactive video retrieval
AI 理解论文
溯源树
样例
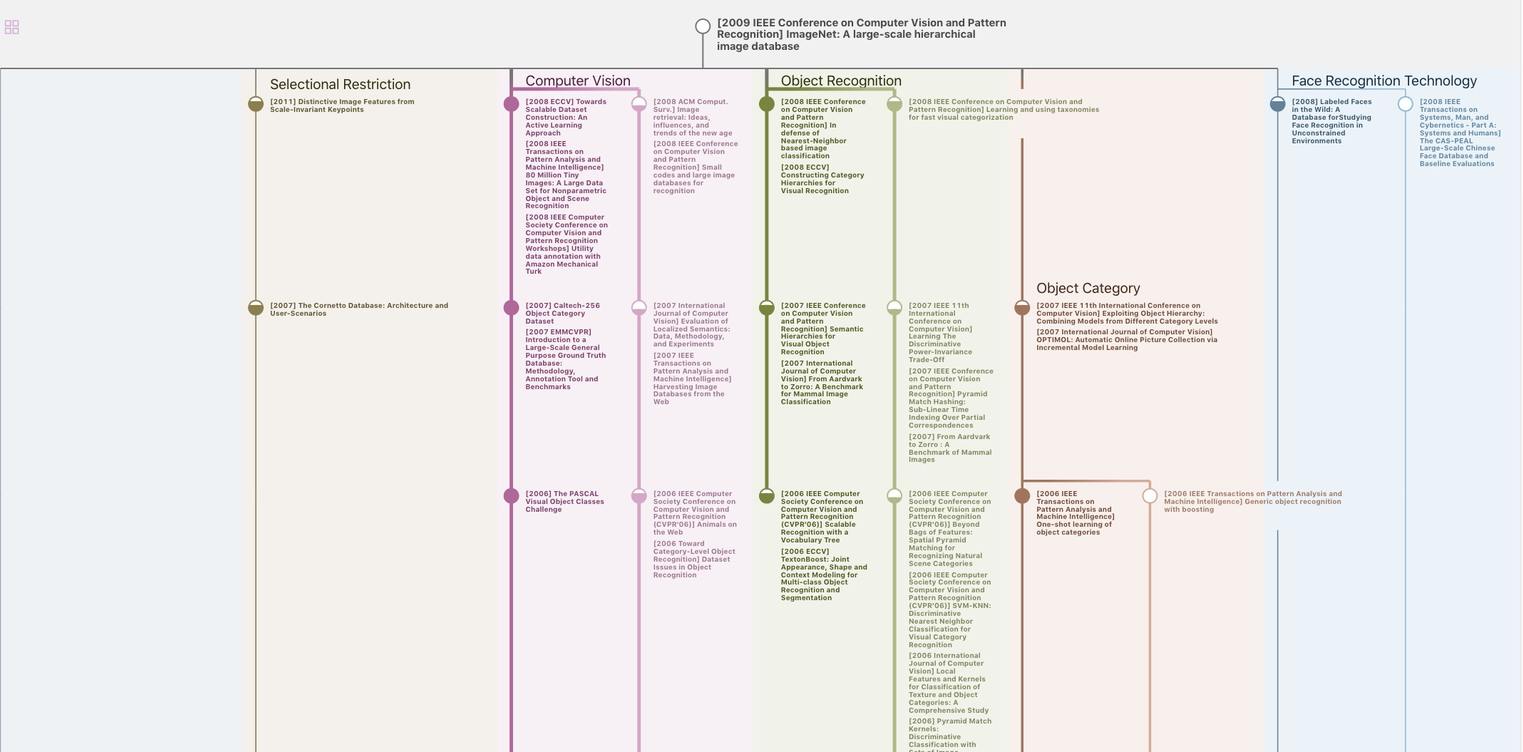
生成溯源树,研究论文发展脉络
Chat Paper
正在生成论文摘要