A General M-estimation Theory in Semi-Supervised Framework
JOURNAL OF THE AMERICAN STATISTICAL ASSOCIATION(2023)
摘要
We study a class of general M-estimators in the semi-supervised setting, wherein the data are typically a combination of a relatively small labeled dataset and large amounts of unlabeled data. A new estimator, which efficiently uses the useful information contained in the unlabeled data, is proposed via a projection technique. We prove consistency and asymptotic normality, and provide an inference procedure based on K -fold cross-validation. The optimal weights are derived to balance the contributions of the labeled and unlabeled data. It is shown that the proposed method, by taking advantage of the unlabeled data, produces asymptotically more efficient estimation of the target parameters than the supervised counterpart. Supportive numerical evidence is shown in simulation studies. Applications are illustrated in analysis of the homeless data in Los Angeles. for this article are available online.
更多查看译文
关键词
Projection method,Semi-supervised inference,Weighted loss function
AI 理解论文
溯源树
样例
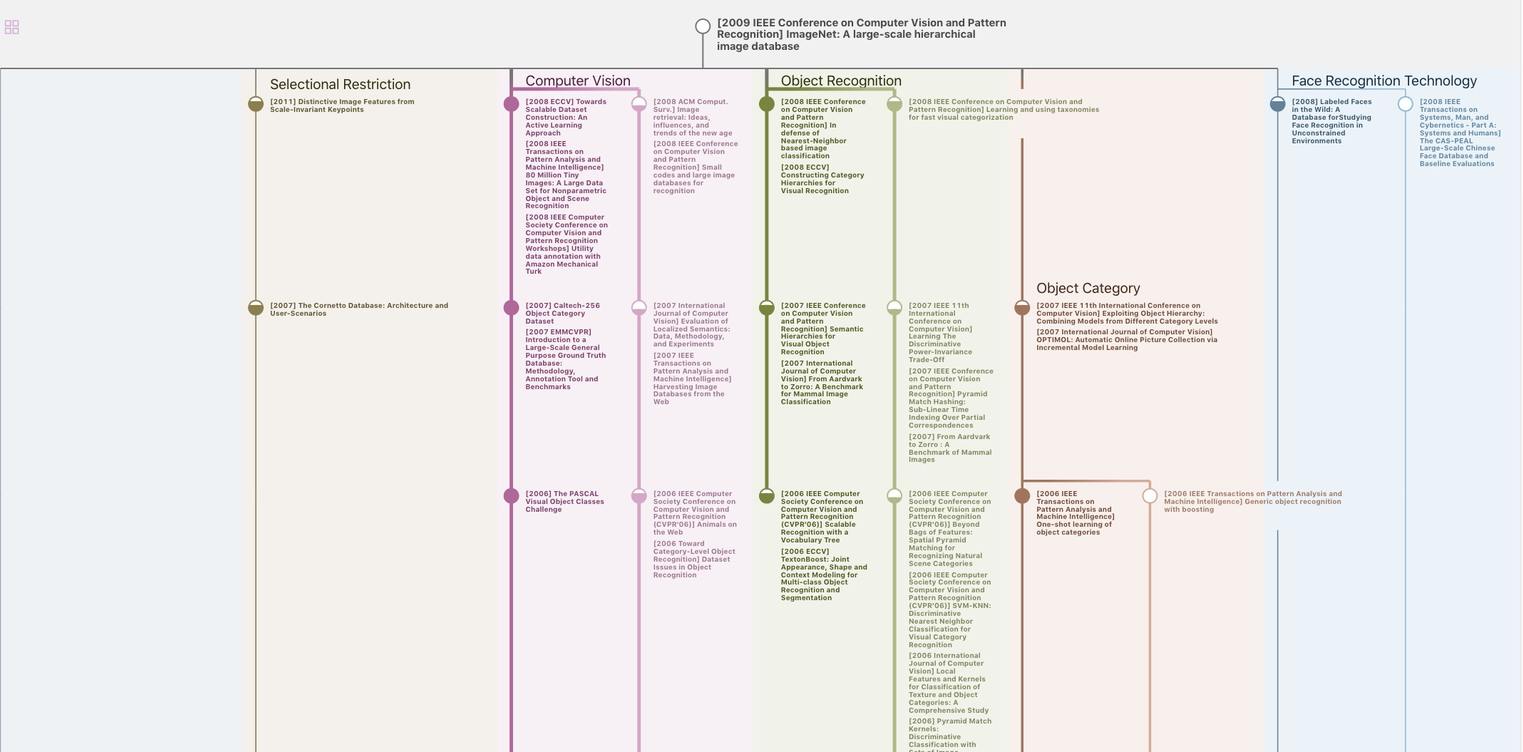
生成溯源树,研究论文发展脉络
Chat Paper
正在生成论文摘要