Bayesian clustering of spatial functional data with application to a human mobility study during covid-19
ANNALS OF APPLIED STATISTICS(2023)
摘要
The coronavirus (COVID-19) global pandemic has made a significant impact on people's social activities. Cell phone mobility data provide unique and rich information on studying this impact. The motivating dataset of this study is the daily leaving-home index data at Harris County in Texas provided by SafeGraph. To study changes in daily leaving-home index and how they relate to public policy and sociodemographic variables, we propose a new Bayesian wavelet model for modeling and clustering spatial functional data, where domain partitioning is achieved by operating on the spanning trees. The resulting clusters can have arbitrary shapes and are spatially contiguous in the input domain. An efficient tailored reversible jump Markov chain Monte Carlo algorithm is proposed to implement the model. The method is applied to the spatial functional data of the daily percentages of people who left home. We focus on the time period covering both lockdown and phased reopening in Texas during the COVID-19 pandemic and study the changing behaviors of those functional curves. By linking the clustering results with the sociodemographic information, we identify several covariates of census blocks that have a noticeable impact on the clustering patterns of people's mobility behaviors.
更多查看译文
关键词
Key words and phrases,Bayesian wavelet model,mobility behavior,reversible jump Markov chain Monte Carlo,spanning tree,spatial functional data
AI 理解论文
溯源树
样例
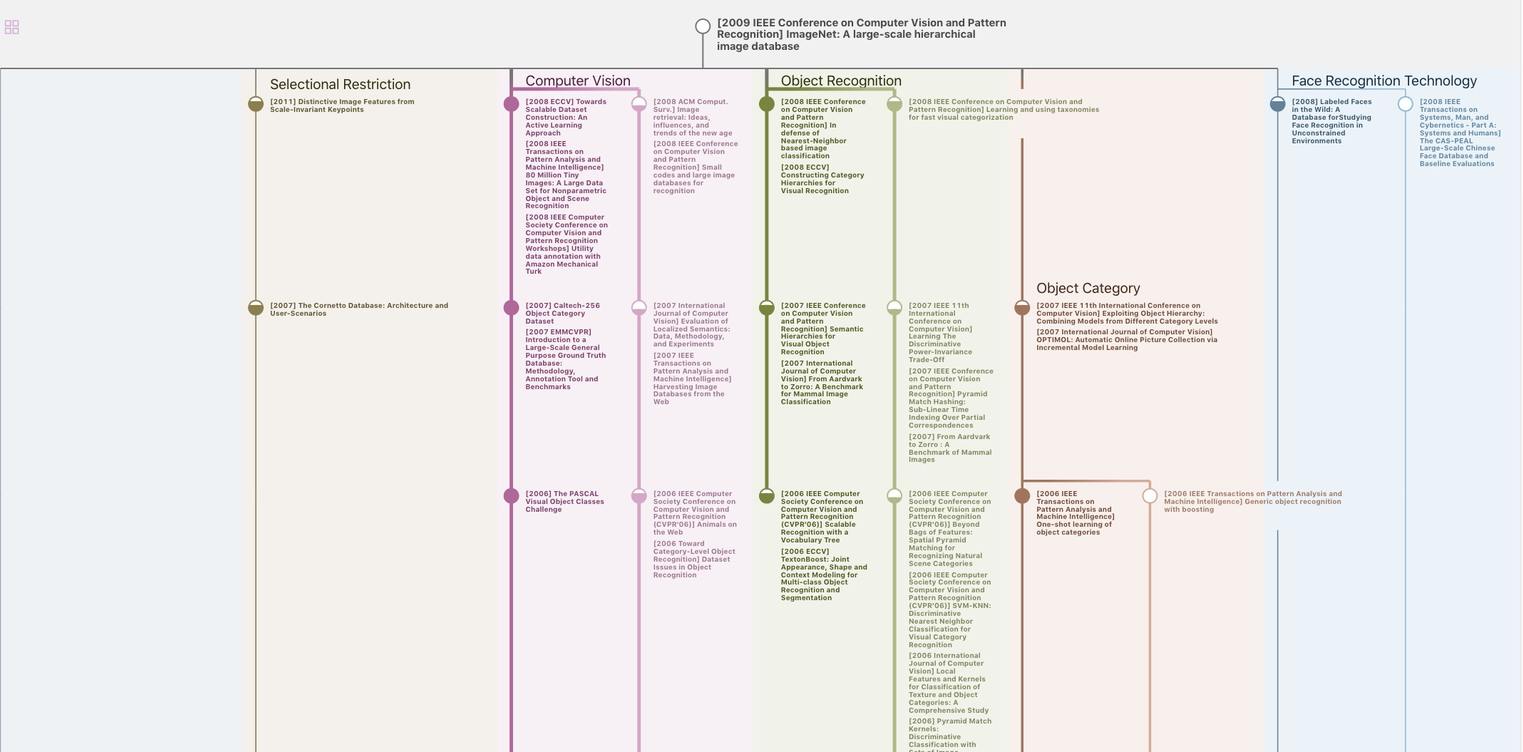
生成溯源树,研究论文发展脉络
Chat Paper
正在生成论文摘要