An Adaptive Multisensor Fault Diagnosis Method for High-Speed Train Bogie
IEEE TRANSACTIONS ON INTELLIGENT TRANSPORTATION SYSTEMS(2023)
摘要
High-speed train bogies are the critical components of high-speed trains, which can play the role of traction, braking, and buffering. In long-term train service, bogies are prone to wear and aging. Currently, most studies on fault diagnosis methods are aimed at single equipment identification. It is challenging to accurately diagnose the faults of such coupled multi-equipment combination systems as bogies. Multiple devices on the bogie have implied correlations in space, and fully exploiting their spatial features enhances the fault diagnosis accuracy. This paper proposes a new bogie fault diagnosis method based on the directional graph of train bogie: RS-GAT model. The model uses the Residual-Squeeze Network (RS-Net) to construct the framework of the model and use the Graph Attention Network (GAT) for spatial information fusion and feature extraction to identify bogie faults. Using six datasets collected under the operation of high-speed trains, experimental results demonstrate that the proposed approach has better effectiveness than the RS-Net class model and single-layer graph class model, with diagnosis accuracy near 96%. Ablation experiments and comparisons between RS-GAT and RS-GCN verify the effectiveness of RS-Net framework and GAT model in fault classification. In addition, the RS-GAT model is found to have strong robustness when different models are analyzed using small-scale data sets.
更多查看译文
关键词
Fault diagnosis,Feature extraction,Axles,Temperature sensors,Convolutional neural networks,Temperature measurement,Safety,graph attention network (GAT),residual-squeeze net (RS-Net),high-speed train bogie
AI 理解论文
溯源树
样例
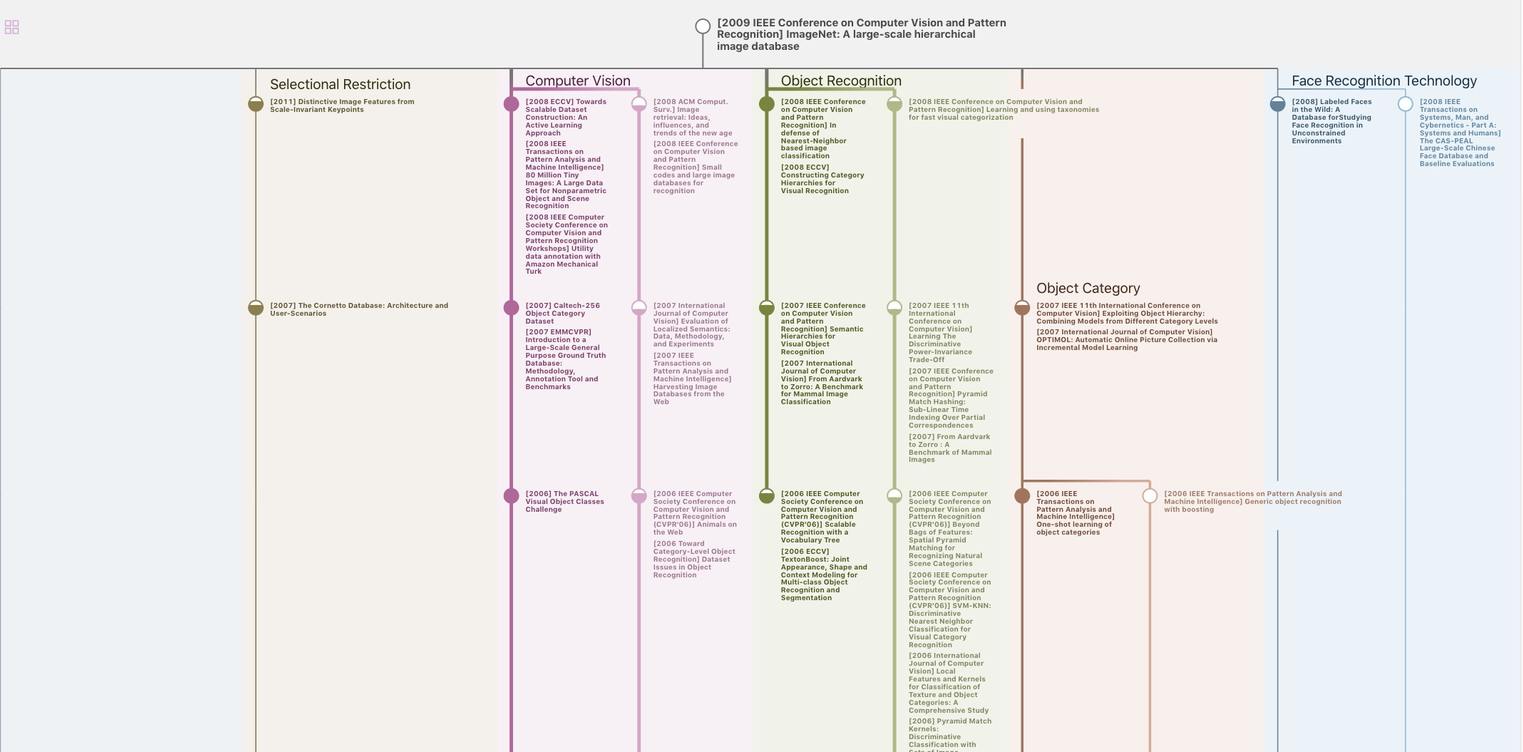
生成溯源树,研究论文发展脉络
Chat Paper
正在生成论文摘要